OpenLog: Incremental Anomaly Classification with Changing, Unbalanced and Unknown Logs.
ISPA/BDCloud/SocialCom/SustainCom(2022)
摘要
With the development of Artificial Intelligence for IT Operations(AIOps), an increasing number of log analysis approaches have been proposed to guarantee the stability and reliability of large-scale systems. However, traditional approaches still exist some limitations. First, they cannot adapt to the changing log statements. Second, most of them are based on large-scale labeled data. Third, most of their frames are fixed. To overcome these limitations, we propose OpenLog based on meta-learning and few-shot learning. Experimental results show that OpenLog can achieve an accuracy of 95.8% on detecting unknown anomaly classes, and an accuracy of 93.3% in anomaly classification on unbalanced logs with unknown anomaly classes. In the few-shot classification, OpenLog can still achieve an accuracy over 80%.
更多查看译文
关键词
Anomaly classification,log analysis,meta-learning,few-shot learning,AIOPs
AI 理解论文
溯源树
样例
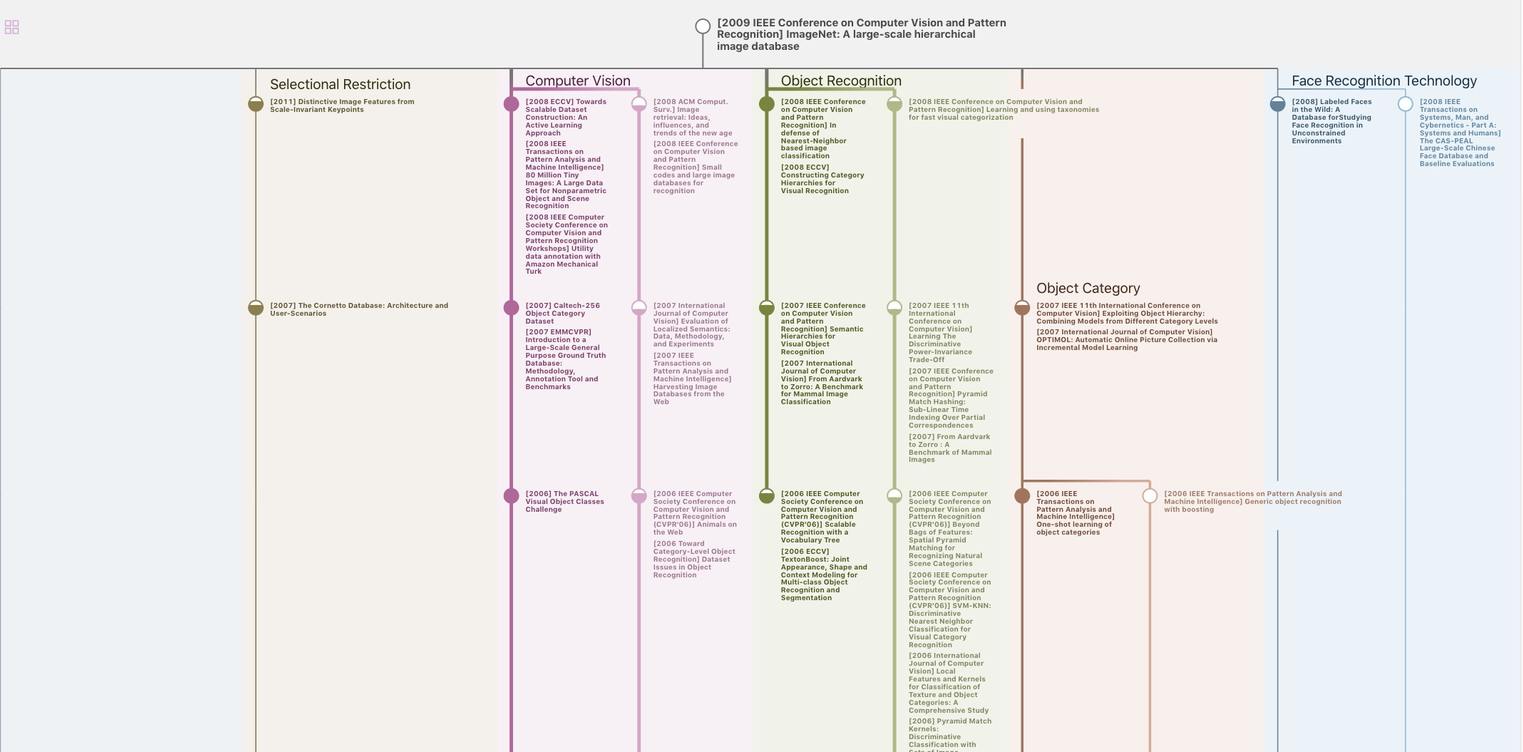
生成溯源树,研究论文发展脉络
Chat Paper
正在生成论文摘要