Speech Emotion Recognition with Complementary Acoustic Representations.
SLT(2022)
摘要
Since CNNs promote local features and Transformers capture long-range dependencies, we explore both models as encoders for acoustic representations in a parallel framework for speech emotion recognition. We choose logMels as input to the CNN encoder and MFCCs to the Transformer encoder. The complementary acoustic representations generated by the two encoders are then fused to predict the frequency distribution of emotions. To further improve the performance, we conduct data augmentation based on vocal tract length perturbation and pretrain the Transformer encoder. The proposed framework is evaluated under the speaker-independent (SI) setting on the improvisation part of the Interactive Emotional Dyadic Motion Capture (IEMOCAP) dataset. Our weighted and unweighted accuracies reached 81.6% and 79.8%, respectively. To the best of our knowledge, this is the state-ofthe-art result reported so far on this dataset in the SI scenario.
更多查看译文
关键词
speech emotion recognition,complementary acoustic representations,convolutional neural network,Transformer,embedding fusion
AI 理解论文
溯源树
样例
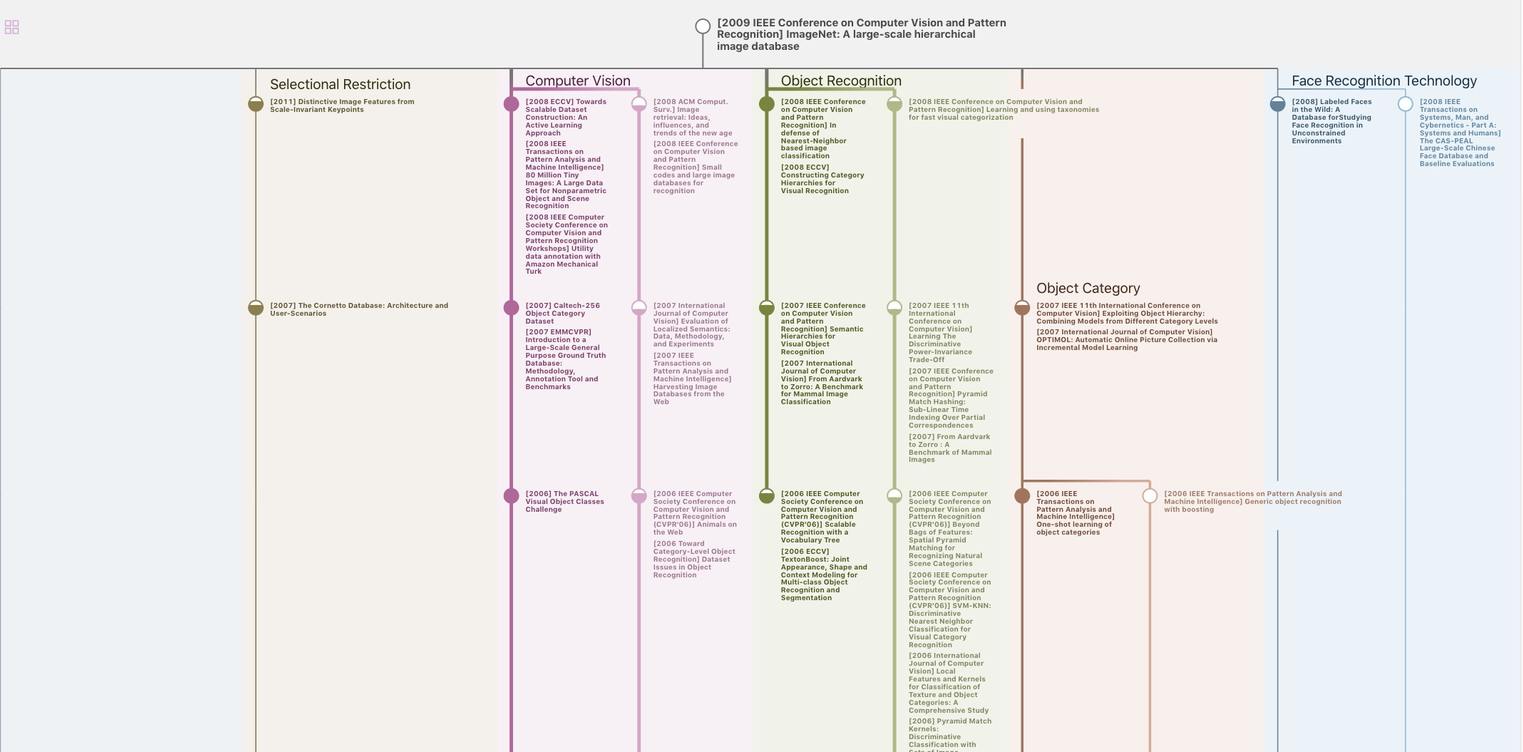
生成溯源树,研究论文发展脉络
Chat Paper
正在生成论文摘要