Iterative Learning and Model Predictive Control for Repetitive Nonlinear Systems via Koopman Operator Approximation
CDC(2022)
Abstract
This paper presents an iterative way of computing a control algorithm with the aim of enabling reference tracking for an unknown nonlinear system. The method consists of three blocks: iterative learning control (ILC), robust model predictive control (MPC), and a linear approximation of the Koopman operator. The method proceeds in iterations, where at the end of an iteration, two steps are performed. First, the trajectories of the system obtained from previous iterations are used to build the linear approximation of the Koopman operator. Second, the linear model is used to compute the ILC signal. While these steps are executed in an offline manner, during an iteration, the control actions are computed online using the robust tubebased MPC. The tubes are defined by constraint tightening sets that compensate for the discrepancy between the true dynamics and its linear approximation. We demonstrate our method on the reference tracking for a 4 tank system.
MoreTranslated text
Key words
4 tank system,constraint tightening sets,ILC signal,iteration,iterative learning control,Koopman operator approximation,linear approximation,reference tracking,repetitive nonlinear systems,robust model predictive control,robust tube-based MPC,unknown nonlinear system
AI Read Science
Must-Reading Tree
Example
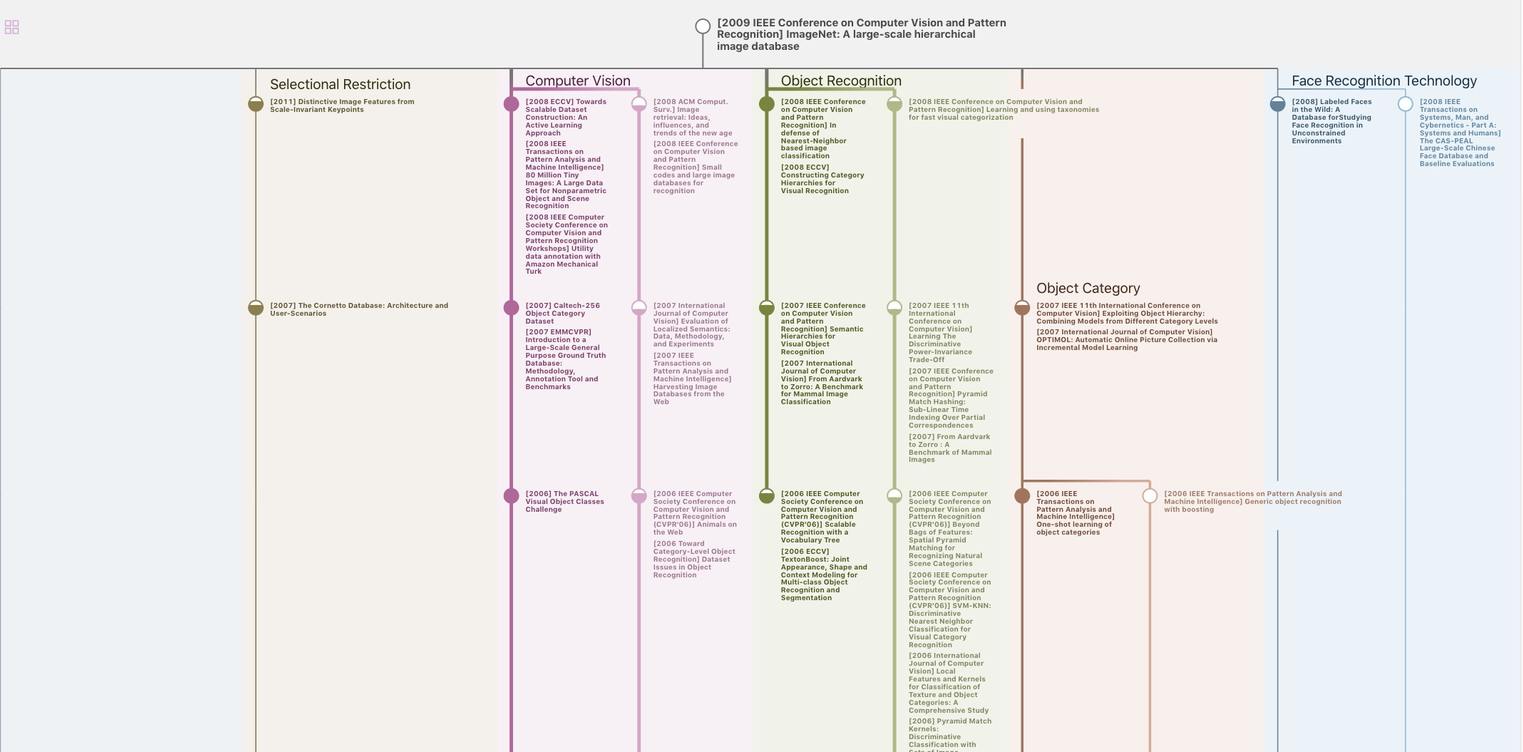
Generate MRT to find the research sequence of this paper
Chat Paper
Summary is being generated by the instructions you defined