Ensemble Machine Learning for Mapping Tree Species Alpha-Diversity Using Multi-Source Satellite Data in an Ecuadorian Seasonally Dry Forest
Remote. Sens.(2023)
摘要
The increased variety of satellite remote sensing platforms creates opportunities for estimating tropical forest diversity needed for environmental decision-making. As little as 10% of the original seasonally dry tropical forest (SDTF) remains for Ecuador, Peru, and Colombia. Remnant forests show high rates of species endemism, but experience degradation from climate change, wood-cutting, and livestock-grazing. Forest census data provide a vital resource for examining remote sensing methods to estimate diversity levels. We used spatially referenced trees >= 5 cm in diameter and simulated 0.10 ha plots measured from a 9 ha SDTF in southwestern Ecuador to compare machine learning (ML) models for six alpha-diversity indices. We developed 1 m tree canopy height and elevation models from stem mapped trees, at a scale conventionally derived from light detection and ranging (LiDAR). We then used an ensemble ML approach comparing single-and combined-sensor models from RapidEye, Sentinel-2 and interpolated canopy height and topography surfaces. Validation data showed that combined models often outperformed single-sensor approaches. Combined sensor and model ensembles for tree species richness, Shannon's H, inverse Simpson's, unbiased Simpson's, and Fisher's alpha indices typically showed lower root mean squared error (RMSE) and increased goodness of fit (R-2). Pielou's J, a measure of evenness, was poorly predicted. Mapped tree species richness (R2 = 0.54, F = 27.3, p = < 0.001) and Shannon's H' (R-2 = 0.54, F = 26.9, p = < 0.001) showed the most favorable agreement with field validation observations (n = 25). Small-scale model experiments revealed essential relationships between dry forest tree diversity and data from multiple satellite sensors with repeated global coverage that can help guide larger-scale biodiversity mapping efforts.
更多查看译文
关键词
seasonally dry tropical forest,alpha-diversity,multispectral imagery,interpolated canopy height and elevation models,ensemble machine learning
AI 理解论文
溯源树
样例
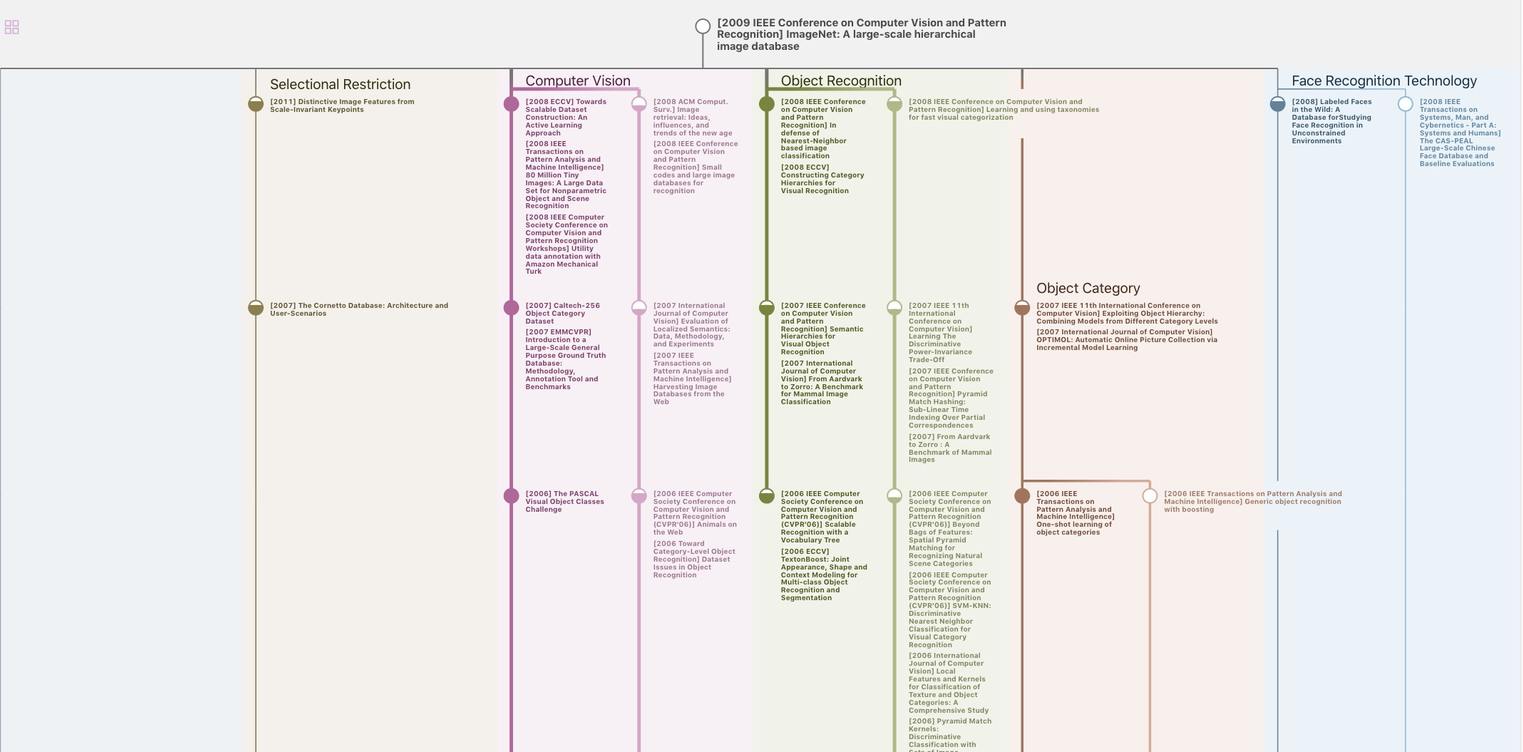
生成溯源树,研究论文发展脉络
Chat Paper
正在生成论文摘要