Control Chart Patterns (CCPs) Forecasting Using Probabilistic Deep Learning.
ICSRS(2022)
摘要
Control chart, a powerful tool of statistical process control, monitors efficiently the production quality. In practice, it is realized that each pattern in a control chart usually corresponds to a functional/failed state of the production process. Most existing works in the literature focus on the identification of control chart patterns (CCPs). In this paper, we propose a prognostic model for CCPs forecasting. The model is constructed based on the probabilistic long-short-term memory (LSTM) network to capture the dependencies and uncertainties of the control chart data. The performance of the proposed model is then validated through a numerical study. The prediction results can be used as a basis for the predictive maintenance decision-making and helping to avoid unexpected failures of the production process.
更多查看译文
关键词
Statistical process control,Control Chart Patterns forecasting,Probabilistic deep learning,Long short Term Memory (LSTM),Monte Carlo (MC) Dropout
AI 理解论文
溯源树
样例
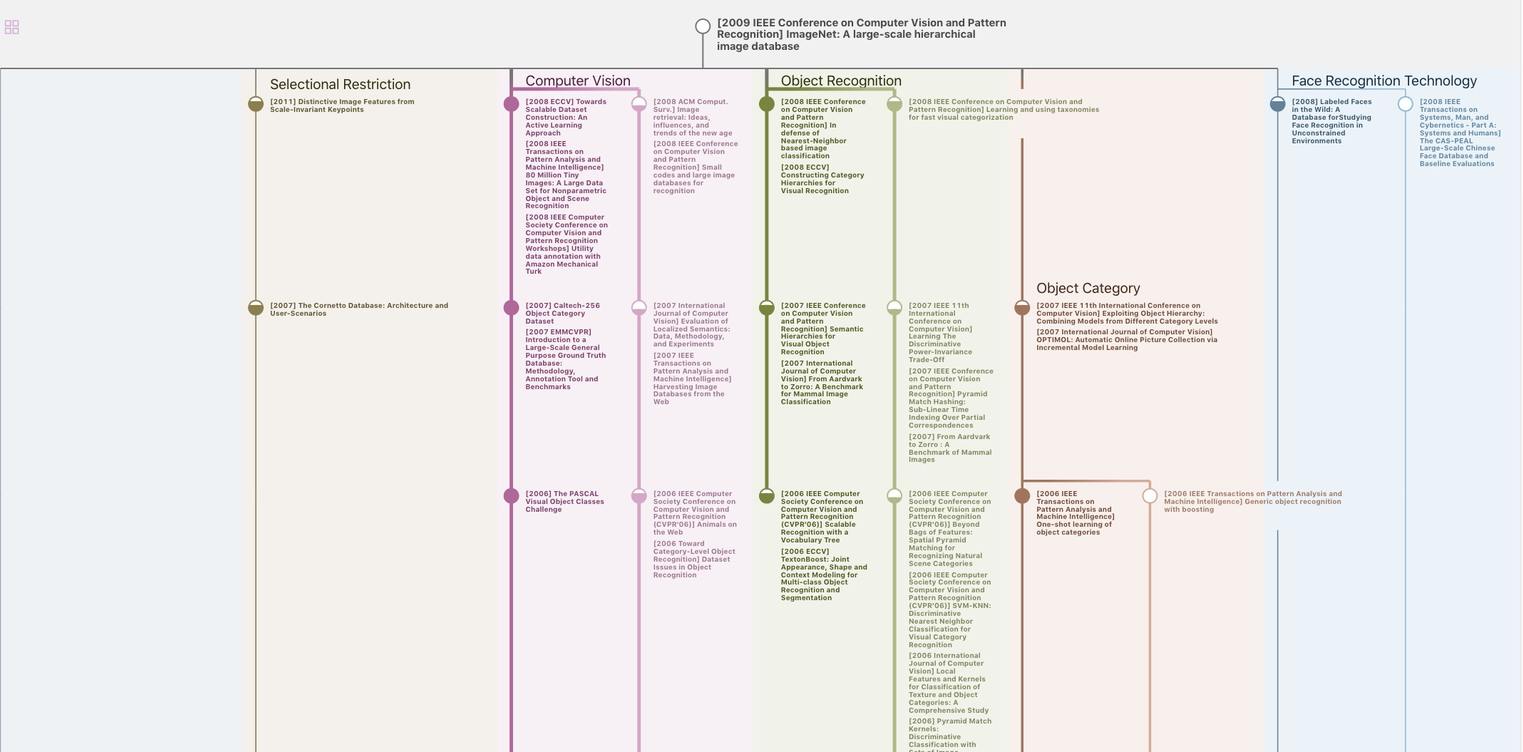
生成溯源树,研究论文发展脉络
Chat Paper
正在生成论文摘要