PAUMER: Patch Pausing Transformer for Semantic Segmentation.
BMVC(2023)
摘要
We study the problem of improving the efficiency of segmentation transformers by using disparate amounts of computation for different parts of the image. Our method, PAUMER, accomplishes this by pausing computation for patches that are deemed to not need any more computation before the final decoder. We use the entropy of predictions computed from intermediate activations as the pausing criterion, and find this aligns well with semantics of the image. Our method has a unique advantage that a single network trained with the proposed strategy can be effortlessly adapted at inference to various run-time requirements by modulating its pausing parameters. On two standard segmentation datasets, Cityscapes and ADE20K, we show that our method operates with about a $50\%$ higher throughput with an mIoU drop of about $0.65\%$ and $4.6\%$ respectively.
更多查看译文
关键词
patch pausing transformer,semantic segmentation
AI 理解论文
溯源树
样例
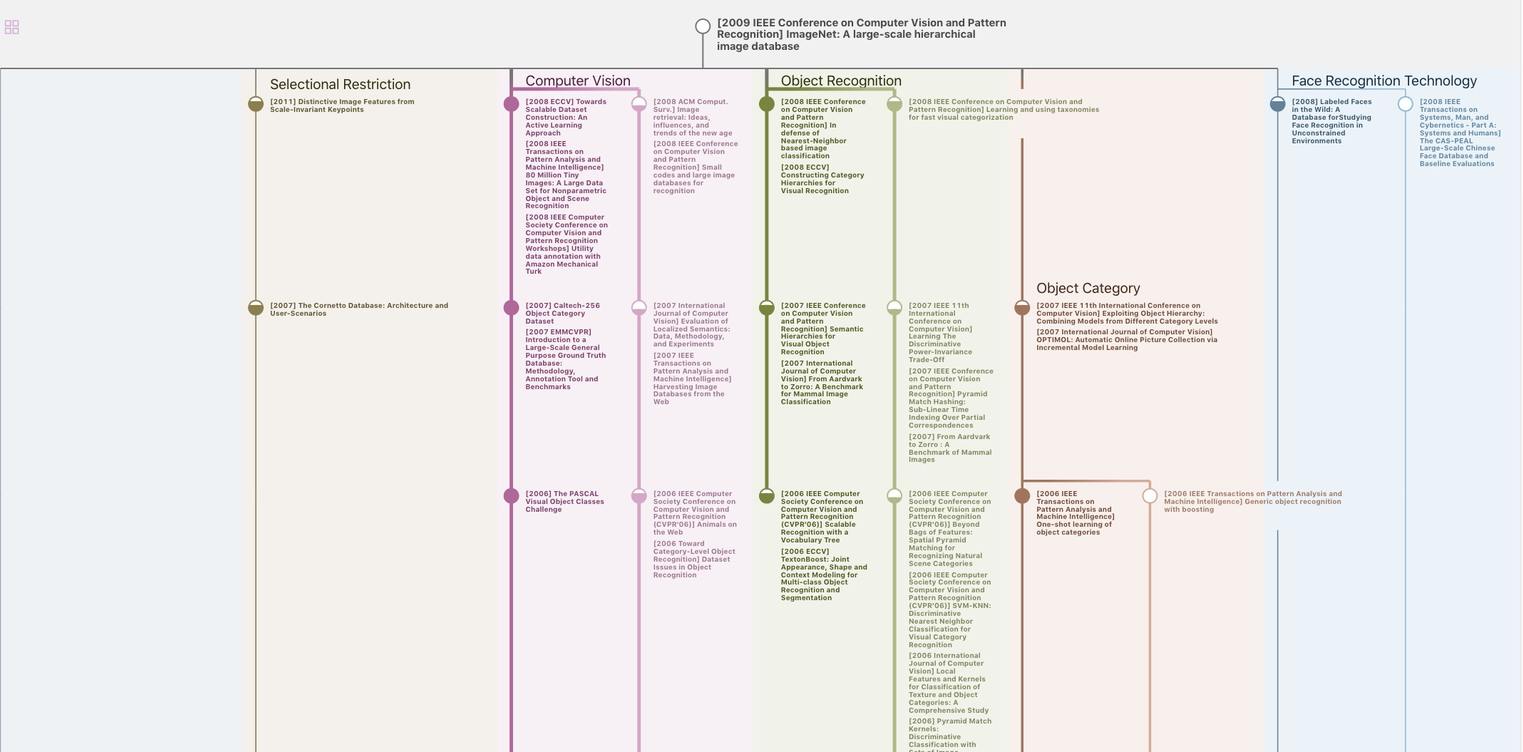
生成溯源树,研究论文发展脉络
Chat Paper
正在生成论文摘要