A Scaling Law for Syn2real Transfer: How Much Is Your Pre-training Effective?
ECML/PKDD (3)(2022)
Abstract
Synthetic-to-real transfer learning is a framework in which a synthetically generated dataset is used to pre-train a model to improve its performance on real vision tasks. The most significant advantage of using synthetic images is that the ground-truth labels are automatically available, enabling unlimited expansion of the data size without human cost. However, synthetic data may have a huge domain gap, in which case increasing the data size does not improve the performance. How can we know that? In this study, we derive a simple scaling law that predicts the performance from the amount of pre-training data. By estimating the parameters of the law, we can judge whether we should increase the data or change the setting of image synthesis. Further, we analyze the theory of transfer learning by considering learning dynamics and confirm that the derived generalization bound is consistent with our empirical findings. We empirically validated our scaling law on various experimental settings of benchmark tasks, model sizes, and complexities of synthetic images.
MoreTranslated text
Key words
syn2real transfer,scaling law,pre-training
AI Read Science
Must-Reading Tree
Example
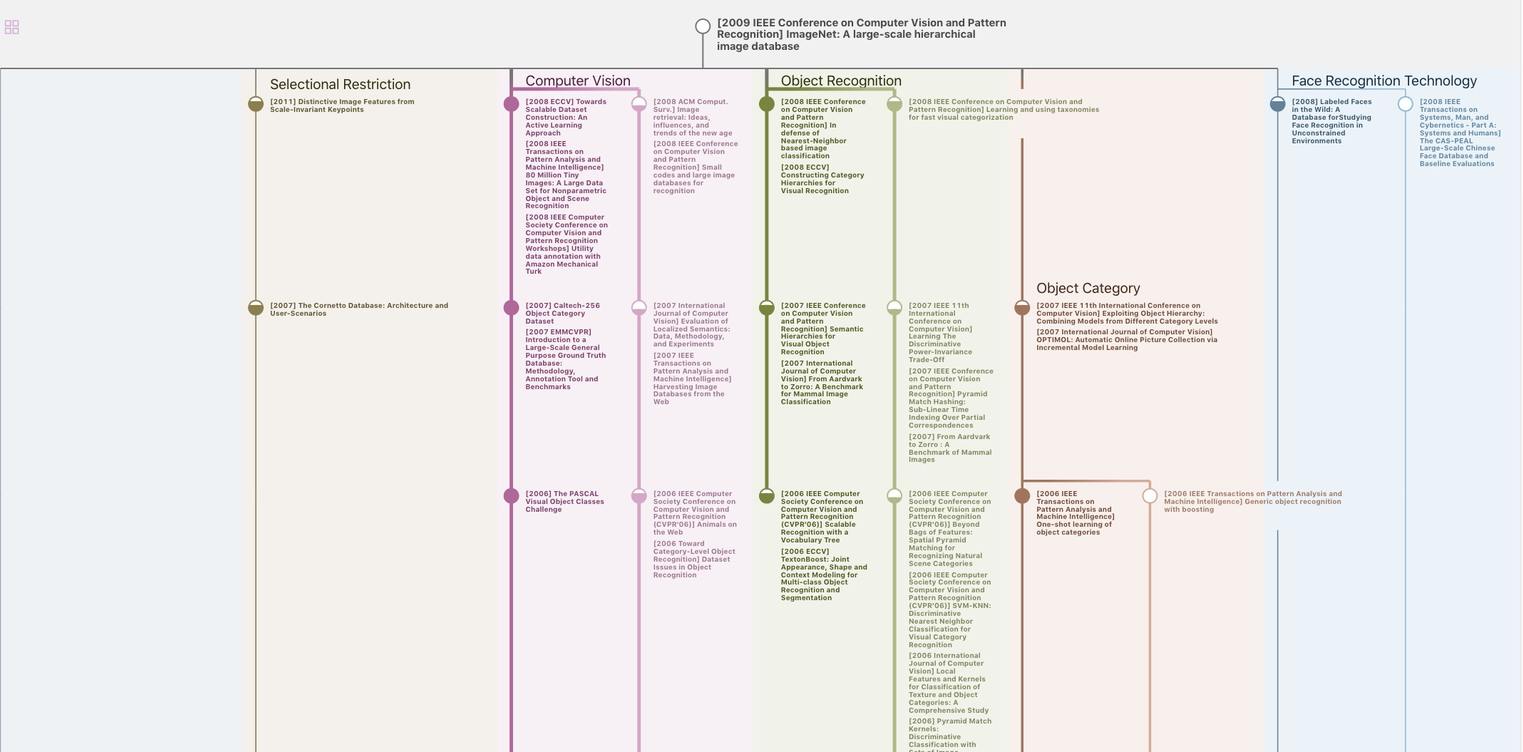
Generate MRT to find the research sequence of this paper
Chat Paper
Summary is being generated by the instructions you defined