CoLISA: Inner Interaction via Contrastive Learning for Multi-choice Reading Comprehension.
ECIR (1)(2023)
摘要
Multi-choice reading comprehension (MC-RC) is supposed to select the most appropriate answer from multiple candidate options by reading and comprehending a given passage and a question. Recent studies dedicate to catching the relationships within the triplet of passage, question, and option. Nevertheless, one limitation in current approaches relates to the fact that confusing distractors are often mistakenly judged as correct, due to the fact that models do not emphasize the differences between the answer alternatives. Motivated by the way humans deal with multi-choice questions by comparing given options, we propose CoLISA (Contrastive Learning and In-Sample Attention), a novel model to prudently exclude the confusing distractors. In particular, CoLISA acquires option-aware representations via contrastive learning on multiple options. Besides, in-sample attention mechanisms are applied across multiple options so that they can interact with each other. The experimental results on QuALITY and RACE demonstrate that our proposed CoLISA pays more attention to the relation between correct and distractive options, and recognizes the discrepancy between them. Meanwhile, CoLISA also reaches the state-of-the-art performance on QuALITY (Our code is available at https://github.com/Walle1493/CoLISA..).
更多查看译文
关键词
Machine reading comprehension, Multi-choice question answering, Contrastive learning
AI 理解论文
溯源树
样例
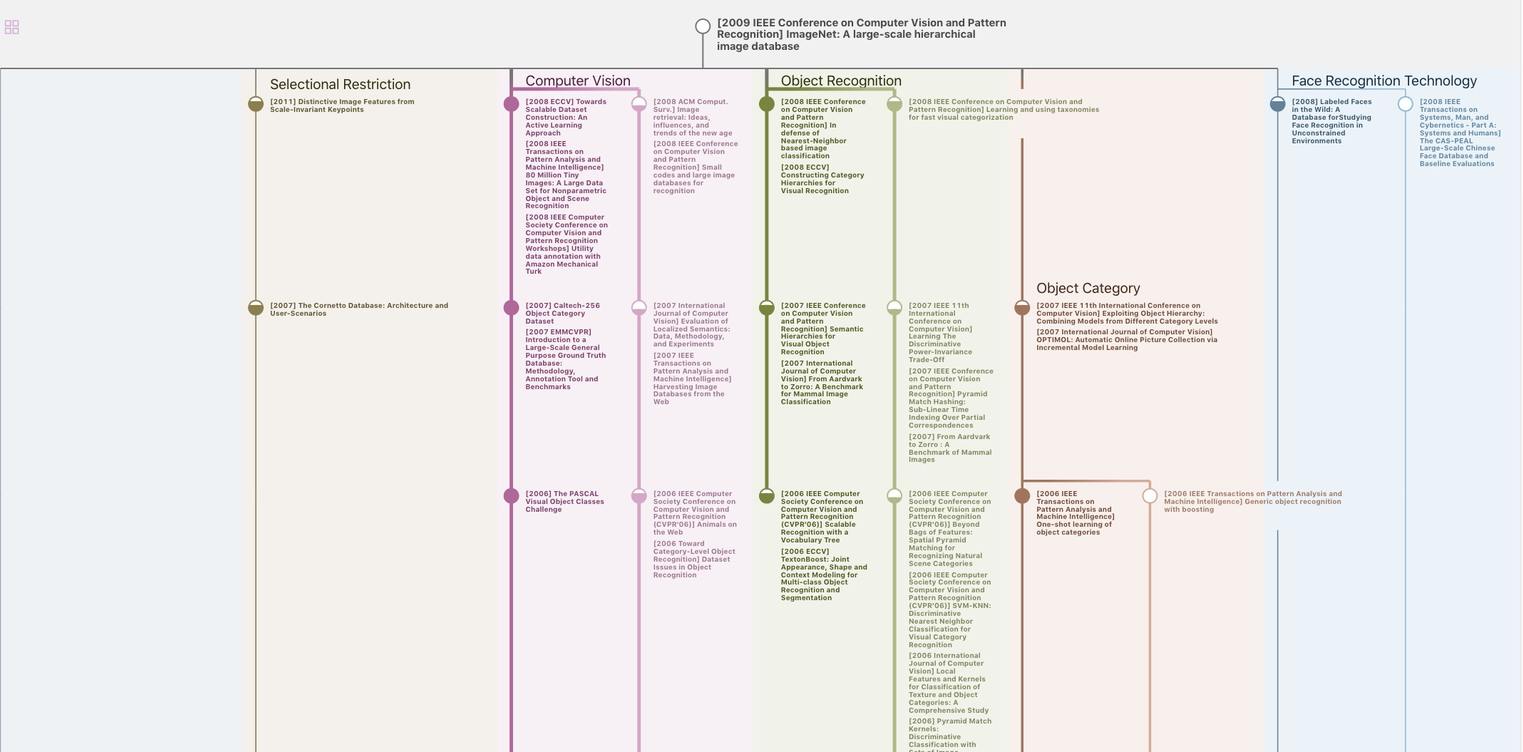
生成溯源树,研究论文发展脉络
Chat Paper
正在生成论文摘要