Identification of the Best Accelerometer Features and Time-Scale to Detect Disturbances in Calves.
AALTD@ECML/PKDD(2022)
摘要
While human activity has received much attention in time-series analysis research, animal activity has received much less attention. The monitoring of cattle in Precision Livestock Farming is a promising application area as it is reasonable to expect that cattle will be equipped with low-cost sensors for animal welfare reasons. In this paper, we present work on feature selection to detect disturbance in calves such as diseases or stressful events from accelerometer sensors attached to a neck-collar. While the long-term objective is to generate an alert when a disturbance is detected, the work presented here focuses on identifying the most discriminating accelerometer features to detect activity changes associated with a calf stressful event. For that purpose, we used accelerometer data from 47 calves who were dehorned at 16 days +/- 10 days, a routine procedure known to be painful and stressful for calves. We calculated 7 primary features that could change after an occurrence of a disturbance, within a 24 h period before and after dehorning for each calf, falling under the areas of energy expenditure and the structure of the calf activity. These features were explored under 17 time-scales from 1 s to 24 h to find the best time-scale associated with each feature. First filtering with Mutual Information (MI) and Gini index was applied to reduce the candidate set of features for the second features selection with Random Forest Features Importance (RFFI). Performance were evaluated with Random Forest, k-Nearest Neighbor and Gaussian Naive Bayes models on test-sets to assess the relevancy of the selected features. Performance of all classifiers is improved or maintained when features from MI and Gini selection are used but decreased when further feature reduction with RFFI is applied. Therefore, based on MI and Gini selection, the most discriminating features are linked to activity peaks (maximum), amount of dynamic behaviors (standard-deviation) and activity structure (spectral entropy, Hurst exponent) with time-scales ranging from 1 s to 24 h depending on the features, which is consistent with animal welfare literature.
更多查看译文
关键词
best accelerometer features,disturbances,time-scale
AI 理解论文
溯源树
样例
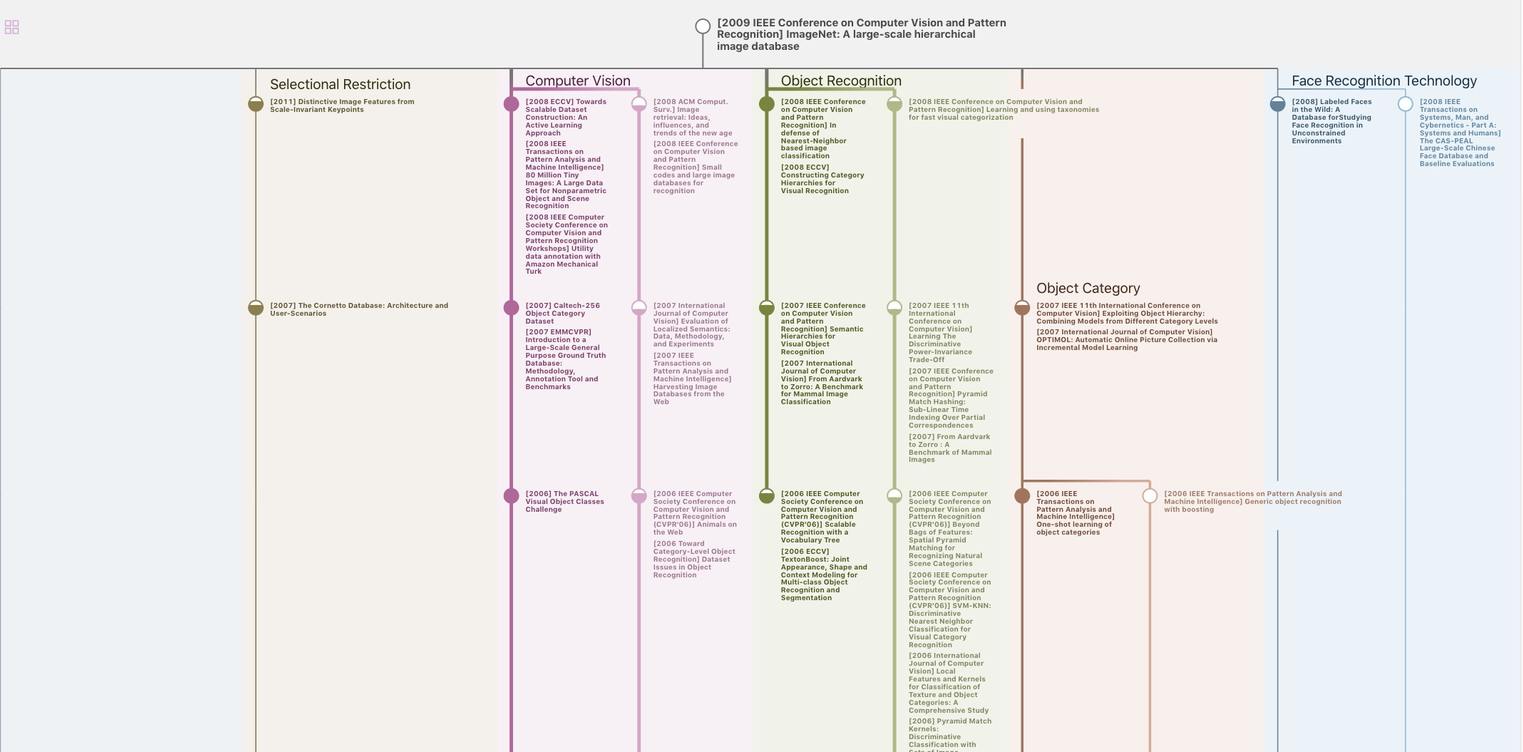
生成溯源树,研究论文发展脉络
Chat Paper
正在生成论文摘要