MORO: A Multi-behavior Graph Contrast Network for Recommendation.
APWeb/WAIM (3)(2022)
摘要
Multi-behavior recommendation models exploit diverse behaviors of users (e.g., page view, add-to-cart, and purchase) and successfully alleviate the data sparsity and cold-start problems faced by classical recommendation methods. In real-world scenarios, the interactive behaviors between users and items are often complex and highly dependent. Existing multi-behavior recommendation models do not fully utilize multi-behavior information in the following two aspects: (1) The diversity of user behavior resulting from the individualization of users’ intents. (2) The loss of user multi-behavior information due to inappropriate information fusion. To fill this gap, we hereby propose a multi-behavior graph contrast network (MORO). Firstly, MORO constructs multiple behavior representations of users from different behavior graphs and aggregate these representations based on behavior intents of each user. Secondly, MORO develops a contrast enhancement module to capture information of high-order heterogeneous paths and reduce information loss. Extensive experiments on three real-world datasets show that MORO outperforms state-of-the-art baselines. Furthermore, the preference analysis implies that MORO can accurately model user multi-behavior preferences.
更多查看译文
关键词
Multi-behavior recommendation, Graph neural network, Multi-task learning, Representation learning
AI 理解论文
溯源树
样例
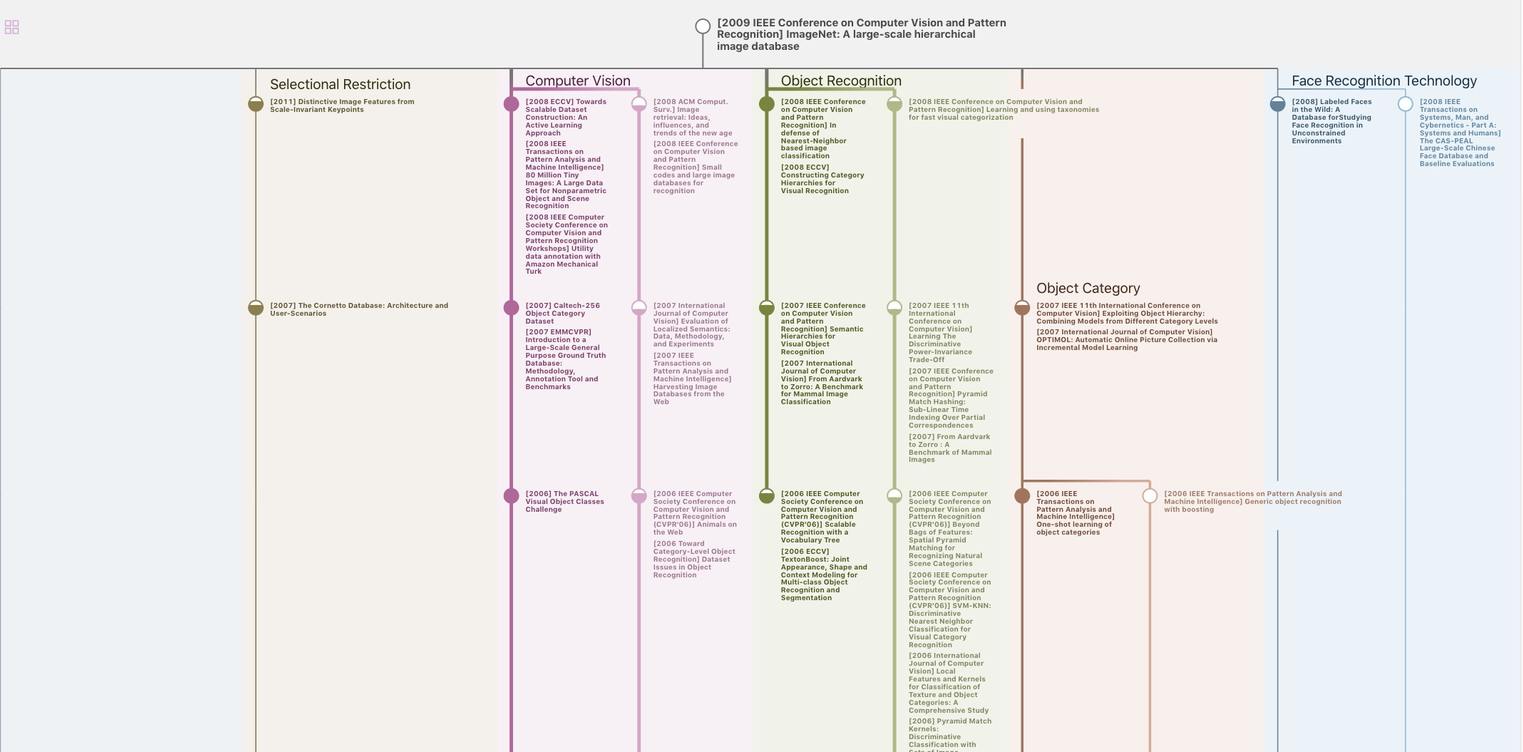
生成溯源树,研究论文发展脉络
Chat Paper
正在生成论文摘要