Model Compression for Deep Reinforcement Learning Through Mutual Information.
IBERAMIA(2022)
摘要
One of the most important limitation of deep learning and deep reinforcement learning, is the number of parameters in their models (dozens to hundreds of millions). Different model compression techniques, such as policy distillation, have been proposed to alleviate this limitation. However, they need a high number of instances to obtain acceptable performance and the use of the source model. In this work, we propose a model compression method based on the comparison of mutual information between the distribution layers of the network. This method automatically determines how much the model should be reduced, and the number of instances required to obtain acceptable performance is considerably lower than the state-of-the-art solutions (19M). It also requires lower resources because only the last two layers of the network are fine-tuned.
更多查看译文
关键词
Model compression, Deep reinforcement learning, Mutual information
AI 理解论文
溯源树
样例
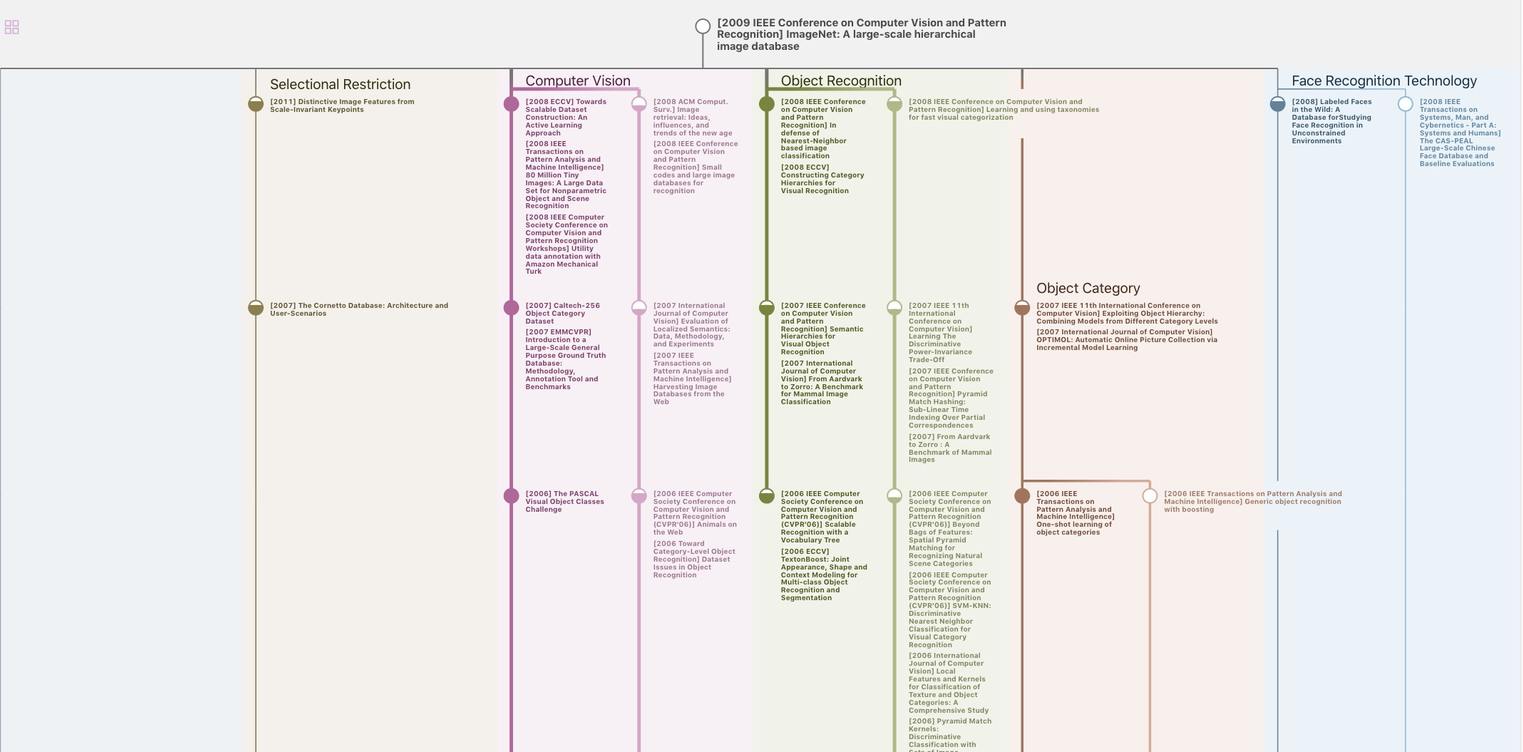
生成溯源树,研究论文发展脉络
Chat Paper
正在生成论文摘要