A Pedestrian Detection Method Based on Improved ResNet for Container Terminal.
HCC(2021)
Abstract
Pedestrian detection in the scene of automated container terminal can bring massive benefits to improving operation safety of the terminal. However, pedestrian detection in container terminal environment exists small pedestrian target size, extreme foregroung-background class imbalance problems. To solve these problems, this paper proposes a one-stage pedestrian detection model based on the improved ResNet. For small target pedestrian detection, we introduce the Channel attention module and spatial attention module to ResNet, enhancing the network's attention to small target pedestrians. Moreover, the feature pyramid network is added to achieve semantic fusion of different scales to enhance the detection of small-scale targets. Finally, the focal loss function is employed as the loss function to suppress the weight influence of simple samples and relieve the problem of extreme foregroung-background class imbalance. The experimental results on the virtual container terminal pedestrian dataset show that the average accuracy of the model is 74.3%, which is 3.9% higher than that of ResNet. The detection accuracy is also improved by comparing with traditional pedestrian detection models. In addition, the effectiveness of the model is verified on Caltech and COCO2017 datasets.
MoreTranslated text
Key words
pedestrian detection method,improved resnet
AI Read Science
Must-Reading Tree
Example
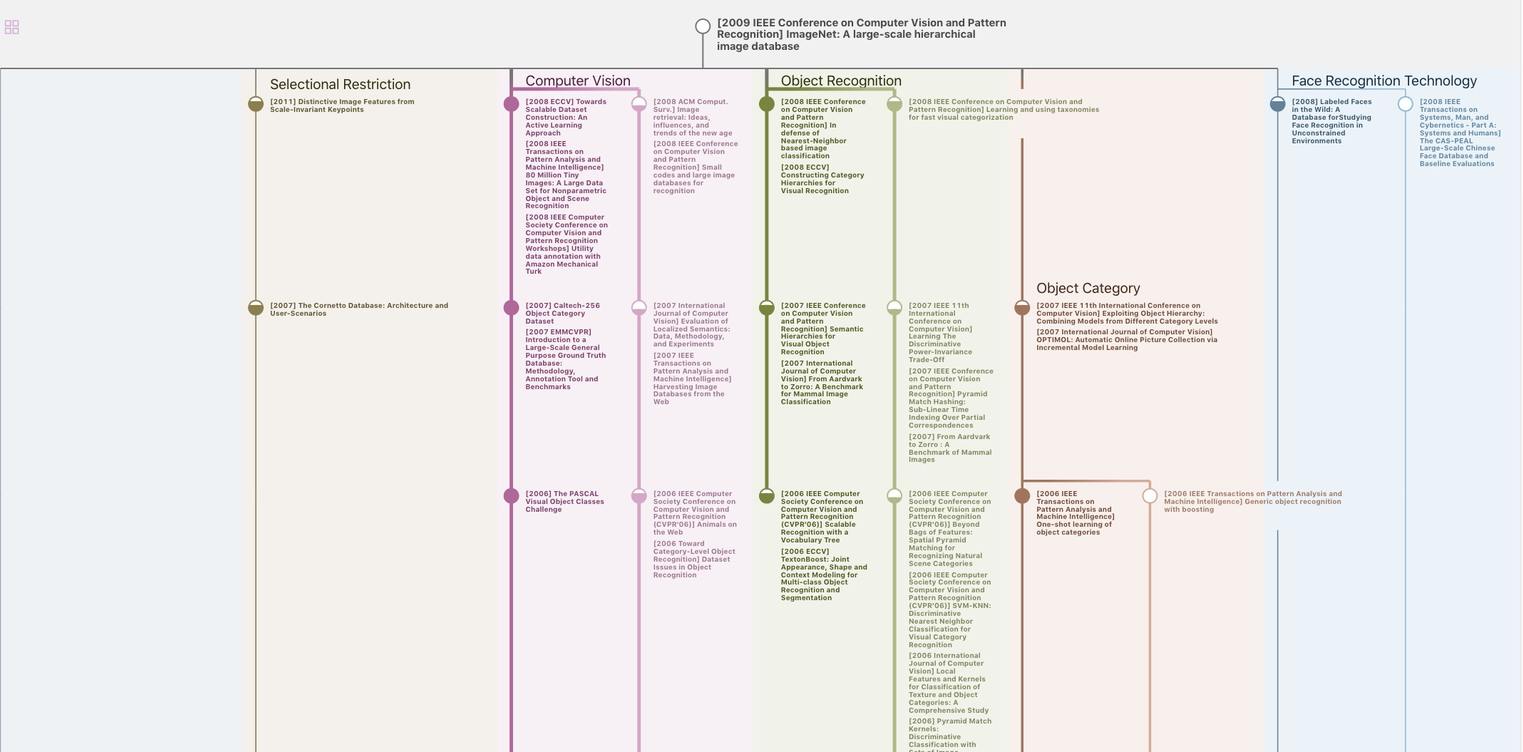
Generate MRT to find the research sequence of this paper
Chat Paper
Summary is being generated by the instructions you defined