Surrogate Modelling of Dynamic Phasor Simulations of Electrical Drives.
IECON(2022)
摘要
This work develops and benchmarks surrogate models for Dynamic Phasor (DP) simulation of electrical drives. DP simulations of complex systems may be time-consuming due to the increased number of equations. Thus, it is desirable to have a data-driven approach to compute the critical state/control variables and power losses. The surrogate models are intended to be used as a steady-state equivalent of the DP simulation model. We consider the Gaussian Process (GP), Multi Layer Perceptron, and Random Forest as surrogate models. Among other techniques, GPs are found to have good accuracy. Moreover, GPs are data-efficient and have desirable properties, such as built-in uncertainty quantification. The study shows that the GP performs better compared to other techniques in terms of the Mean Squared Error of the prediction, while still being very fast to evaluate. We illustrate the potential of these surrogate models to also predict transient behavior.
更多查看译文
关键词
dynamic phasor simulations,electrical drives,surrogate modelling
AI 理解论文
溯源树
样例
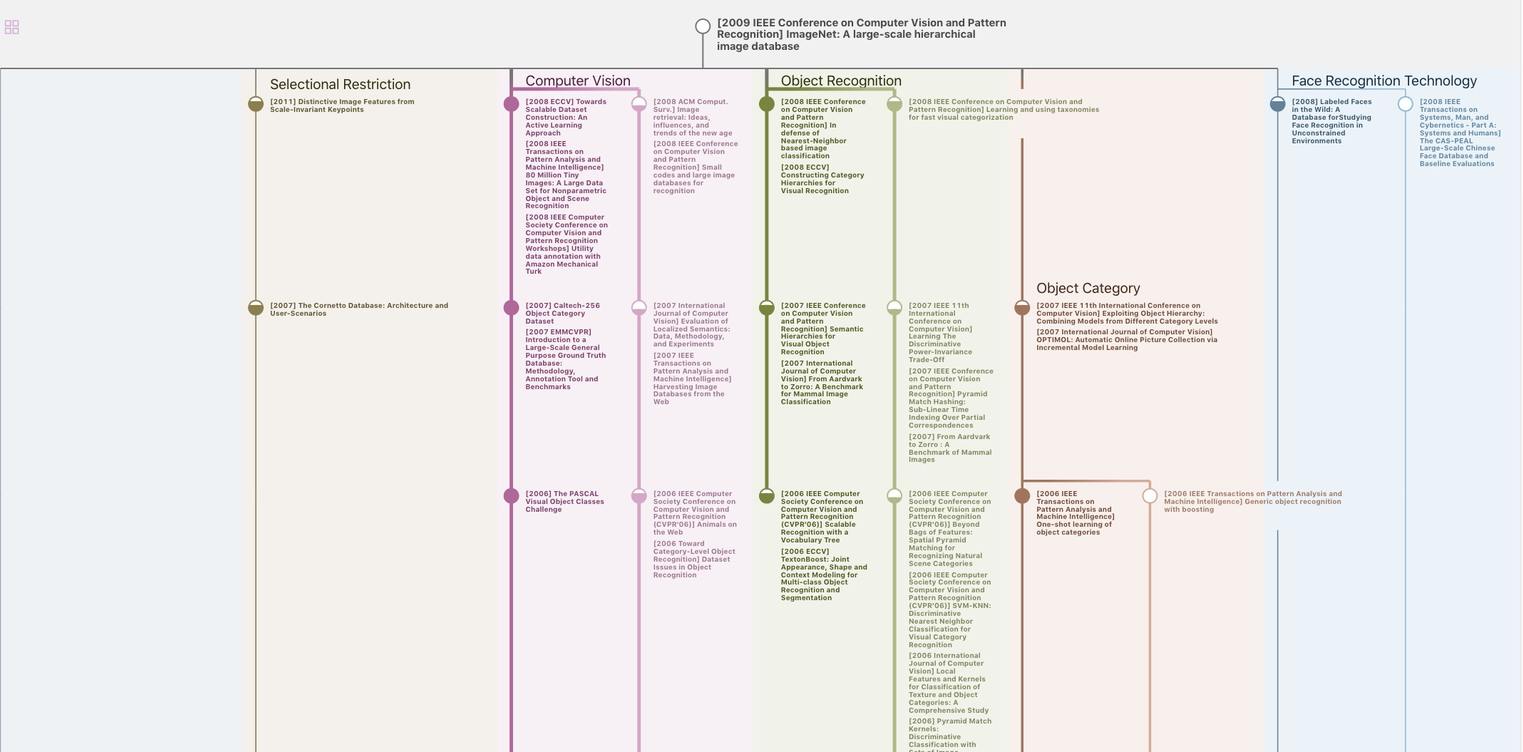
生成溯源树,研究论文发展脉络
Chat Paper
正在生成论文摘要