A Convex Formulation for Graph Convolutional Training: Two Layer Case.
ICDM(2022)
摘要
Graph convolutional networks (GCNs) are popular neural network models for relational graph data and have inspired de facto models for learning on relational datasets with high-dimensional vertex features. GCNs have also been successfully applied for data mining applications. Though GCNs have been studied from the lens of expressivity and generalisability, their theoretical and especially optimisation properties are less well understood. Understanding the theoretical properties is a great challenge because of the highly non-convex training and non-linear structure of GCNs. In this paper, we provide the first steps towards understanding the optimisation properties of GCNs by introducing a convex program that globally solves the training problem for GCNs and other closely related neural models with two layers equipped with rectified linear unit (ReLU) activations.
更多查看译文
关键词
graph convolutional training,convex formulation,layer
AI 理解论文
溯源树
样例
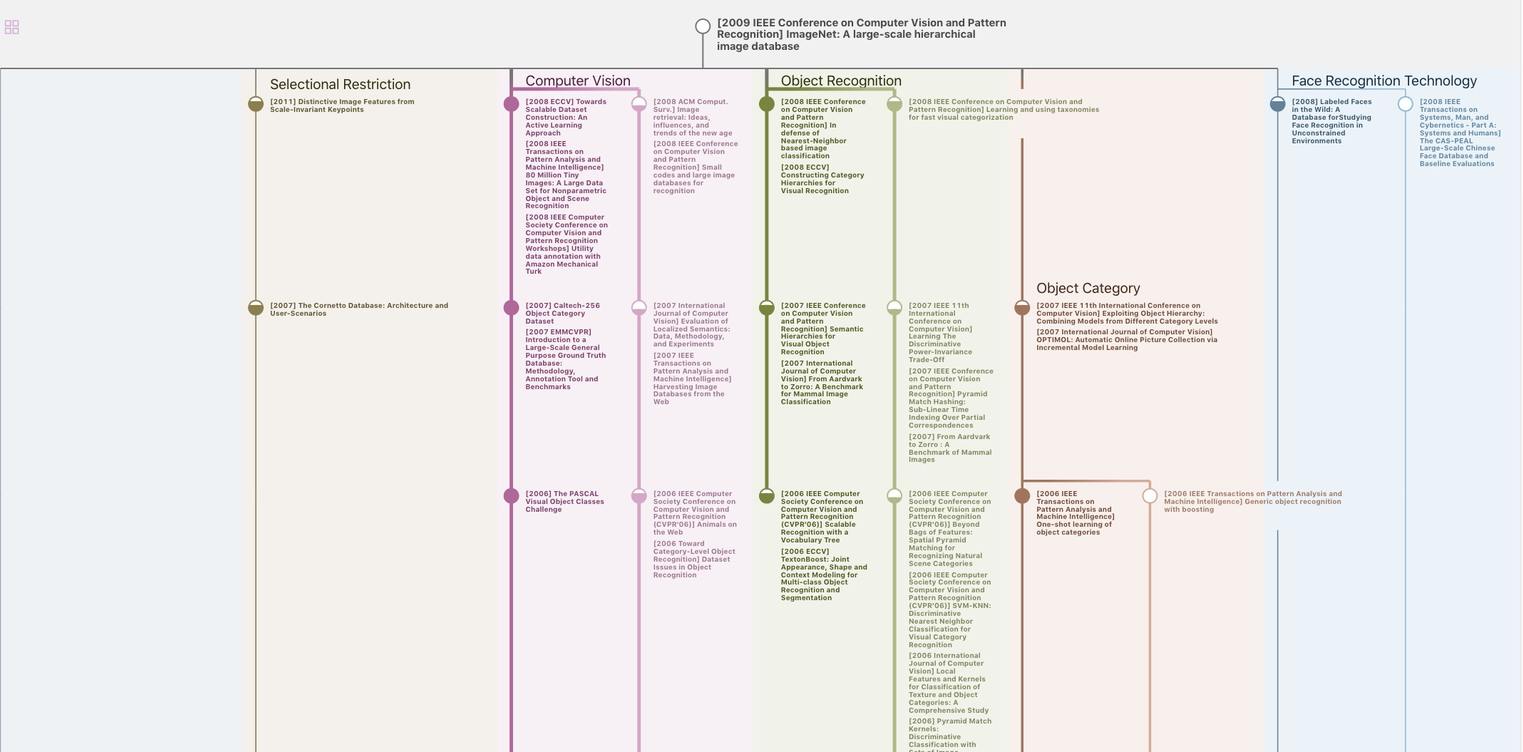
生成溯源树,研究论文发展脉络
Chat Paper
正在生成论文摘要