Efficient DNN Backdoor Detection Guided by Static Weight Analysis.
Inscrypt(2022)
摘要
Despite the great progress of deep neural networks (DNNs), they are vulnerable to backdoor attacks. To detect and provide concrete proof for the existence of backdoors, existing techniques generally adopt the reverse engineering approach. However, most of them suffer from high computational complexity and weak scalability. In this paper, we make a key observation that the weights connected to the backdoor target labels in trojaned DNNs tend to have abnormal distributions, including dissimilarity to other labels and anomalously large magnitude. Based on this observation, we propose an efficient and scalable backdoor detection framework guided by static weight analysis. Our approach first detects the outlier existing in weight distributions and identifies suspicious backdoor target/victim label pairs. Then we conduct reverse engineering to recover the triggers, including a newly designed reverse engineering approach for global transformation attacks and one existing approach for local patch attacks. Finally, we analyze the characteristics of the recovered triggers to suppress false positives. Experimental results show that our approach has state-of-the-art performance on MNIST, CIFAR-10, ImageNet, and TrojAI. In particular, it outperforms NC, ABS, and K-Arm by 31%, 8.7%, and 5% on the public detection benchmark TrojAI in terms of detection accuracy while maintaining the highest efficiency.
更多查看译文
关键词
Deep neural network, Backdoor detection, Static weight analysis, Reverse engineering
AI 理解论文
溯源树
样例
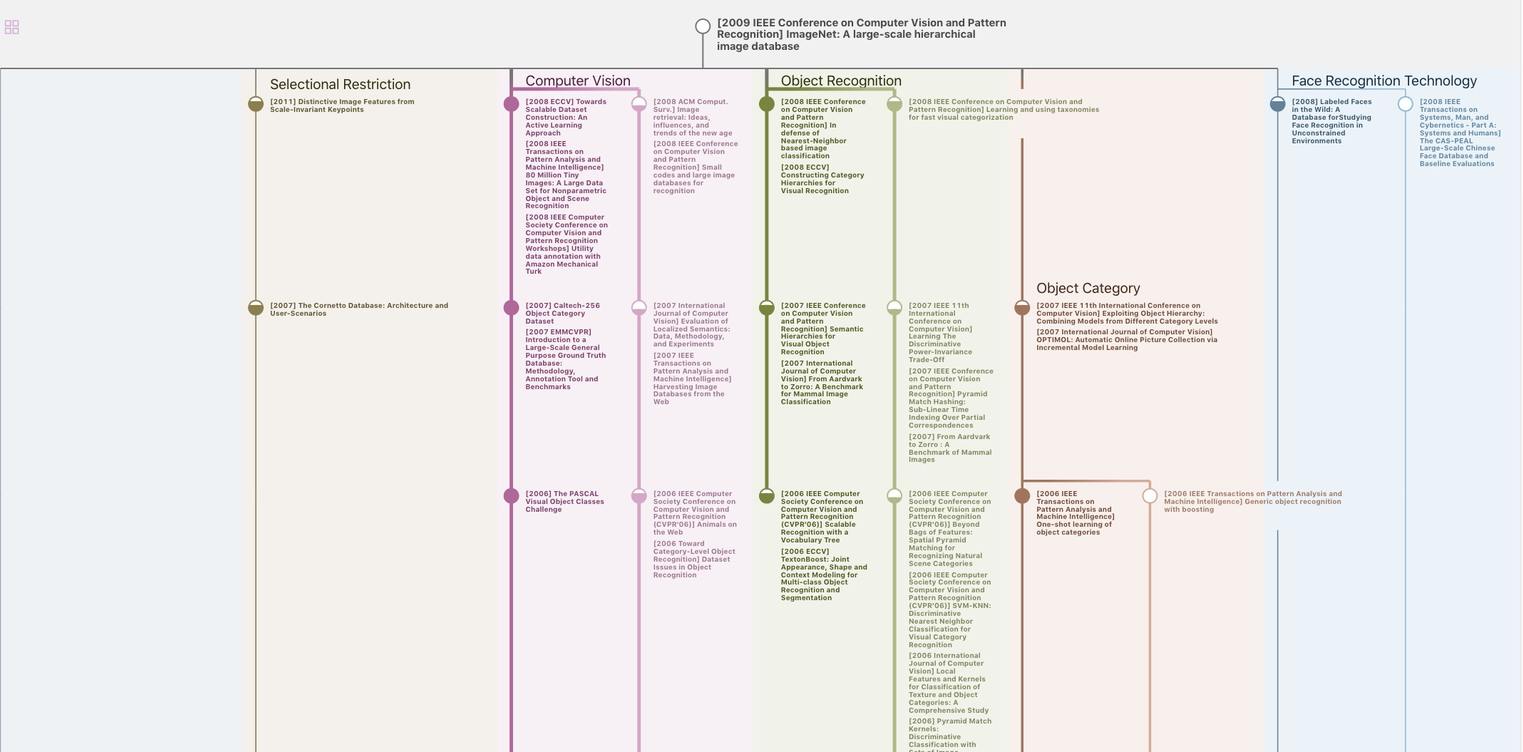
生成溯源树,研究论文发展脉络
Chat Paper
正在生成论文摘要