Malware Classification and Detection using Quantum Neural Network (QNN).
SIGCSE (2)(2023)
Abstract
Quantum Machine Learning (QML) is an emerging field that involves training a parameterized quantum circuit in order to analyze quantum or classical datasets. QML has generated great excitement in recent years with the aim to develop Machine Learning (ML) models specifically designed for quantum computers that would allow exponential advantage in making accurate predictions on quantum data. Cybersecurity threats are evolving with the advancement of computing technology concurrently. Utilizing the concept of proactive prevention and early detection of security vulnerabilities may provide advantages to mitigate cybersecurity risk. In this paper, we adopt the Quantum Neural Network (QNN), a subset of QML for malware classification and detection. We use Google Collab as IDE and utilize an open-source ClaMP Dataset from Kaggle. We demonstrate our repository to classify and detect malware using a quantum neural network (QNN) and the result indicates that we achieved an accuracy of 94 % which shows initial efficiency in quantum machine learning (QML) that would improve over time.
MoreTranslated text
AI Read Science
Must-Reading Tree
Example
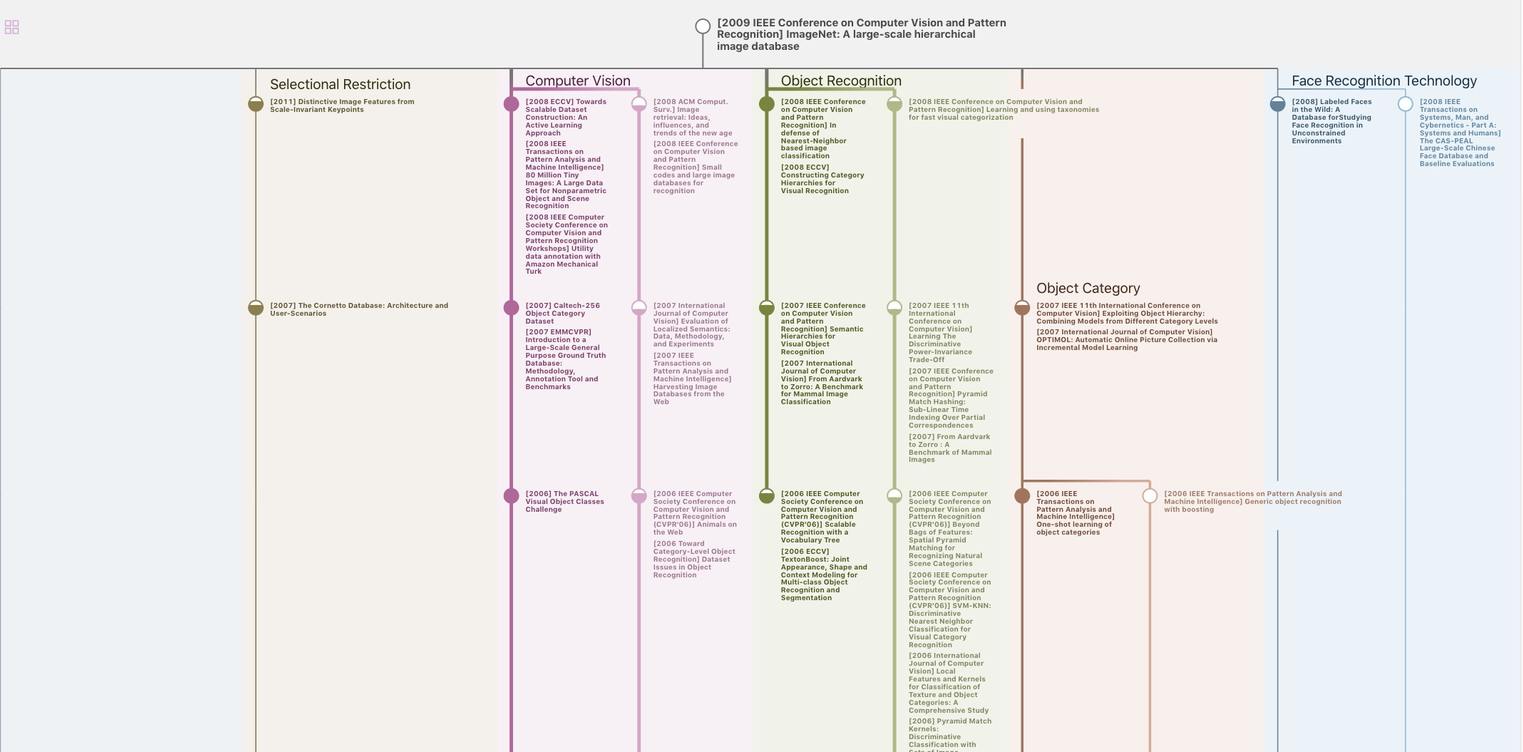
Generate MRT to find the research sequence of this paper
Chat Paper
Summary is being generated by the instructions you defined