CycleGAN based Unsupervised Domain Adaptation for Machine Fault Diagnosis
SenSys(2022)
摘要
Fault diagnosis plays a vital role in ensuring the normal operation of the machine and safe production. In recent years, data-driven techniques have gained a lot of popularity for machine fault diagnosis. But most of these techniques assume the training and test data have the same distribution. However, in most practical application scenarios, domain discrepancy can be observed between the training (source) and test (target) data due to different factors like changes in the operating conditions, different sensor locations, etc. Classical approaches fail to address such domain discrepancy, which leads to poor performance. The problem becomes more challenging when the target is completely unlabeled. To address this scenario, domain adaptation techniques are used to transfer the knowledge learned from the labeled source domain to the unlabeled target domain. Recently, adversarial network based domain adaptation has been extensively explored for fault diagnosis. But the adversarial loss alone does not guarantee the translation of the source to the desired target domain (class consistent). Here, we propose to use cycle-consistency loss employing 1D-CycleGAN for learning the source to target mapping for unsupervised adaptation for bearing fault diagnosis. The proposed method is evaluated for two different scenarios, with the source and target from (i) same machine but different working conditions and (ii) different but related machines. Experimental results show that while the proposed method performs comparable to the best-performing benchmark for the first case, it significantly outperforms all the state-of-the-art methods for the challenging second case.
更多查看译文
关键词
Domain adaptation, Machine fault diagnosis, CycleGAN, Unsupervised learning
AI 理解论文
溯源树
样例
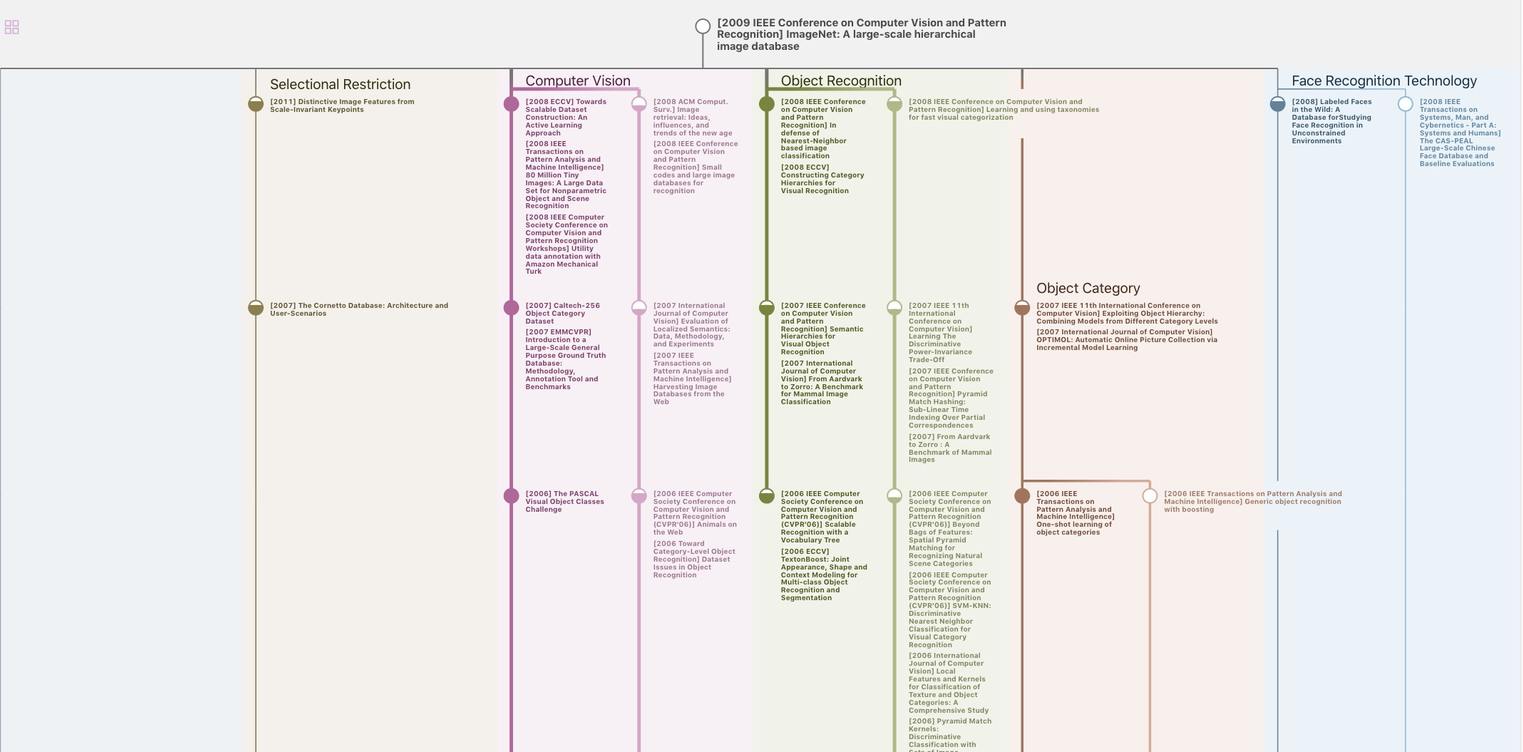
生成溯源树,研究论文发展脉络
Chat Paper
正在生成论文摘要