Leveraging Efficiency through Hybrid Prioritized Experience Replay in Door Environment.
ROBIO(2022)
摘要
Experience replay enables agents to remember and reuse past experiences in reinforcement learning, just as human beings utilize the past memory. At present, the experience buffer of on-policy algorithm iterates fast and cause the problem of low sample utilization, which leads to the low efficiency of the training agents based on uniform selected samples. Most of the existing rule-based replay strategies have been applied in the off-policy algorithm, which have shown good results. Replay strategy adjustment is challenging, as replay memory samples have large noise levels, which leads to unstable value functions. One of the most challenging aspects is deciding what experience to prioritize. To solve this problem, we propose a method called Proximal Policy Optimization with Hybrid Prioritized Experience Replay (HPER-PPO) to adjust the sample priority and guide the selection, through which the policy can be better optimized and the cumulative reward can be maximized. We select two kinds door- related long horizon tasks to better measure whether the agent has greater ability to learn and obtain cumulative rewards in our method. The results show that our method can reduce the training time and potentially increase long-term return. Further, we propose a possible explanation for the reason why this method improves the efficiency and brings changes to on-policy algorithm experience replay mechanism.
更多查看译文
关键词
Reinforcement Learning,Experience Reply,Proximal Policy Optimization,Sample Efficiency
AI 理解论文
溯源树
样例
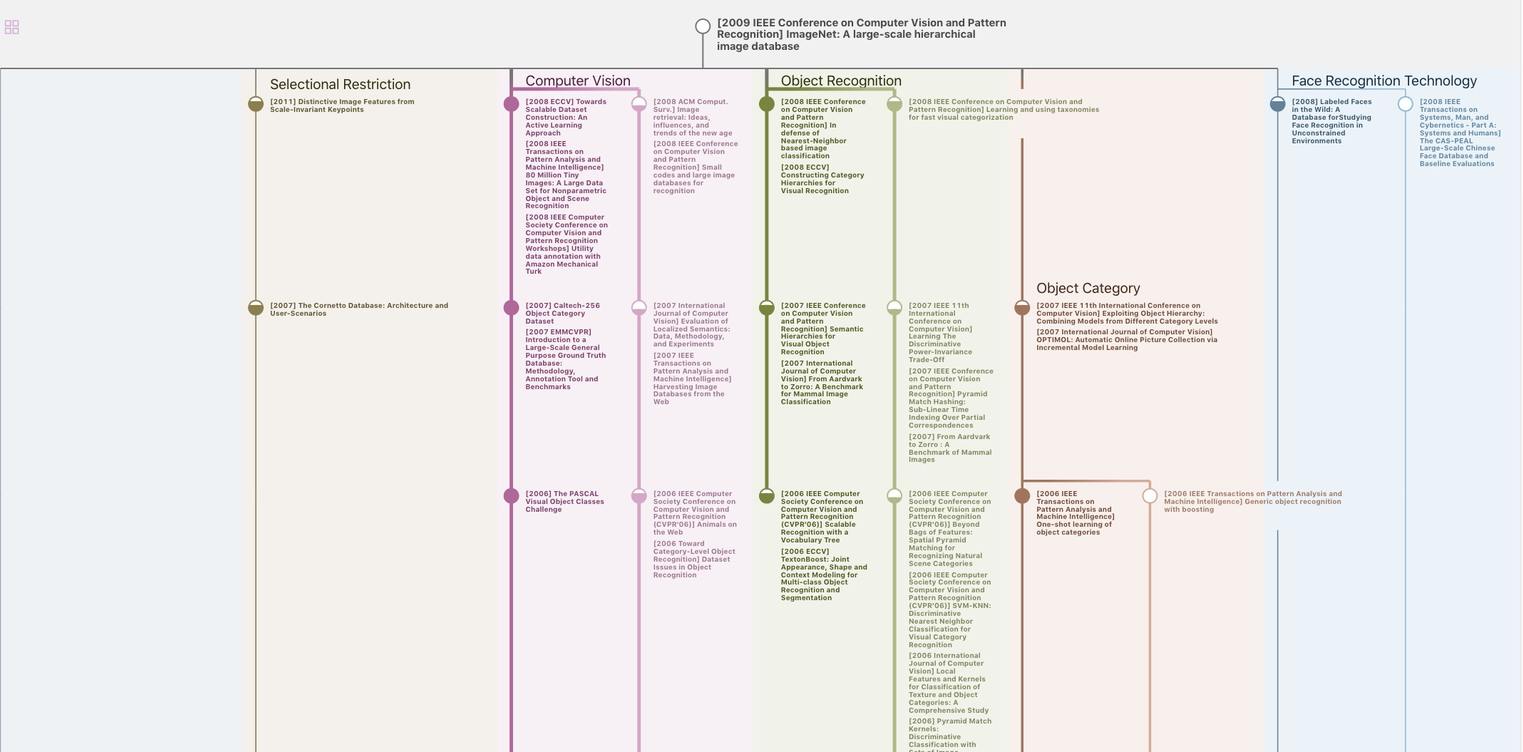
生成溯源树,研究论文发展脉络
Chat Paper
正在生成论文摘要