Exploration of Language-Specific Self-Attention Parameters for Multilingual End-to-End Speech Recognition.
SLT(2022)
摘要
In the last several years, end-to-end (E2E) ASR models have mostly surpassed the performance of hybrid ASR models. E2E is particularly well suited to multilingual approaches because it doesn't require language-specific phone alignments for training. Recent work has improved multilingual E2E modeling over naive data pooling on up to several dozen languages by using both language-specific and language-universal model parameters, as well as providing information about the language being presented to the network. Complementary to previous work we analyze language-specific parameters in the attention mechanism of Conformer-based encoder models. We show that using language-specific parameters in the attention mechanism can improve performance across six languages by up to 12% compared to standard multilingual baselines and up to 36% compared to monolingual baselines, without requiring any additional parameters during monolingual inference nor fine-tuning.
更多查看译文
关键词
Multilingual,CTC,self-attention
AI 理解论文
溯源树
样例
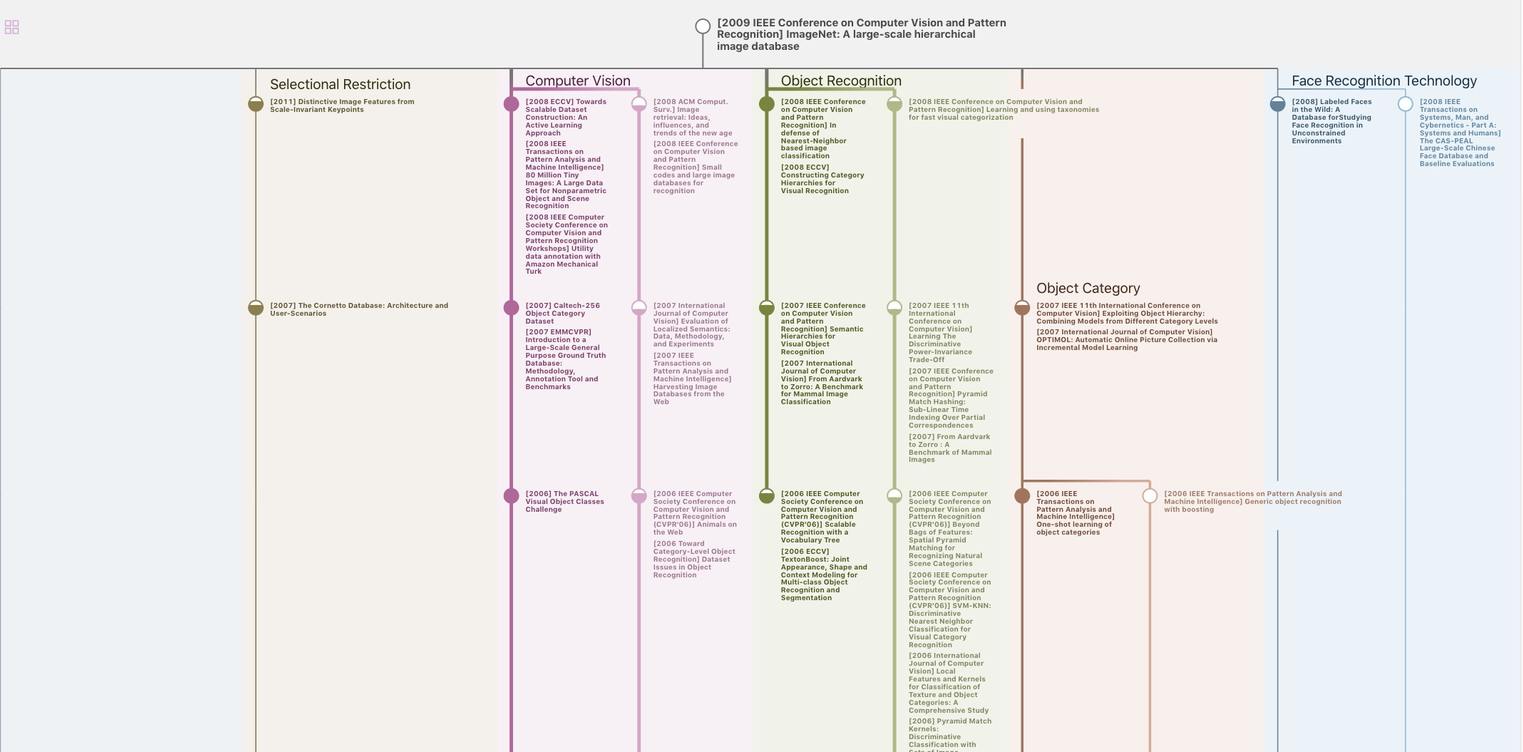
生成溯源树,研究论文发展脉络
Chat Paper
正在生成论文摘要