Switching Functional Network Models of Oscillatory Brain Dynamics.
IEEECONF(2022)
Abstract
Oscillations in brain activity are critical for information processing, and changes in brain-wide oscillatory coupling networks can be used to track changes in brain state in health and disease. Classical methods for estimating these rhythmic functional networks such as pairwise coherence analysis and more recent methods such as global coherence analysis are limited in their statistical efficiency and temporal resolution. In this work, we introduce a state-space modeling framework, which allows us to estimate the connectivity structure of several distinct functional network modes and estimate which network mode is being expressed at each moment. We use the Kalman filter and the Rauch-Tung-Striebel (RTS) smoother to estimate the states of the underlying oscillations and use the expectation-maximization (EM) algorithm to estimate the model parameters. We demonstrate the application of this model framework in an analysis of EEG data from a subject undergoing general anesthesia, and compare the estimated network structures to the modes estimated by global coherence analysis. Our method captures similar network modes, and additionally tracks instantaneous changes in network state that can depend on multiple simultaneous oscillations.
MoreTranslated text
Key words
State-space models, functional networks, functional connectivity
AI Read Science
Must-Reading Tree
Example
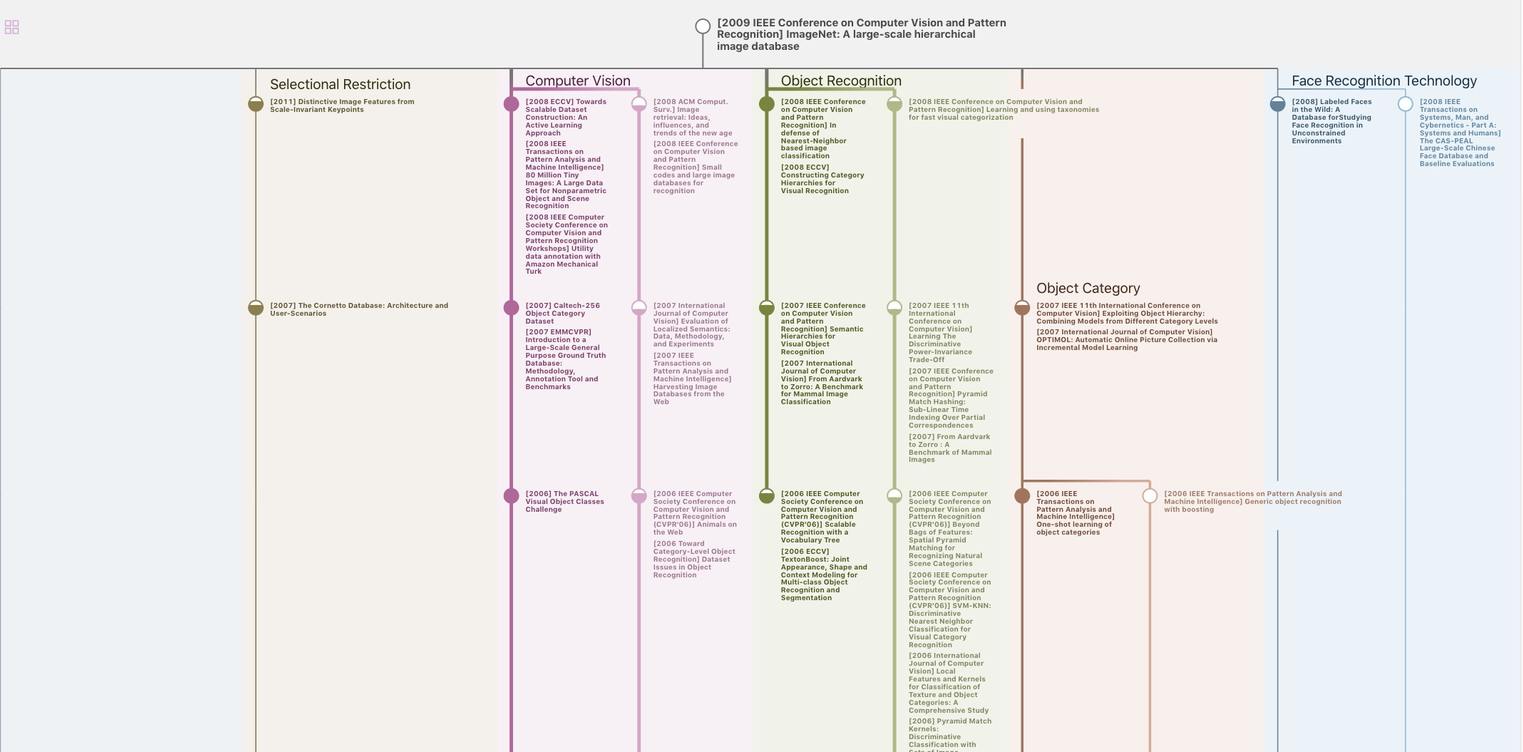
Generate MRT to find the research sequence of this paper
Chat Paper
Summary is being generated by the instructions you defined