Learning Query-Space Document Representations for High-Recall Retrieval.
ECIR (2)(2023)
摘要
Recent studies have shown that significant performance improvements reported by neural rankers do not necessarily extend to a diverse range of queries. There is a large set of queries that cannot be effectively addressed by neural rankers primarily because relevant documents to these queries are not identified by first-stage retrievers. In this paper, we propose a novel document representation approach that represents documents within the query space, and hence increases the likelihood of recalling a higher number of relevant documents. Based on experiments on the MS MARCO dataset as well as the hardest subset of its queries, we find that the proposed approach shows synergistic behavior to existing neural rankers and is able to increase recall both on MS MARCO dev set queries as well as the hardest queries of MS MARCO.
更多查看译文
AI 理解论文
溯源树
样例
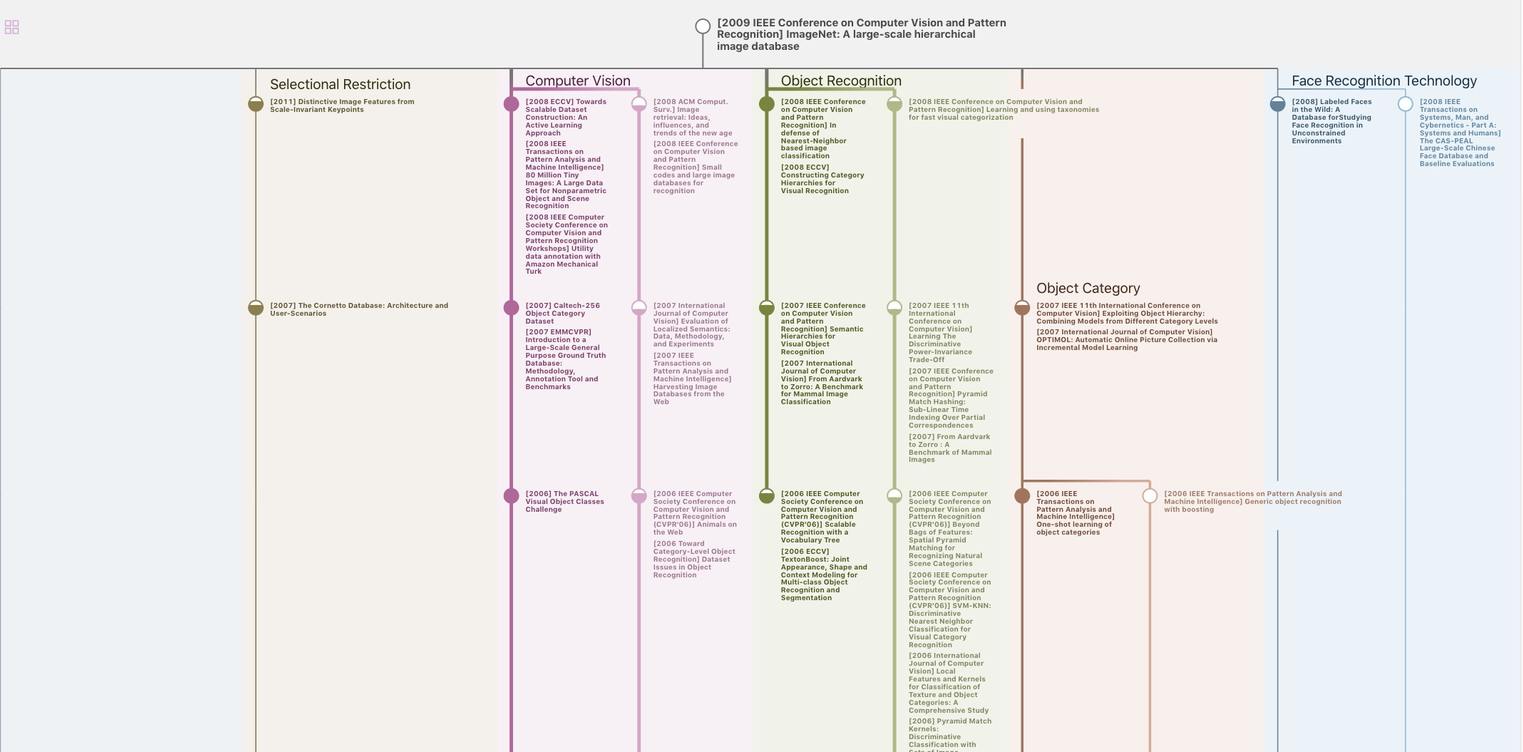
生成溯源树,研究论文发展脉络
Chat Paper
正在生成论文摘要