A Multi-source Information Graph-based Web Service Recommendation Framework for a Web Service Ecosystem.
J. Web Eng.(2022)
摘要
Web service recommendation remains a highly demanding yet challenging task in the field of services computing. In recent years, researchers have started to employ side information comprised in a heterogeneous Web service ecosystem to address the issues of data sparsity and cold start in Web service recommendation. Some recent works have exploited the deep learning tech-niques to learn user/Web service representations accumulating information from multiplex sources. However, we argue that they still struggle to utilize multi-source information in a discriminating, unified and flexible manner. To tackle this problem, this paper presents a novel multi-source information graph-based Web service recommendation framework (MGASR), which can automatically and efficiently extract multifaceted knowledge from the heterogeneous Web service ecosystem. Specifically, different node-type and edge-type dependent parameters are designed to model corresponding types of objects (nodes) and relations (edges) in the Web service ecosystem. We then leverage graph neural networks (GNNs) with an attention mechanism to construct a multi-source information neural network (MIN) layer, for mining diverse significant dependencies among nodes. By stacking multiple MIN layers, each node can be characterized by a highly contextualized representation due to capturing high-order multi-source information. As such, MGASR can generate representations with rich semantic information toward supporting Web service recommendation tasks. Extensive experiments con-ducted over three real-world Web service datasets demonstrate the superior performance of our proposed MGASR as compared to various baseline methods.
更多查看译文
关键词
web service recommendation framework,web service ecosystem,web service,multi-source,graph-based
AI 理解论文
溯源树
样例
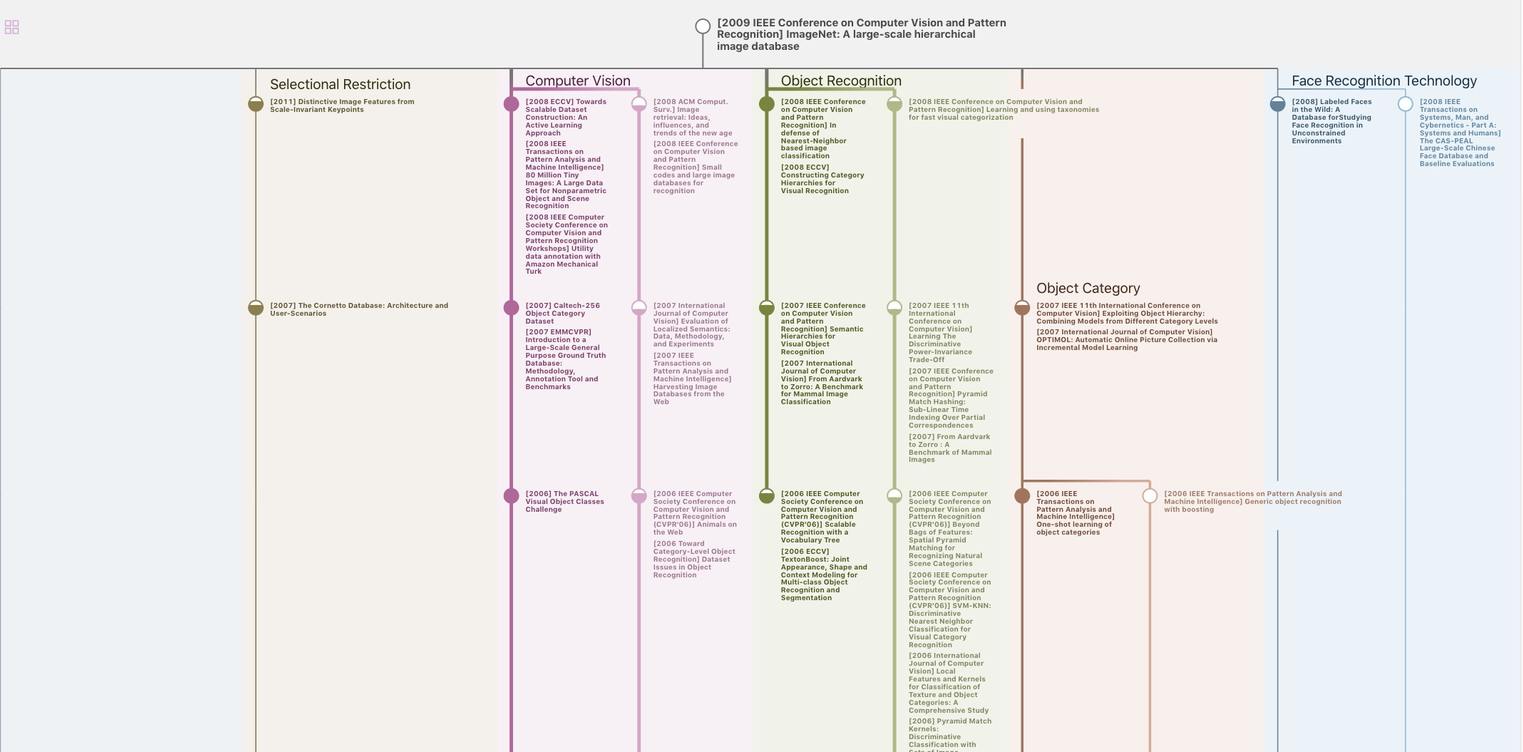
生成溯源树,研究论文发展脉络
Chat Paper
正在生成论文摘要