Semi-supervised active learning hypothesis verification for improved geometric expression in three-dimensional object recognition.
Eng. Appl. Artif. Intell.(2023)
摘要
Efficient three-dimensional (3D) object recognition plays an important role in the 3D reconstruction of light -field displays. However, presently, the error rate of 3D implicit shape object recognition remains high, because the local features are sparse in the geometric expression of 3D reconstruction. To address this issue, a hypothesis verification method based on semi-supervised active learning-based K-means++ combined with 3D feature extraction is proposed. The proposed approach consists of the offline and online phases. The algorithm time complexity is O(n) and O(n2), respectively. The offline phase includes keypoint detection, normal estimation, fast point feature histograms (FPFH) descriptor extraction, geometric word weight saving, and indexing structure construction. In addition to the FPFH extraction, the online phase includes nearest geometric word searching, corresponding direction and center voting, and non-maximum suppression. Comparative experiments were conducted in which the models and scenes were tested on the 3D datasets Mian and Tosca that is high-resolution. The experimental results demonstrate that the proposed method resolves the low recognition rate problem of 3D implicit objects, with the highest 3D intersection over union (IoU) reaching 88.89%.
更多查看译文
关键词
K-means plus plus,Hypothesis verification,3D object recognition,Geometric expression,FPFH descriptor extraction
AI 理解论文
溯源树
样例
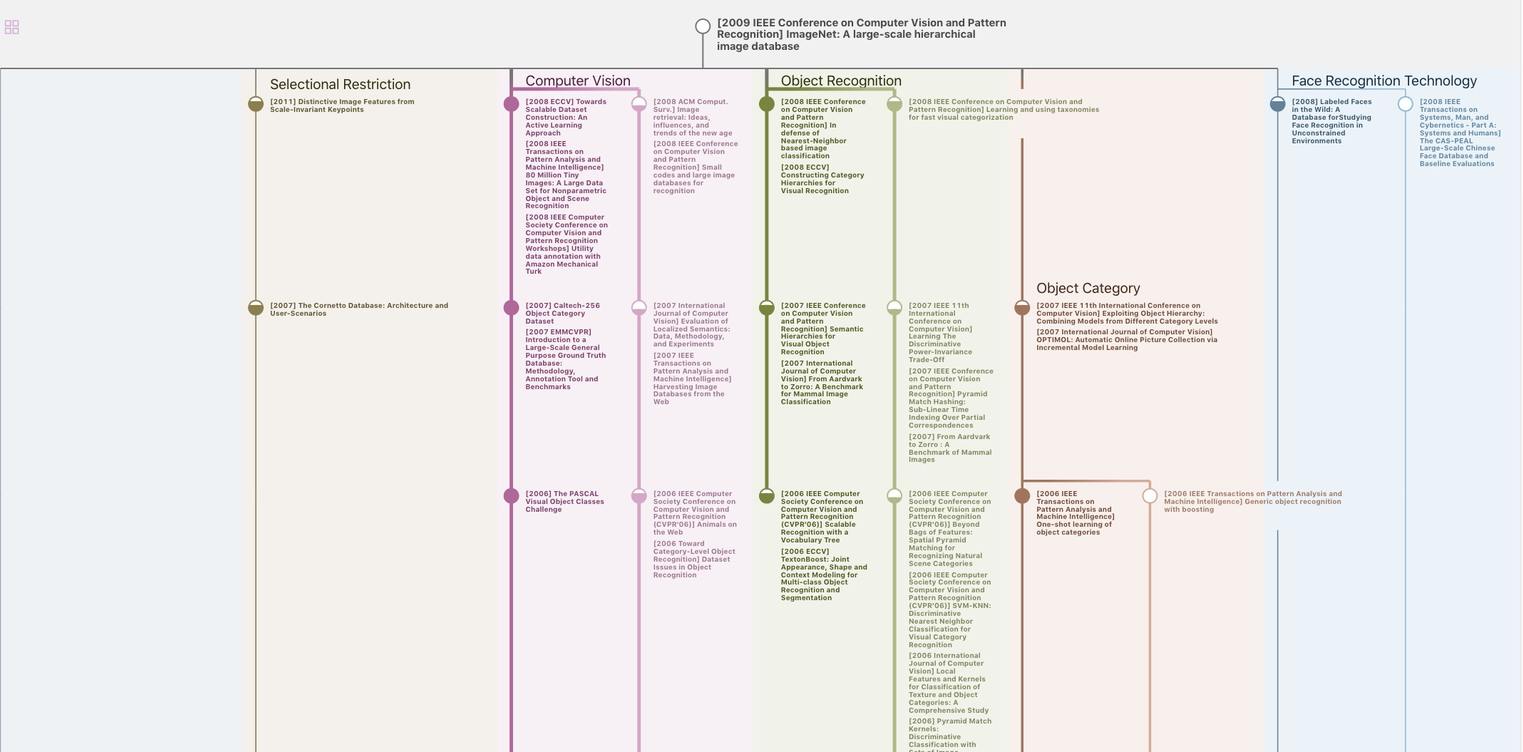
生成溯源树,研究论文发展脉络
Chat Paper
正在生成论文摘要