UNet-eVAE: Iterative Refinement Using VAE Embodied Learning for Endoscopic Image Segmentation.
MLMI@MICCAI(2022)
摘要
While endoscopy is routinely used for surveillance, high operator dependence demands robust automated image analysis methods. Automated segmentation of region-of-interest (ROI) that includes lesions, inflammations, and instruments can serve to cope with the operator dependence problem in this field. Most supervised methods are developed by fitting models on the available ground truth mask samples only. This work proposes a joint training approach using the UNet coupled with a variational auto-encoder (VAE) to improve endoscopic image segmentation by exploiting original samples, predicted masks and ground truth masks. In the proposed UNet-eVAE, VAE utilises the masks to constrain ROI-specific feature representations for reconstruction as an auxiliary task. The fine-grained spatial information from VAE is fused with the UNet decoder to enrich the feature representations and improve segmentation performance. Our experimental results on both colonoscopy and ureteroscopy datasets demonstrate that the proposed architecture can learn robust representations and generalise segmentation performance on unseen samples while improving the baseline.
更多查看译文
关键词
Endoscopy,Variational autoencoder,Segmentation,Polyp,Colonoscopy,Kidney stone,Ureteroscopy,Laser lithotripsy
AI 理解论文
溯源树
样例
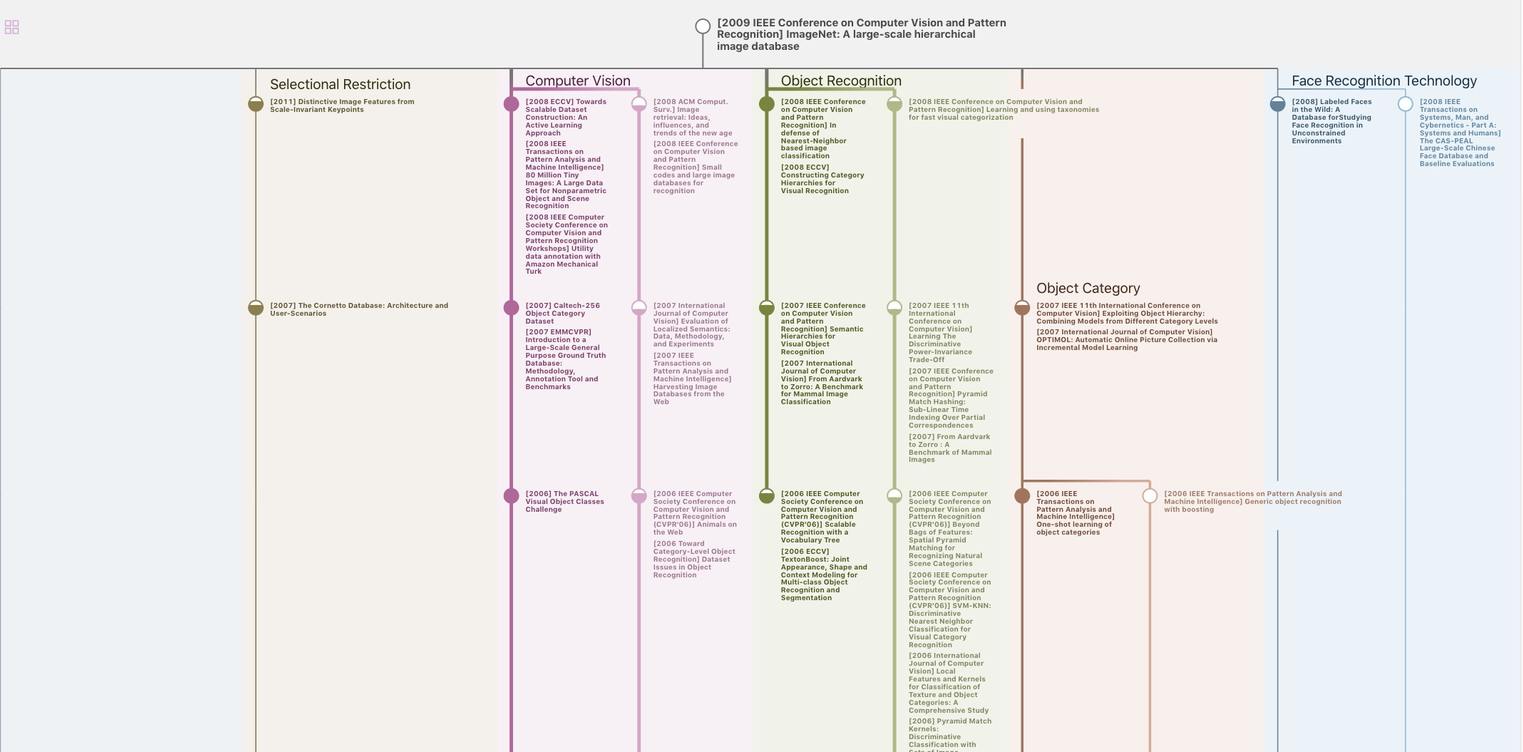
生成溯源树,研究论文发展脉络
Chat Paper
正在生成论文摘要