Improving News Recommendation with Channel-Wise Dynamic Representations and Contrastive User Modeling.
WSDM(2023)
摘要
News modeling and user modeling are the two core tasks of news recommendation. Accurate user representation and news representation can enable the recommendation system to provide users with precise recommendation services. Most existing methods use deep learning models such as CNN and Self-Attention to extract text features from news titles and abstracts to generate specific news vectors. However, the CNN-based methods have fixed parameters and cannot extract specific features for different input words, while the Self-Attention-based methods have high computational costs and are difficult to capture local features effectively. In our proposed method, we build a category-based dynamic component to generate suitable parameters for different inputs and extract local features from multiple perspectives. Meanwhile, users will mistakenly click on some news terms they are not interested in, so there will be some interaction noises in the datasets. In order to explore the critical user behaviors in user data and reduce the impact of noise data on user modeling, we adopt a frequency-aware contrastive learning method in user modeling. Experiments on real-world datasets verify the effectiveness of our proposed method.
更多查看译文
AI 理解论文
溯源树
样例
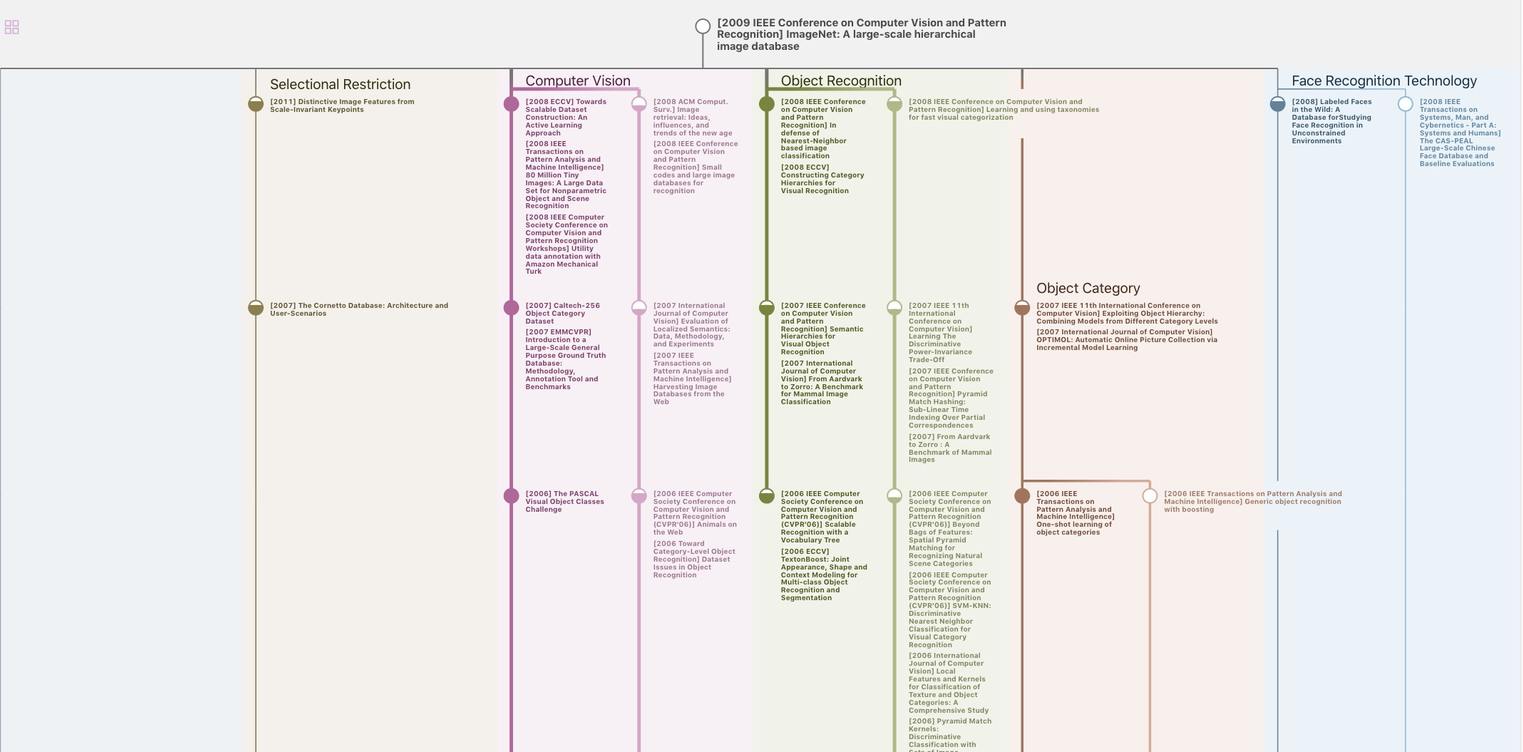
生成溯源树,研究论文发展脉络
Chat Paper
正在生成论文摘要