Recommendation Algorithm Based on Deep Light Graph Convolution Network in Knowledge Graph.
ECIR (1)(2023)
摘要
Recently, recommendation algorithms based on Graph Convolution Network (GCN) have achieved many surprising results thanks to the ability of GCN to learn more efficient node embeddings. However, although GCN shows powerful feature extraction capability in user-item bipartite graphs, the GCN-based methods appear powerless for knowledge graph (KG) with complex structures and rich information. In addition, all of the existing GCN-based recommendation systems suffer from the over-smoothing problem, which results in the models not being able to utilize higher-order neighborhood information, and thus these models always achieve their best performance at shallower layers. In this paper, we propose a Deep Light Graph Convolution Network for Knowledge Graph (KDL-GCN) to alleviate the above limitations. Firstly, the User-Entity Bipartite Graph approach (UE-BP) is proposed to simplify knowledge graph, which leverages entity information by constructing multiple interaction graphs. Secondly, a Deep Light Graph Convolution Network (DLGCN) is designed to make full use of higher-order neighborhood information. Finally, experiments on three real-world datasets show that the KDL-GCN proposed in this paper achieves substantial improvement compared to the state-of-the-art methods.
更多查看译文
关键词
Recommendation, Graph convolution network, Knowledge graph
AI 理解论文
溯源树
样例
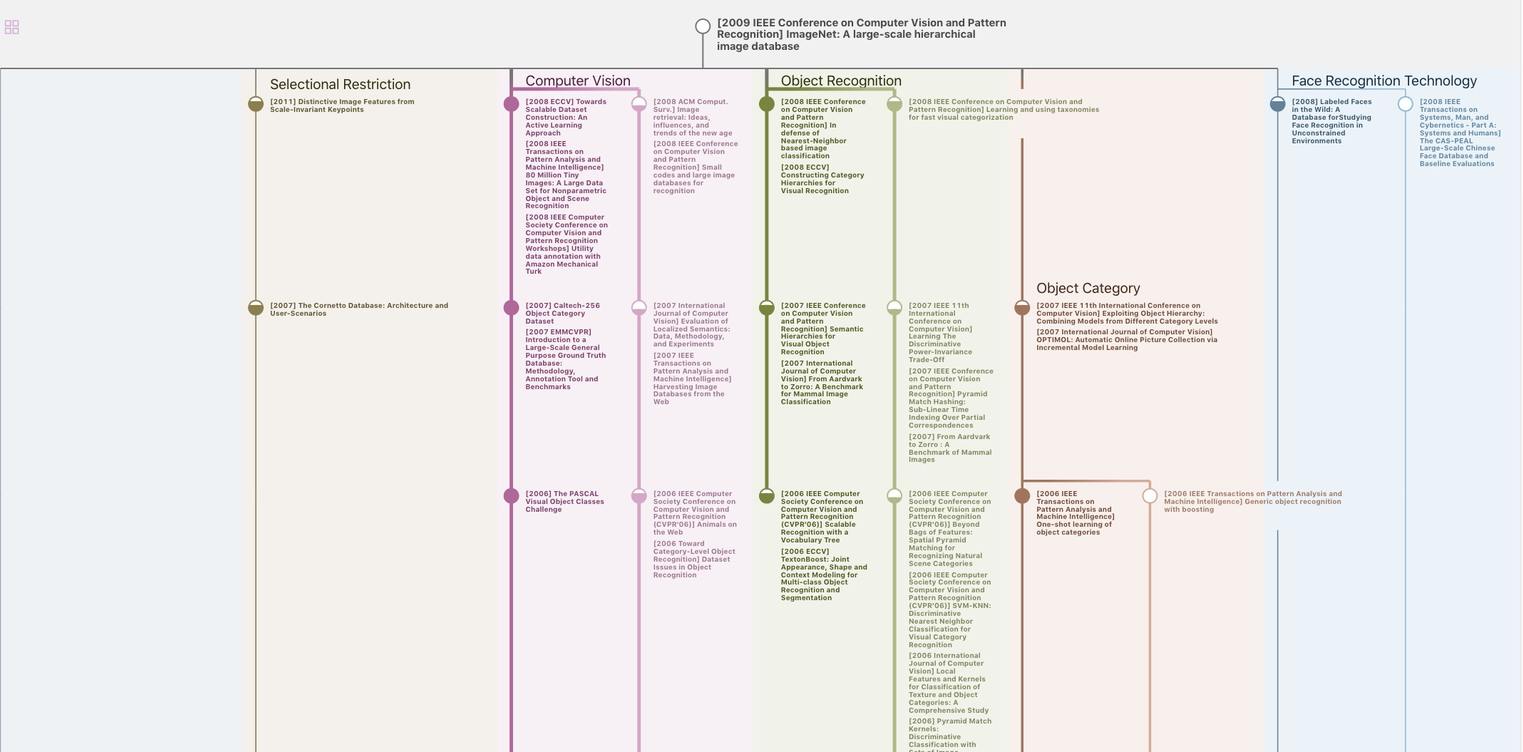
生成溯源树,研究论文发展脉络
Chat Paper
正在生成论文摘要