Contrastive Learning for Simulation-to-Real Domain Adaptation of LiDAR Data.
EUROCAST(2022)
摘要
The accuracy of supervised deep learning algorithms is heavily dependent on the availability of annotated data and, in many cases, labeling this data accurately involves a very large outlay. Consequently, simulated data becomes an enticing option, since this data can be parameterized to resemble a real environment. However, the domain shift cannot be disregarded. To tackle this problem, we present a method which formulates an cloud-to-cloud translation as an image-to-image task from simulated to real scenarios. Our approach is capable of learning to extract the best features from the geometry of the environment, encode the information into a voxelized representation and generate a version similar to the one captured by a real sensor for complete scenarios. Our results on the CARLA to SemanticKITTI translation demonstrate that our method is able to provide adequate samples that help improve the accuracy, for selected categories of the SemanticKITTI validation set, of a semantic segmentation network trained only on real data.
更多查看译文
关键词
contrastive learning,adaptation,simulation-to-real
AI 理解论文
溯源树
样例
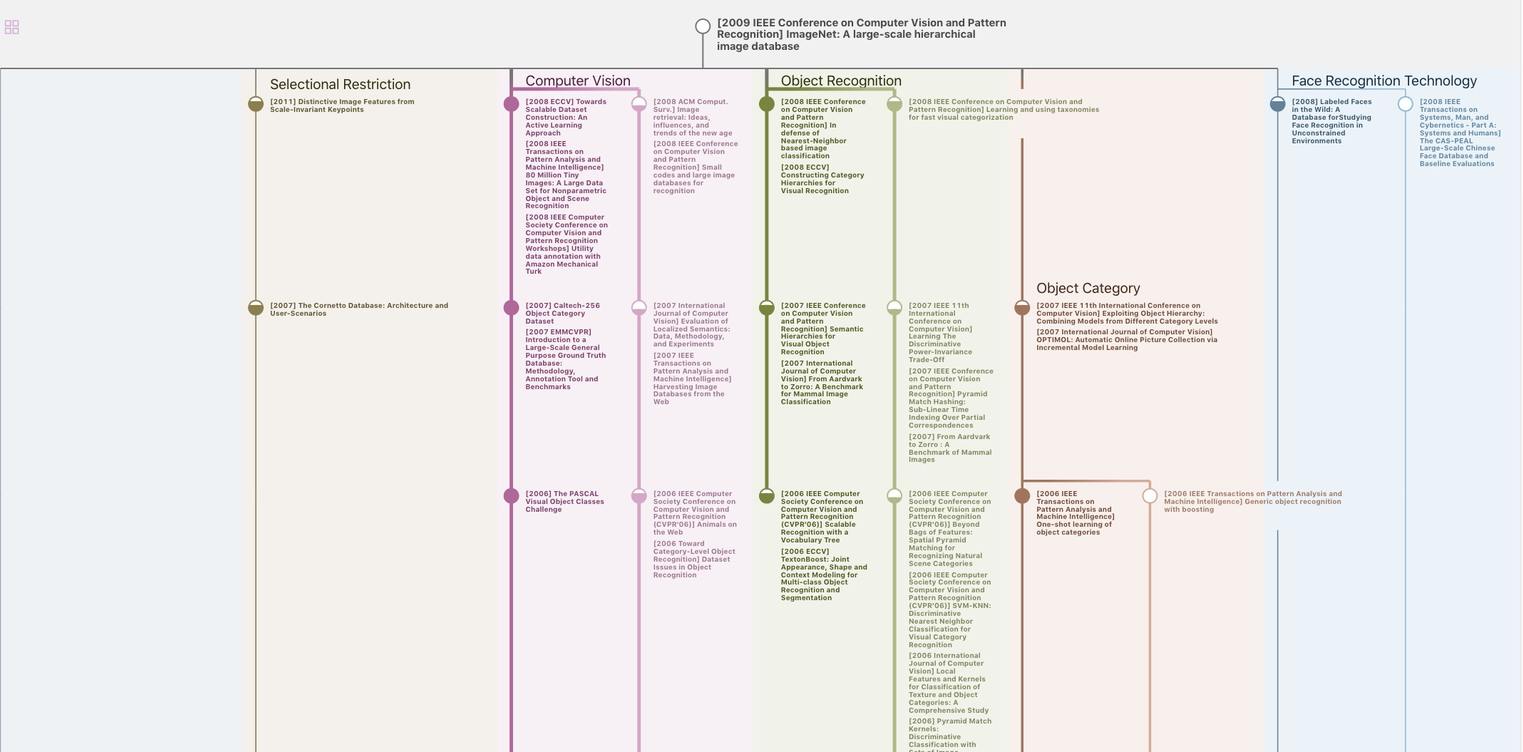
生成溯源树,研究论文发展脉络
Chat Paper
正在生成论文摘要