Love of Variety Based Latency Analysis for High Definition Map Updating: Age of Information and Distributional Robust Perspectives.
IEEE Trans. Intell. Veh.(2023)
Abstract
High definition (HD) map is a key technology that enables autonomous driving, which has the characteristic of frequent updates and low delay requirements. In order to minimize the HD map updating latency, this paper jointly considers the generation and transmission processes of HD map. The generation process of HD map relies heavily on the data captured by different types of sensors, which is modeled by the federated analytics process. Also, in order to quantify the diversity of utilized sensors, the concept of love of variety is introduced in this paper. Then, considering HD map is composed of dynamic and static layers that have different latency requirements during the transmission process, this paper proposes a method to allocate edge server capacity to each HD map layer such that the overall information staleness can be minimized. Firstly, the deterministic edge capacity case is discussed and the solution is derived by obtaining the Karush-Kuhn-Tucker conditions. Then, considering the practice that an edge server provides services to multiple attached devices simultaneously, contentions among these devices make available capacity for the autonomous vehicle variational. Therefore, an uncertain edge capacity case is discussed as well, where the uncertainty is described by Wasserstein metrics and the problem is reformulated into distributional robust chance constrained optimization problem. And for the low latency purpose, we utilize an inner approximation to reduce the complexity of the original problem and find a suboptimal solution. Finally, simulation results demonstrate the effectiveness of our proposed methods that achieves the lowest latency for HD map updating.
MoreTranslated text
Key words
High definition map,autonomous driving,federated analytics,love of variety,age of information,distributional robust chance constrained optimization
AI Read Science
Must-Reading Tree
Example
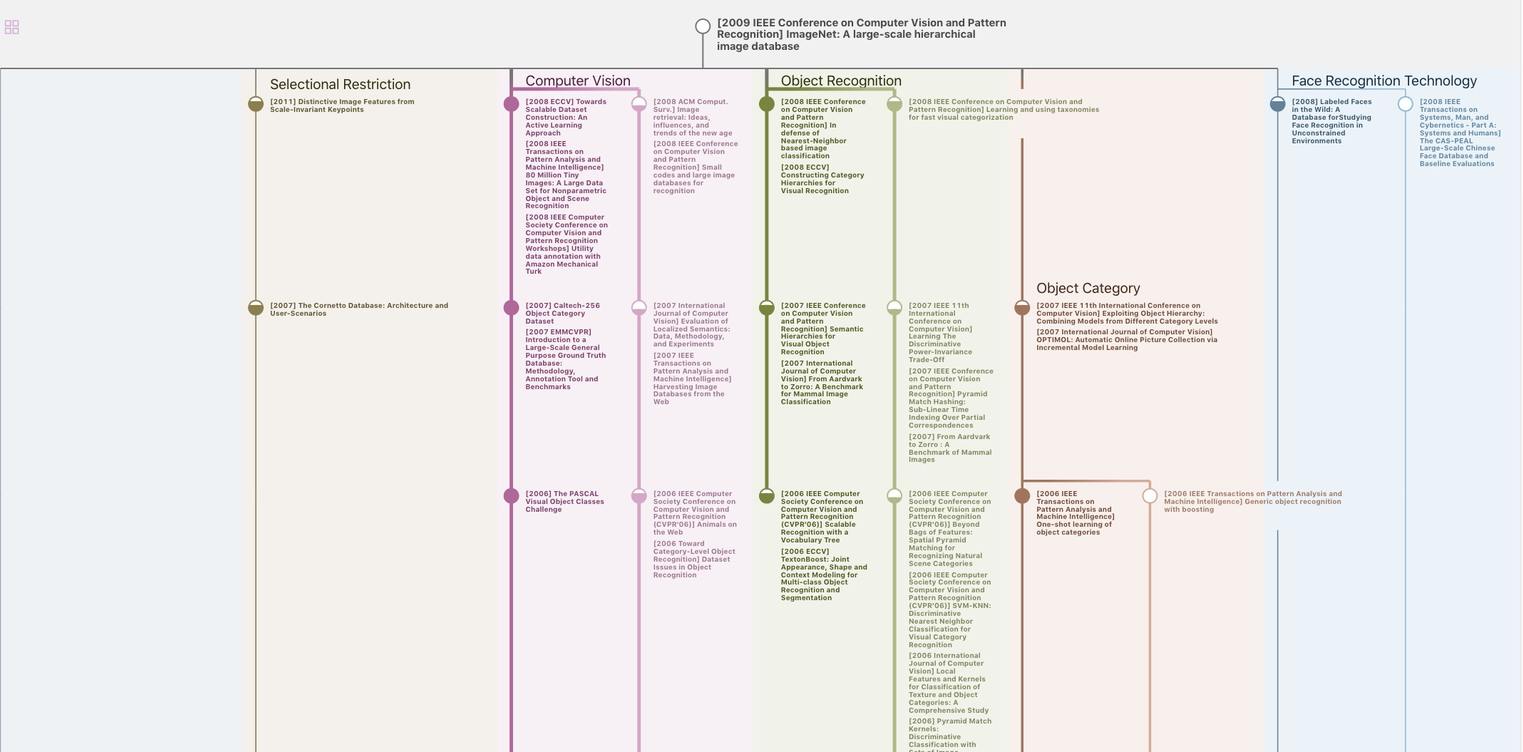
Generate MRT to find the research sequence of this paper
Chat Paper
Summary is being generated by the instructions you defined