Handcrafted and Neural Network Based Features for Outlier Modulation Detection.
IEEECONF(2022)
摘要
Outlier detection is an important topic in radio frequency signal detection and classification. In this paper, two methods for outlier signal detection in the presence of strongly and moderately confusing modulations are proposed. The first method feeds selected handcrafted features to the distance discrimination classifiers for outlier detection. By removing the redundant features, this method can reach above 95% sensitivity and specificity at signal-to-noise ratio (SNR) equals 0dB and nearly 100% at high SNRs for mild confusers. In the second method, handcrafted features and neural network based features are used as inputs to several state-of-the-art machine learning classifiers such as one-class support vector machine, isolation forest, and one-class neural networks. From the results, the first method can achieve 83.90% accuracy; isolation forest and one-class neural networks with handcrafted features can reach 79.58% and 76.76% accuracy over all outlier signals at SNR=5dB which are noticeably higher than existing works in this field.
更多查看译文
关键词
Outlier detection,feature engineering,deep neural network,modulation classification
AI 理解论文
溯源树
样例
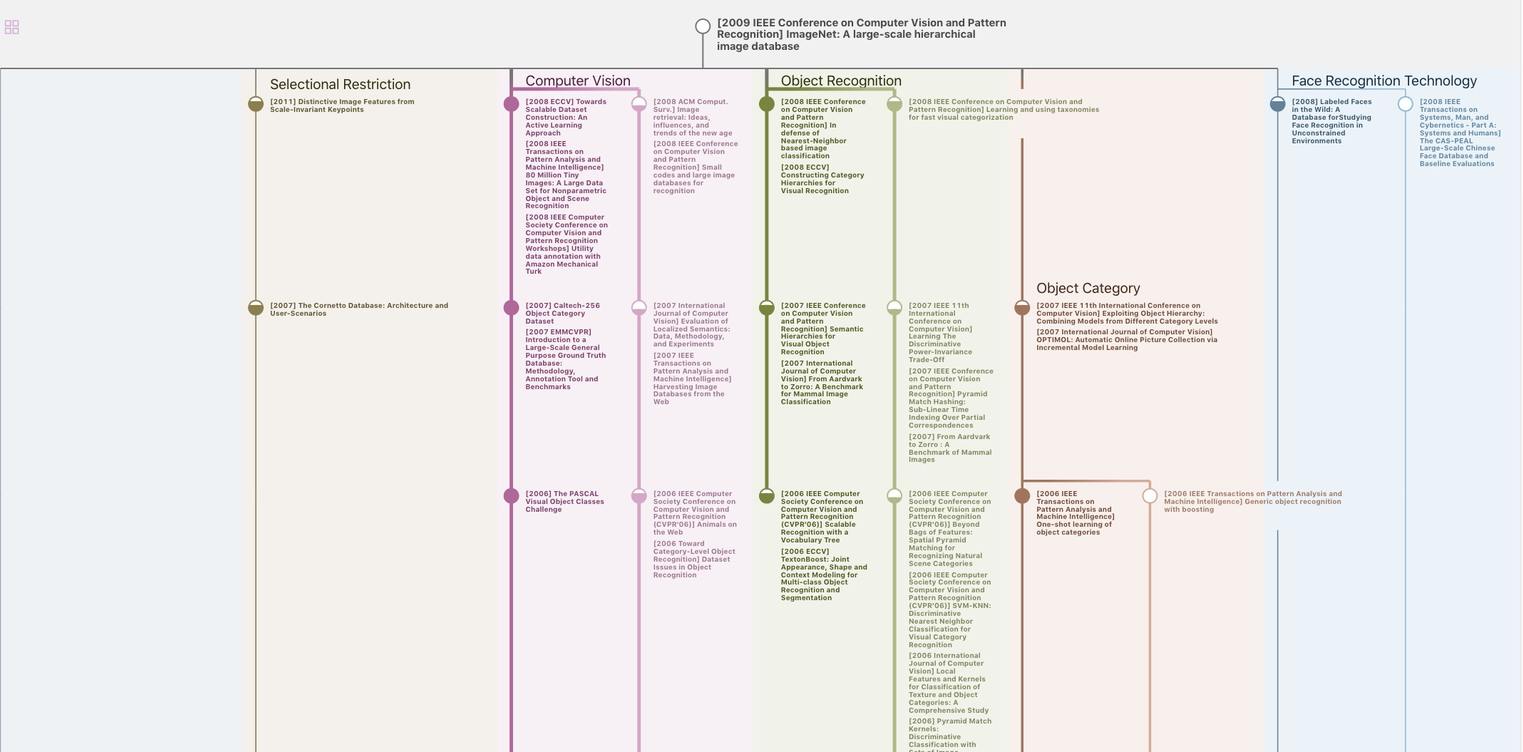
生成溯源树,研究论文发展脉络
Chat Paper
正在生成论文摘要