Investigating the Performances of Control Parameterizations for Nonlinear Model Predictive Control
ICARCV(2022)
摘要
Solving Direct Shooting Model Predictive Control (MPC) optimization problems online can be computationally expensive if a large horizon is used while also maintaining a dense time sampling. In these cases, it is accepted that tradeoffs between computational load and performances should be sought in order to meet real-time feasibility requirements. However, making the problem more tractable for the hardware should not necessarily imply a decrease in performances. One technique that has been proposed in the literature makes use of control input parameterizations to decrease the numerical complexity of nonlinear MPC problems without necessarily affecting the performances significantly. In this paper, we review the use of parameterizations and propose a simple Sequential Quadratic Programming algorithm for nonlinear MPC. We then benchmark the performances of the solver in simulation, showing that parameterizations allow to attain good performances with (significantly) lower computation times than state-of-the-art solvers.
更多查看译文
关键词
computational load,control input parameterizations,Control parameterizations,dense time sampling,Direct Shooting Model Predictive Control optimization problems,lower computation times,nonlinear Model Predictive Control,nonlinear MPC problems,real-time feasibility requirements
AI 理解论文
溯源树
样例
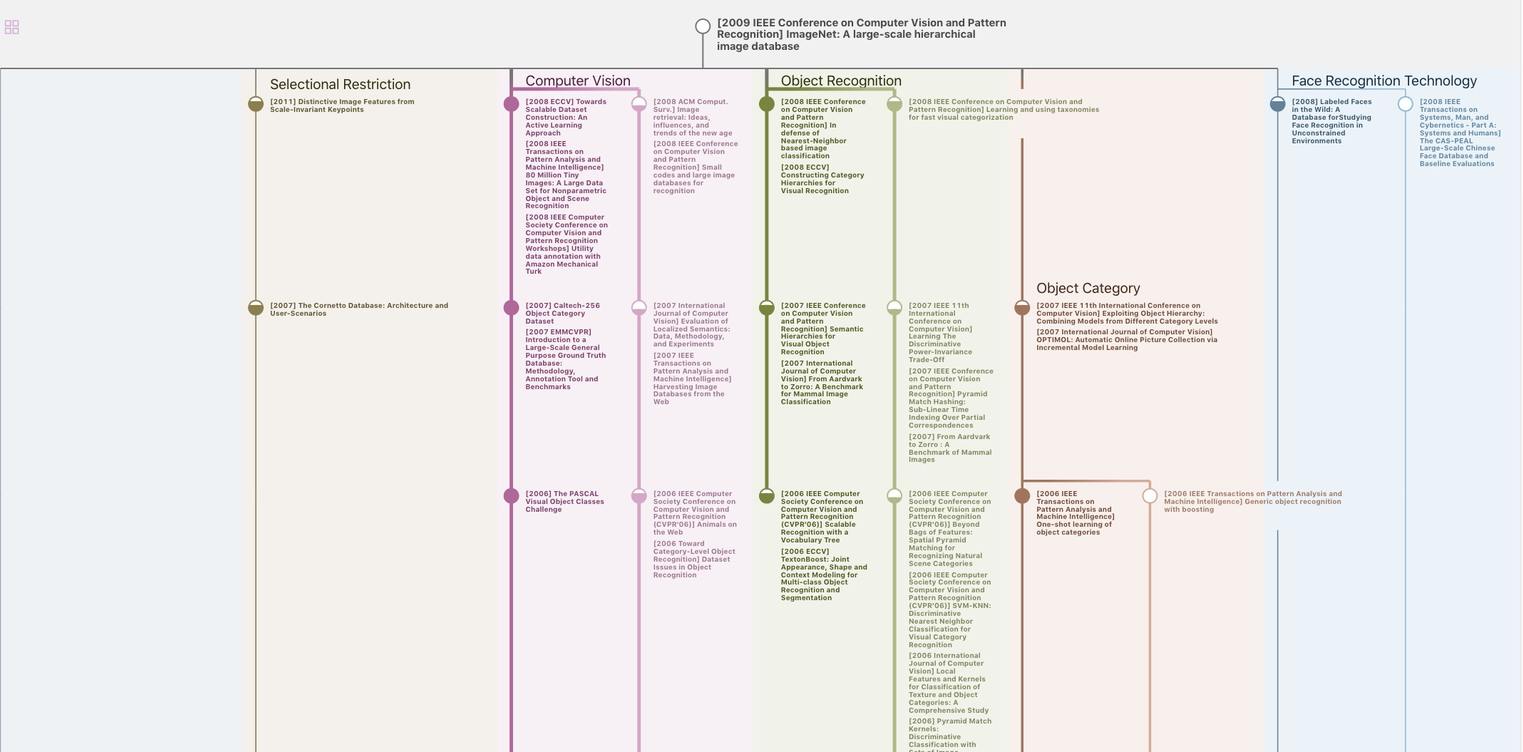
生成溯源树,研究论文发展脉络
Chat Paper
正在生成论文摘要