Abdominal CT Organ Segmentation by Accelerated nnUNet with a Coarse to Fine Strategy.
FLARE@MICCAI(2022)
摘要
Abdominal CT organ segmentation is known to be challenging. The segmentation of multiple abdominal organs enables quantitative analysis of different organs, providing invaluable input for computeraided diagnosis (CAD) systems. Based on nnUNet, we develop an abdominal organ segmentation method applicable to both abdominal CT and whole-body CT data. The proposed new training pipeline combines the Kullback-Leibler semi-supervised learning and fully supervised learning, and employs a coarse to fine strategy and GPU accelerated interpolation. Our method achieves a mean Dice Similarity Coefficient (DSC) of 0.873/0.870 and a Normalized Surface Dice (NSD) of 0.911/0.915 on the FLARE 2022 validation/test dataset, with an average process time of 12.27 s per case. Overall, we ranked the fifth place in the FLARE 2022 Challenge. The code is available at https://github.com/Solor- pikachu/ Infer-MedSeg- With- Low-Resource.
更多查看译文
关键词
segmentation,ct
AI 理解论文
溯源树
样例
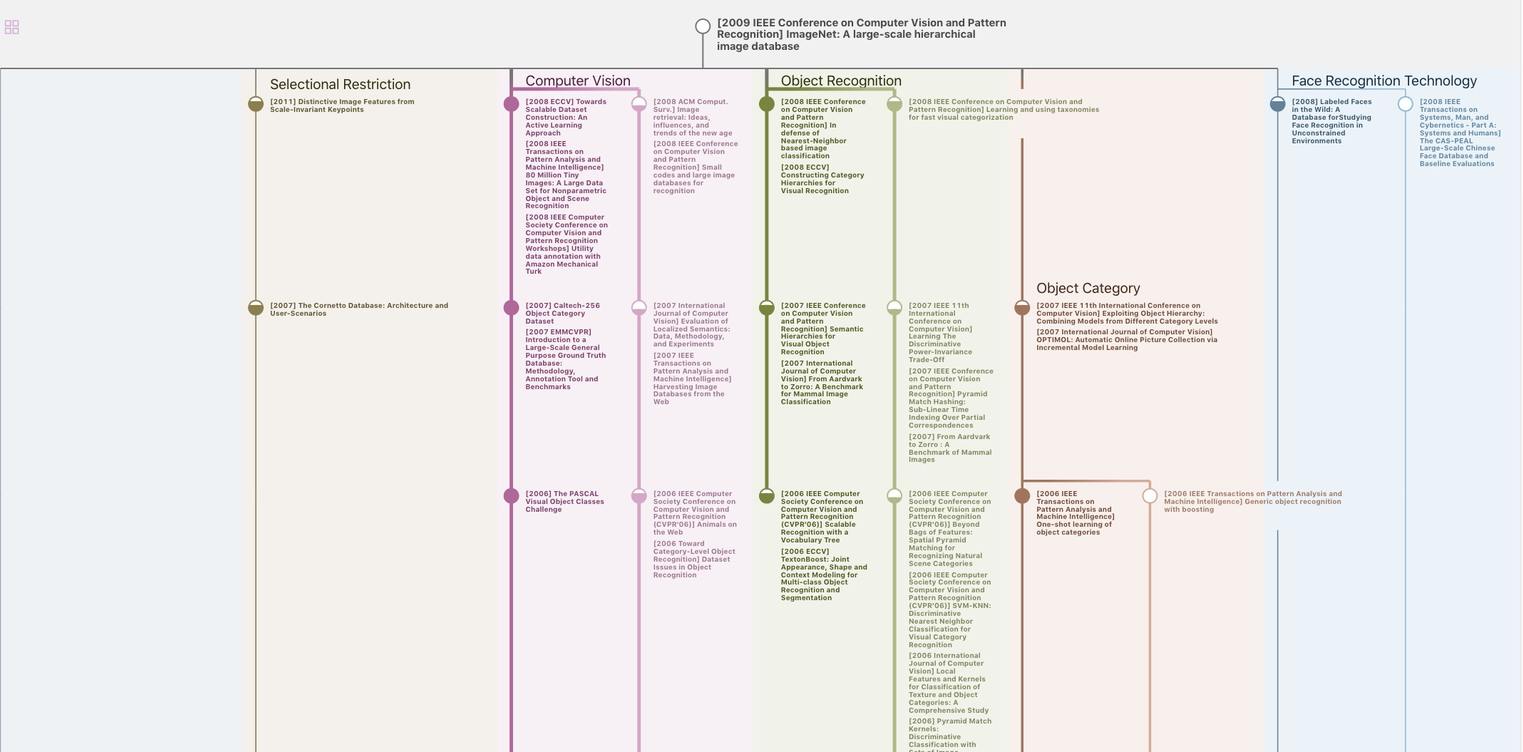
生成溯源树,研究论文发展脉络
Chat Paper
正在生成论文摘要