Exploring the Geometry of Generative Priors with Applications in Cellular MRI.
IEEECONF(2022)
Abstract
Judicious utilization of appropriate priors is essential for solving ill-posed inverse problems, especially with limited measurements. Recently, generative priors have shown impressive performance in solving inverse problems for image reconstruction, including biomedical imaging. On the one hand, the architecture of generative models plays a key role in shaping the priors, which can capture hidden structures in the signal of interest. For example, ReLU generative networks induce special partitions in the latent space and affinely map them to affine sets in the image space. On the other hand, the training data also manipulates the geometry of the latent space by adjusting the parameters of trained generative priors. In this work, we study the interplay between the architecture of generative priors and training data, and how it can be utilized for designing better reconstruction algorithms. We will focus on a specific class of inverse problems that arise in cellular and molecular magnetic resonance imaging (MRI), where labeled macrophages and cells are tracked in vivo to understand immunotherapy responses. We will utilize our understanding of this interplay to develop algorithms that can potentially enhance the performance of cellular MRI. (1)
MoreTranslated text
Key words
appropriate priors,biomedical imaging,cellular MRI,cellular resonance imaging,generative models,image reconstruction,image space,inverse problems,latent space,molecular magnetic resonance imaging,ReLU generative networks,trained generative priors,training data
AI Read Science
Must-Reading Tree
Example
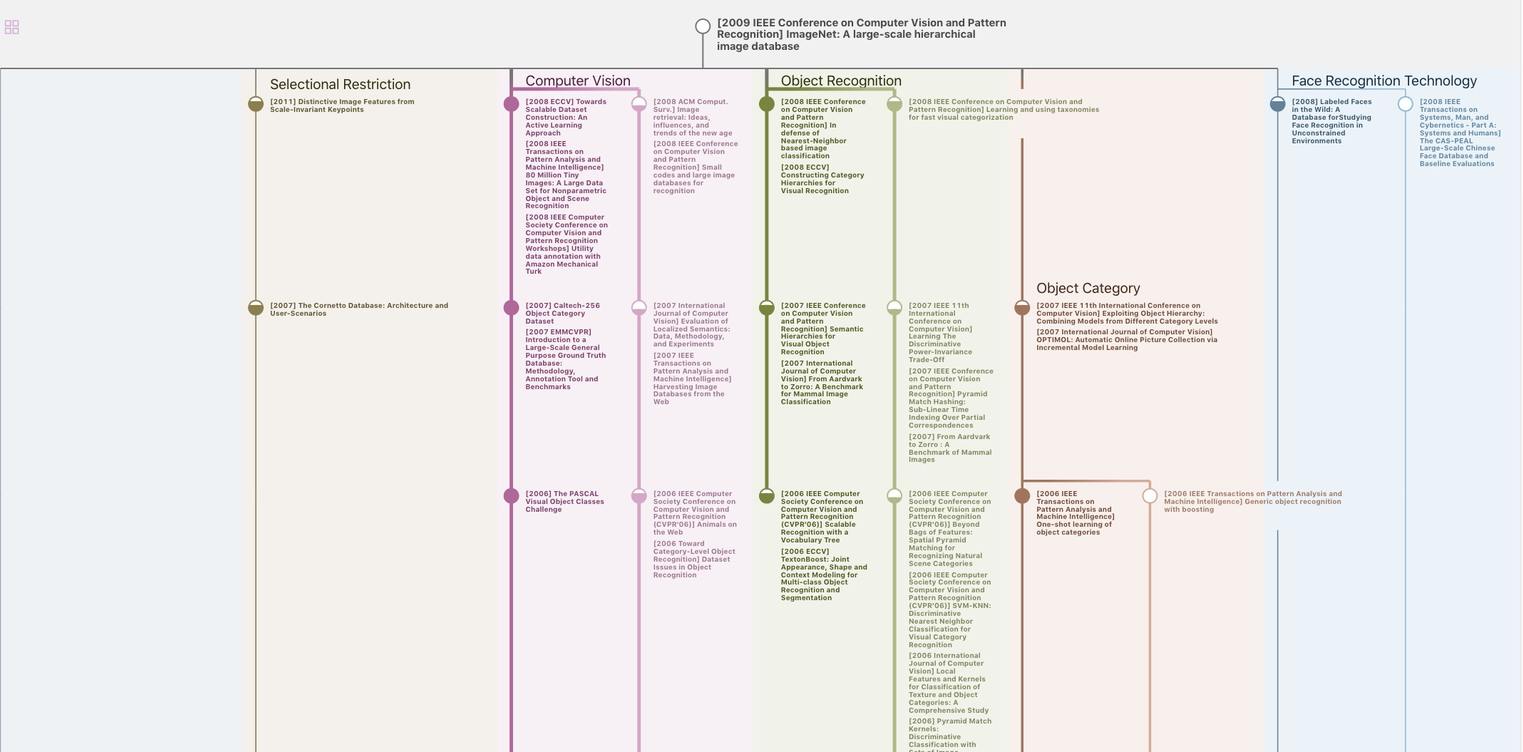
Generate MRT to find the research sequence of this paper
Chat Paper
Summary is being generated by the instructions you defined