New RUL Prediction Method for Rotating Machinery via Data Feature Distribution and Spatial Attention Residual Network.
IEEE Trans. Instrum. Meas.(2023)
摘要
The prediction of remaining useful life (RUL) is one of the important measures to ensure the safety and reliability of mechanical equipment. Aiming at the low accuracy of residual life prediction caused by difficulty in feature extraction from high data redundancy in the monitoring process of rotating machinery, an RUL prediction method based on data feature distribution and spatial attention residual network (SARN) is proposed. In the proposed method, multisensor data is segmented utilizing distribution feature difference analysis, so as to highlight the degradation characteristics in the sample sequence. Combined with the proposed SARN based on a spatial attention mechanism and residual network, more extensive and complementary features can be extracted. It not only focuses on the importance screening of feature information from all input data, but also realizes the mapping between global sample features and labels, to improve the accuracy of RUL prediction. Finally, the method is verified by the experiments on PHM2012 and C-MAPSS datasets. The results indicate that, compared with the CNNLSTM method on the C-MAPSS dataset, the proposed method has an average reduction of 14.37% in the MAE index and an average increase of 22.59% in the R2 index. This shows that the proposed method has satisfied RUL prediction performance for rotating machinery.
更多查看译文
关键词
machinery,data feature distribution,prediction
AI 理解论文
溯源树
样例
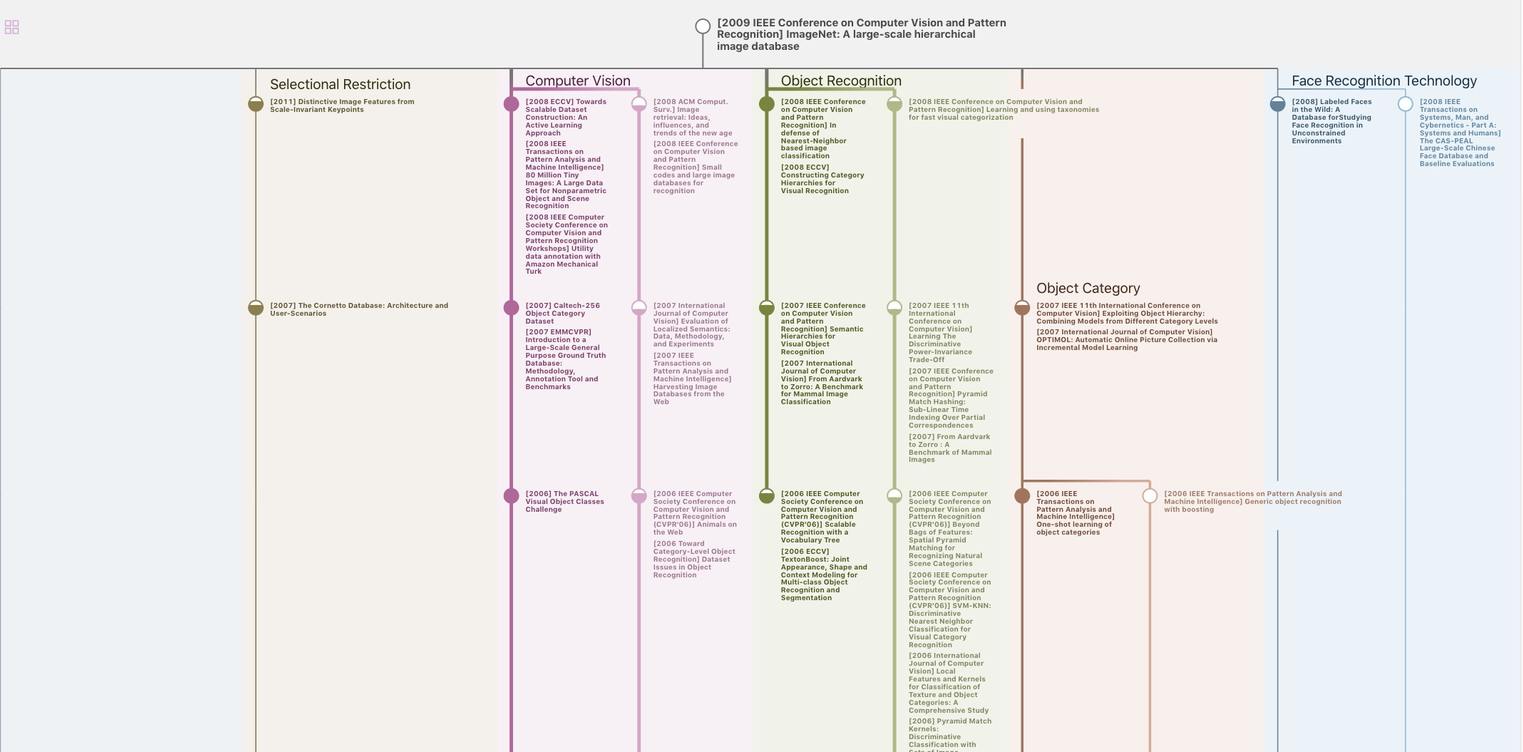
生成溯源树,研究论文发展脉络
Chat Paper
正在生成论文摘要