AD-AUG: Adversarial Data Augmentation for Counterfactual Recommendation.
ECML/PKDD (1)(2022)
摘要
Collaborative filtering (CF) has become one of the most popular and widely used methods in recommender systems, but its performance degrades sharply in practice due to the sparsity and bias of the real-world user feedback data. In this paper, we propose a novel counter-factual data augmentation framework AD-AUG to mitigate the impact of the imperfect training data and empower CF models. The key idea of AD-AUG is to answer the counterfactual question: "what would be a user's feedback if his previous purchase history had been different?". Our framework is composed of an augmenter model and a recommender model. The augmenter model aims to generate counterfactual user feedback based on the observed ones, while the recommender leverages the original and counterfactual user feedback data to provide the final recommendation. In particular, we design two adversarial learning-based methods from both "bottom-up" data-oriented and "top-down" model-oriented perspectives for counterfactual learning. Extensive experiments on three real-world datasets show that the AD-AUG can greatly enhance a wide range of CF models, demonstrating our framework's effectiveness and generality.
更多查看译文
关键词
Counterfactual augmentation, Collaborative filtering, Recommending systems
AI 理解论文
溯源树
样例
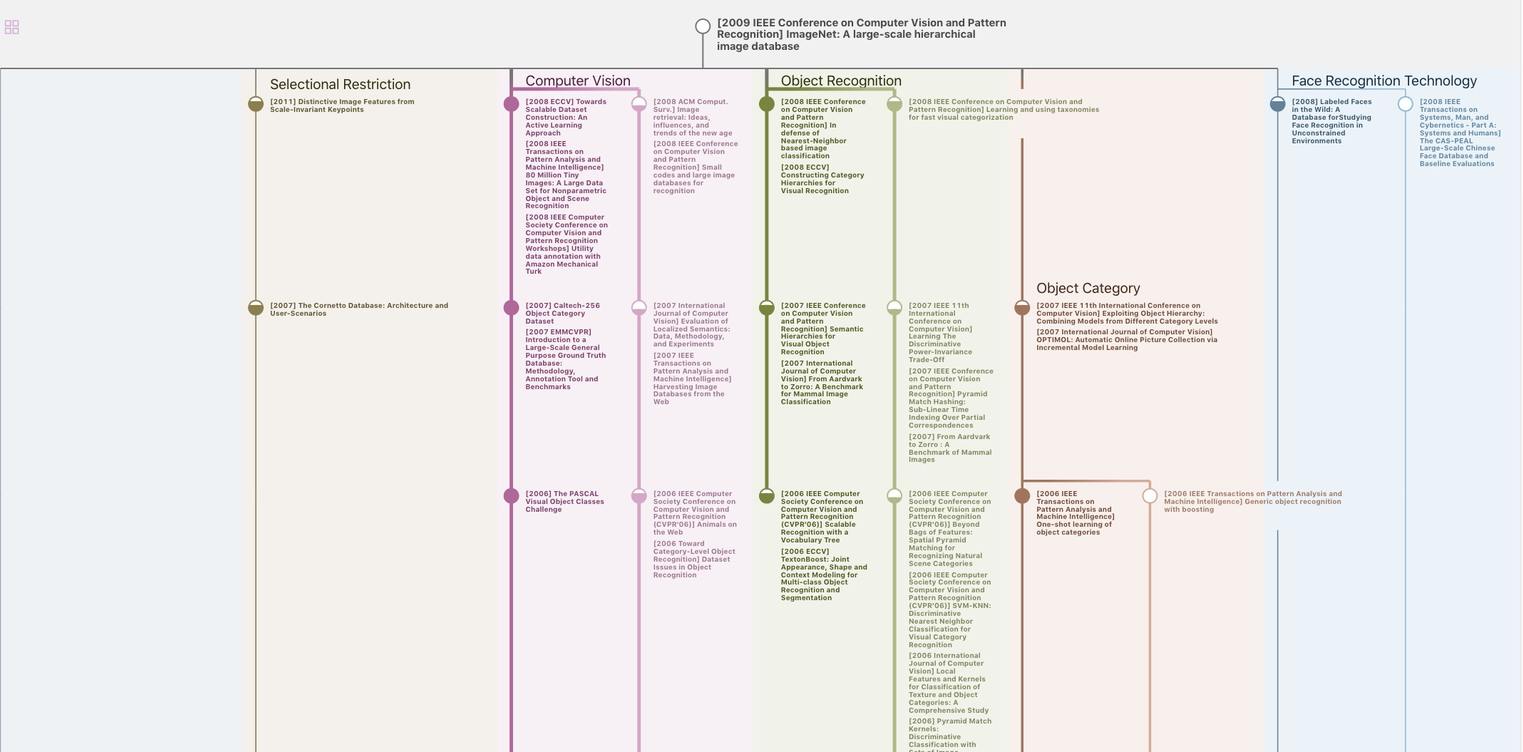
生成溯源树,研究论文发展脉络
Chat Paper
正在生成论文摘要