Straightforward Clustering and Identification of Morphological-Level Pancreatic Carcinoma.
GCCE(2022)
Abstract
Invisibility is the main challenge of diagnosis that there are usually no symptoms of Pancreatic carcinoma (Pc) at the early stage. Therefore, the early and accurate identification of Pc is crucial which is expected to capture the imperceptible region of interest of pathological images (PIs) to support diagnosis and further treatment. This paper presents an attempt at a straightforward clustering autoencoder framework for Pc identification alternative. With merging the Marginal Entropy and Conditional Entropy into the framework, the proposed method shows promise of clustering (optimal cluster is 20) experimenting with an authoritative PIs dataset of Pc. Meanwhile, we design a tool used for tracking the attention of clustering decisions, to provide a certain interpretability for the clinical diagnosis. The proposed method is a plain but potential framework that can be used in further development of a complex deep architecture for more accurate cancer-related research.
MoreTranslated text
Key words
accurate cancer related research,authoritative PIs dataset,clinical diagnosis,clustering decisions,conditional entropy,invisibility,marginal entropy,morphological level pancreatic carcinoma,optimal cluster,pathological images,Pc identification alternative,straightforward clustering autoencoder framework
AI Read Science
Must-Reading Tree
Example
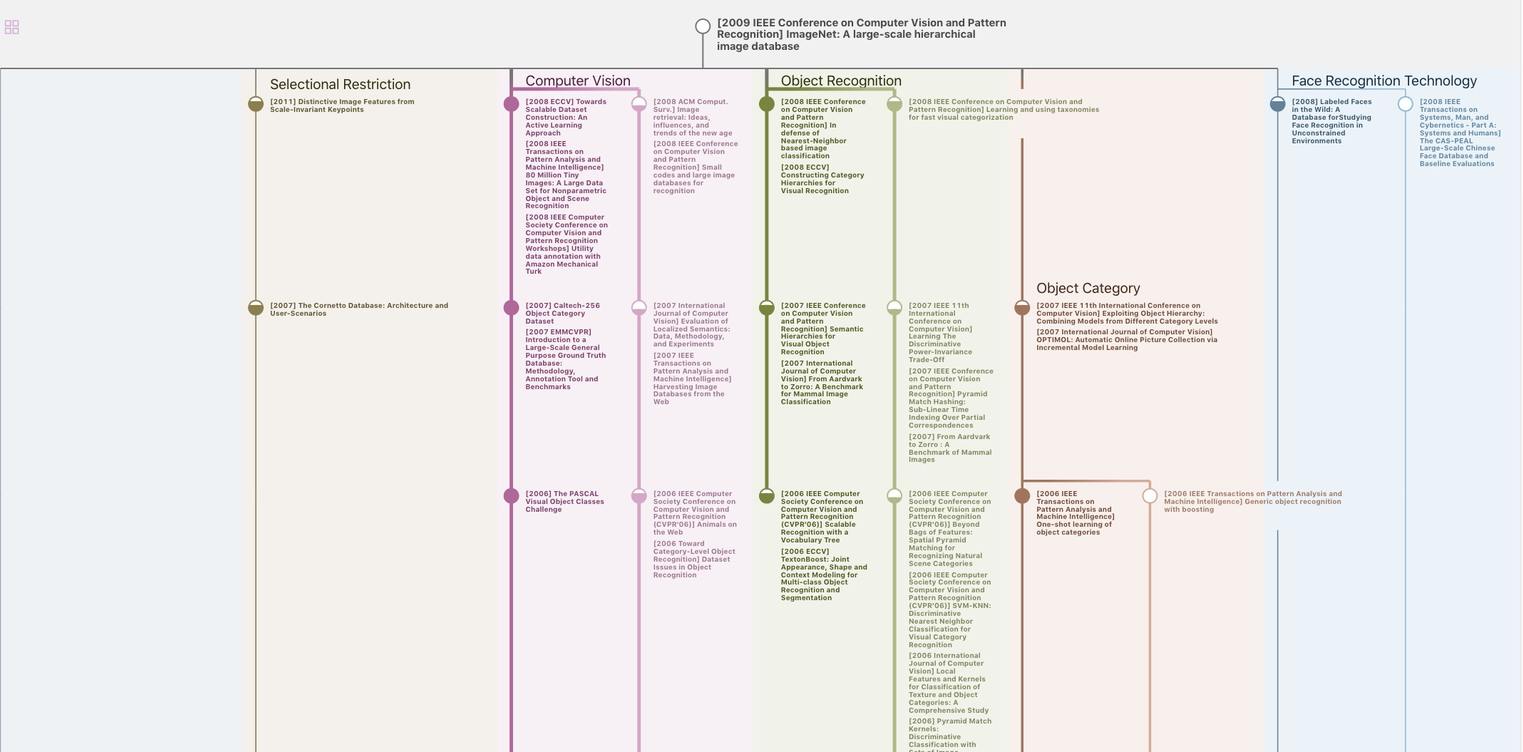
Generate MRT to find the research sequence of this paper
Chat Paper
Summary is being generated by the instructions you defined