Topic-Enhanced Personalized Retrieval-Based Chatbot.
ECIR (2)(2023)
摘要
Building a personalized chatbot has drawn much attention recently. A personalized chatbot is considered to have a consistent personality. There are two types of methods to learn the personality. The first mainly model the personality from explicit user profiles (e.g., manually created persona descriptions). The second learn implicit user profiles from the user's dialogue history, which contains rich, personalized information. However, a user's dialogue history can be long and noisy as it contains long-time, multi-topic historical dialogue records. Such data noise and redundancy impede the model's ability to thoroughly and faithfully learn a consistent personality, especially when applied with models that have an input length limit (e.g., BERT). In this paper, we propose deconstructing the long and noisy dialogue history into topic-dependent segments. We only use the topically related dialogue segment as context to learn the topic-aware user personality. Specifically, we design a Topic-enhanced personalized Retrieval-based Chatbot, TopReC. It first deconstructs the dialogue history into topic-dependent dialogue segments and filters out irrelevant segments to the current query via a Heter-Merge-Reduce framework. It then measures the matching degree between the response candidates and the current query conditioned on each topic-dependent segment. We consider the matching degree between the response candidate and the cross-topic user personality. The final matching score is obtained by combining the topic-dependent and cross-topic matching scores. Experimental results on two large dataset show that TopReC outperforms all previous state-of-the-art methods.
更多查看译文
关键词
Personalization, Dialogue systems
AI 理解论文
溯源树
样例
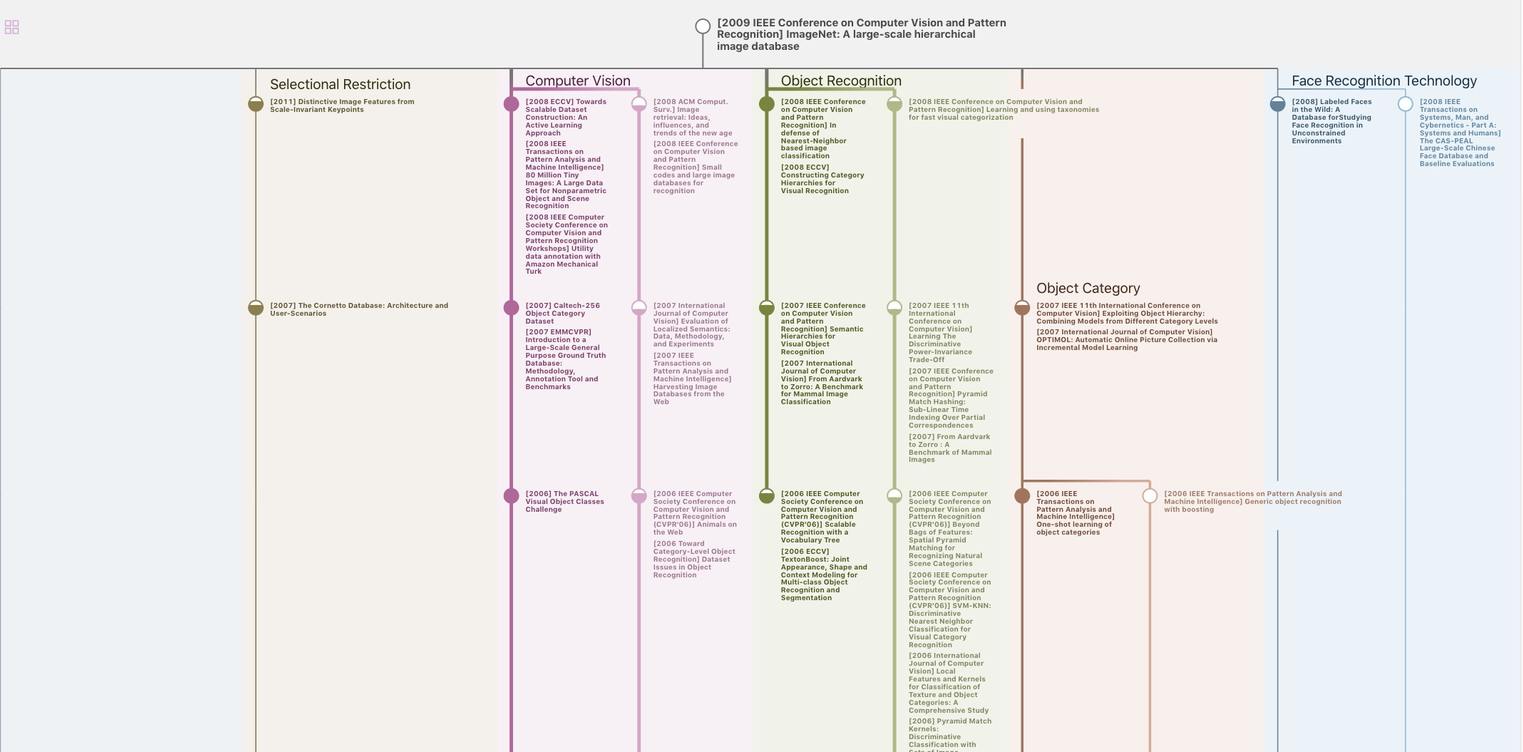
生成溯源树,研究论文发展脉络
Chat Paper
正在生成论文摘要