Non-Myopic Knowledge Gradient Policy for Ranking and Selection.
WSC(2022)
摘要
We consider the ranking and selection (R&S) problem with fixed simulation budget, in which the budget is assumed to be allocated sequentially. Deriving the optimal sampling procedure for this problem amounts to solving a stochastic dynamic program that is highly intractable. To overcome this difficulty, the existing R&S procedures are often designed from a myopic viewpoint. However, these myopic procedures are only single-step optimal and may have a poor performance for general sequential R&S problems. Therefore, in this paper, we combine two popular lookahead strategies and design a non-myopic knowledge gradient (KG) procedure. Meanwhile, to streamline the computation of procedure, we propose a modified Monte Carlo tree search method specifically designed under the R&S context. We show that the new procedure can exhibit a performance superior to the classic KG.
更多查看译文
关键词
ranking,knowledge,selection,non-myopic
AI 理解论文
溯源树
样例
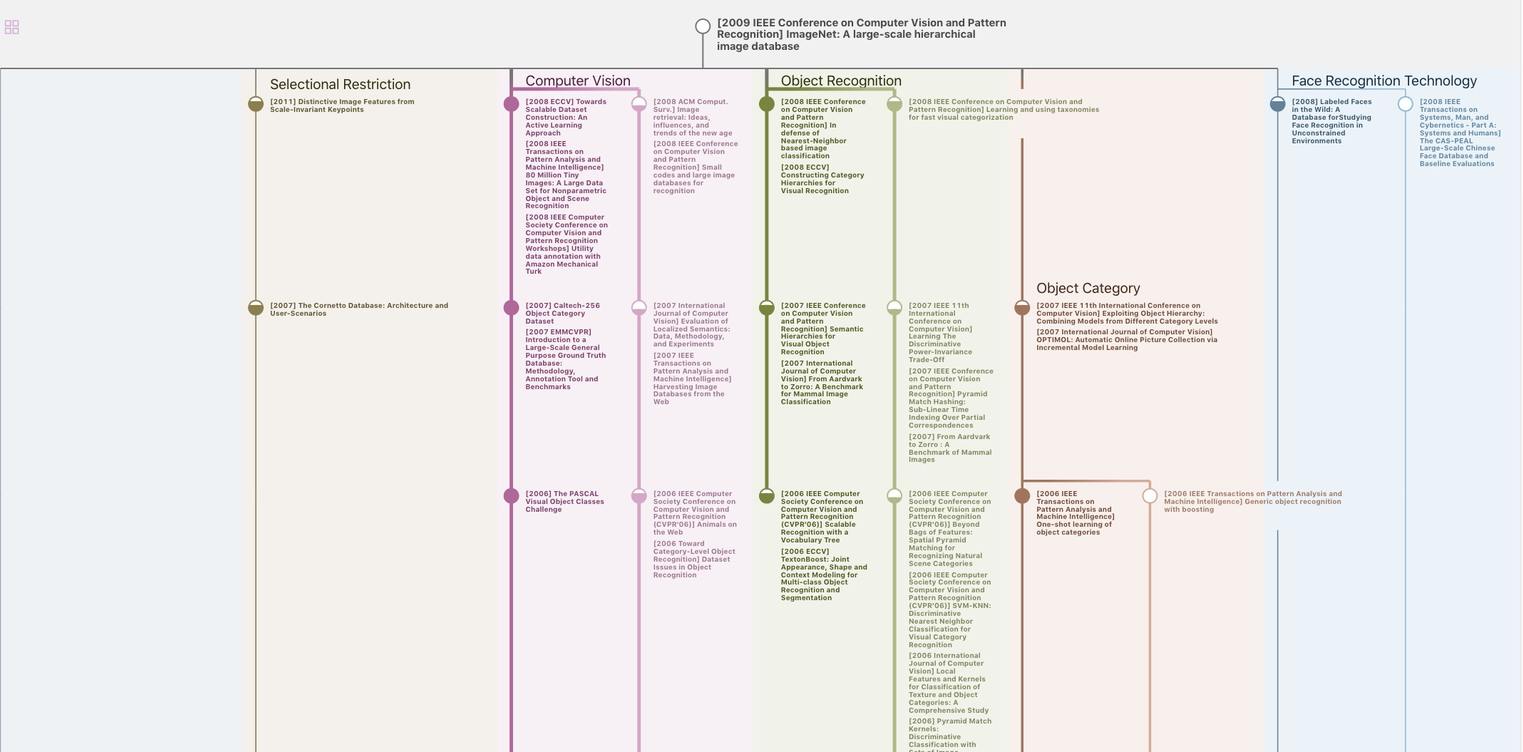
生成溯源树,研究论文发展脉络
Chat Paper
正在生成论文摘要