Self-guided Contrastive Learning for Sequential Recommendation.
APWeb/WAIM (3)(2022)
摘要
Sequential recommendation has injected plenty of vitality into online marketing and retail industry. Existing contrastive learning-based models usually resolve data sparsity issue of sequential recommendation with data augmentations. However, the semantic structure of sequences is typically corrupted by data augmentations, resulting in low-quality views. To tackle this issue, we propose Self- guided contrastive learning enhanced BERT for sequential recommendation ( Self-BERT ). We devise a self-guided mechanism to conduct contrastive learning under the guidance of BERT encoder itself. We utilize two identically initialized BERT encoders as view generators to pass bi-directional messages. One of the BERT encoders is parameter-fixed, and we use the all Transformer layers’ output as a series of views. We employ these views to guide the training of the other trainable BERT encoder. Moreover, we modify the contrastive learning objective function to accommodate one-to-many positive views constraints. Experiments on four real-world datasets demonstrate the effectiveness and robustness of Self-BERT .
更多查看译文
关键词
sequential recommendation,contrastive learning,self-guided
AI 理解论文
溯源树
样例
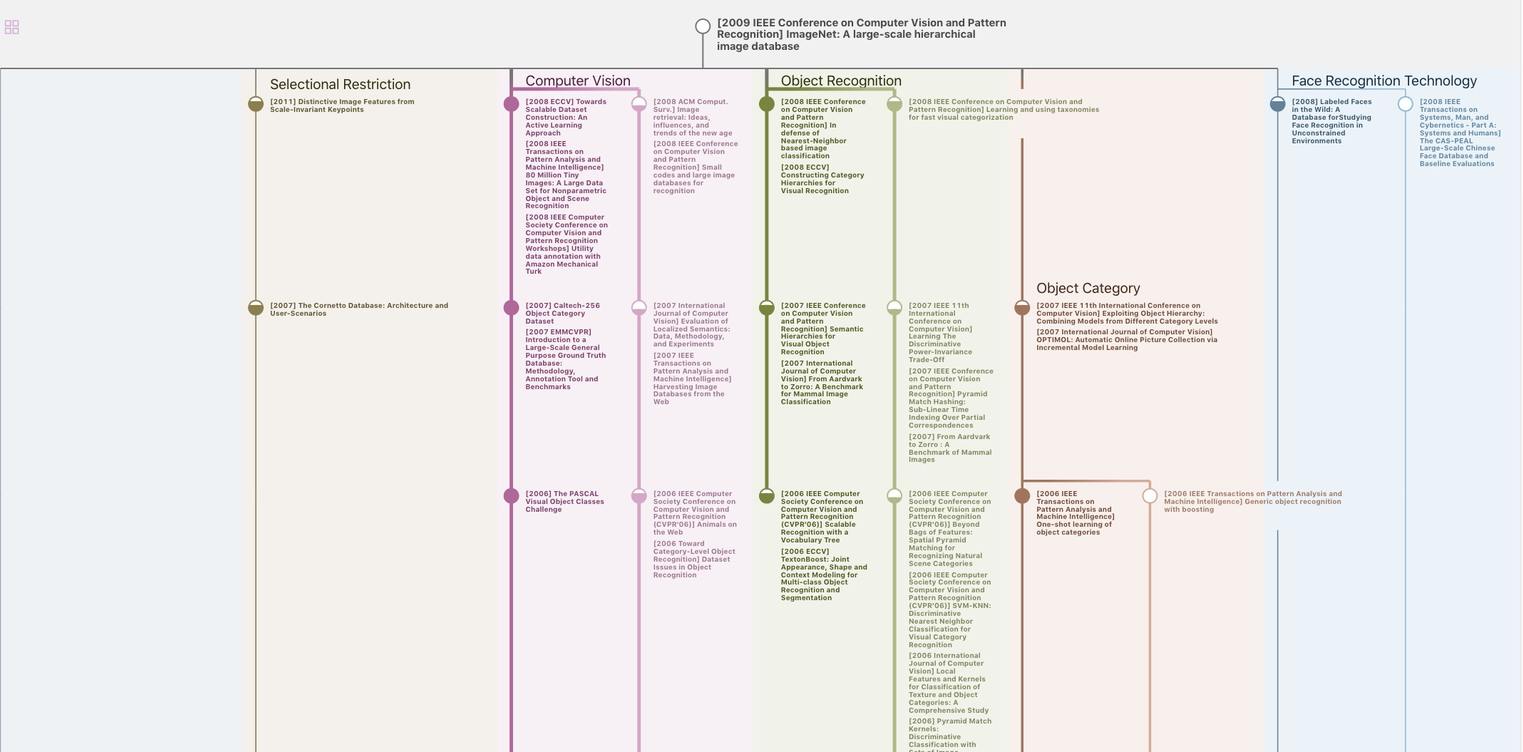
生成溯源树,研究论文发展脉络
Chat Paper
正在生成论文摘要