Detecting DDoS Attacks in SDN using a Hybrid Method with Entropy and Machine Learning.
CANDARW(2022)
摘要
Software Defined Network (SDN) brings a new concept in terms of network architecture. Despite its benefits, SDN architecture also presents new security challenges, in particular, those related to Distributed Denial of Service (DDoS) attacks. Many current approaches have used statistical techniques, such as entropy, or Machine Learning (ML) to detect these attacks. However, there is an important trade-off between these approaches. The definition of a threshold that determine whether particular traffic is spurious or not is not trivial in statistical techniques. ML solutions may provide better accuracy as compared to statistical techniques, but require considerable computational resources and time to converge. Current hybrid approaches try to balance between these two approaches by either using the results from entropy as input in ML algorithms (Entropy → ML) or using entropy as a filter and ML algorithms to identify attacks. By combining these techniques, this paper presents a 3-step solution (Entropy → ML→ Entropy) called ML-Entropy which inherits the intelligence of ML algorithms to dynamically adjust the threshold used by entropy, improving the separation of legitimate from spurious traffic with reduced error rates. The solution was implemented and evaluated in a real-corporate environment. The experimental results show that the attack detection accuracy of ML-Entropy surpasses 99%, providing superior results as compared to entropy and its combination with ML algorithms.
更多查看译文
AI 理解论文
溯源树
样例
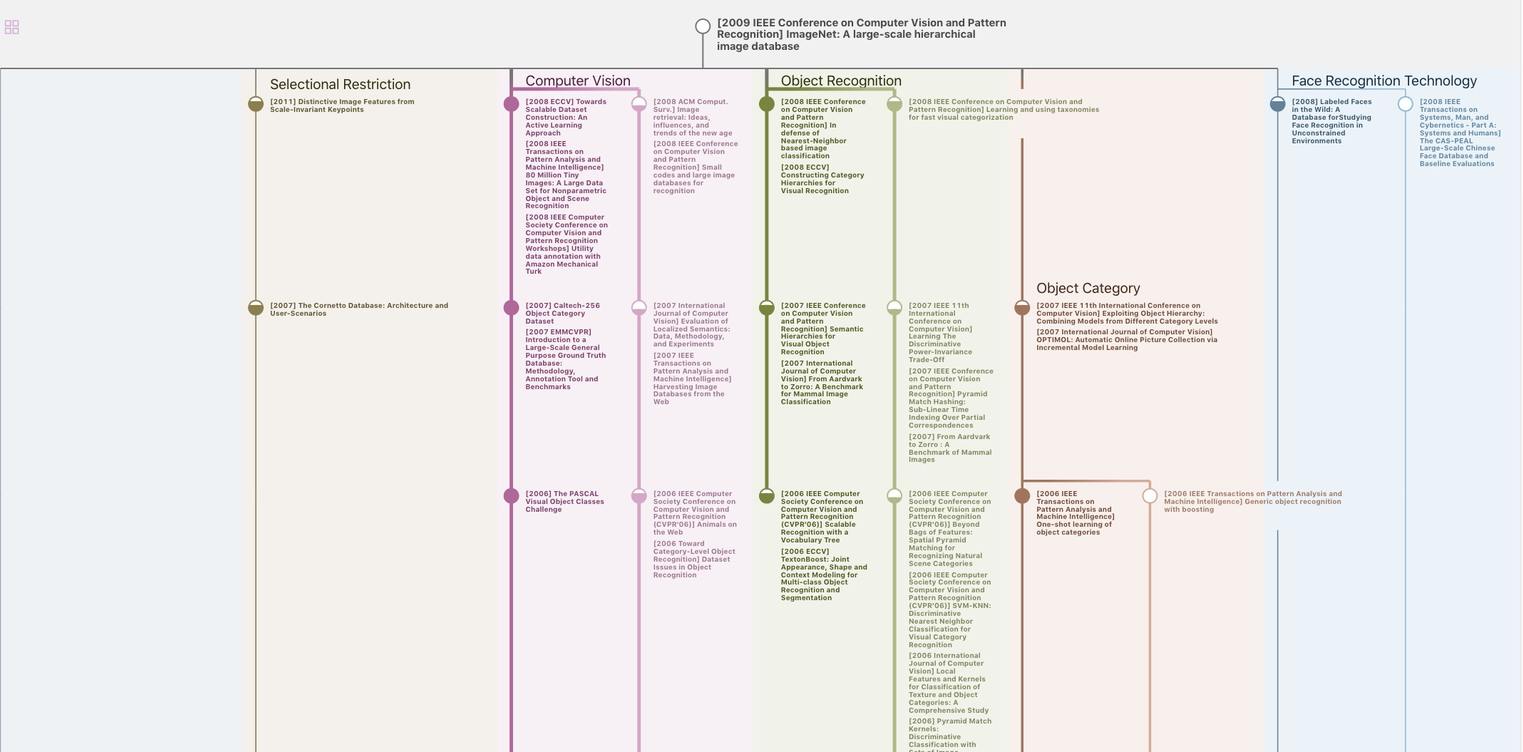
生成溯源树,研究论文发展脉络
Chat Paper
正在生成论文摘要