DREAM: Domain Invariant and Contrastive Representation for Sleep Dynamics.
ICDM(2022)
摘要
Sleep staging is a key challenge in diagnosing and treating sleep-related diseases due to its labor-intensive, timeconsuming, costly, and error-prone. With the availability of largescale sleep signal data, many deep learning methods are proposed for automatic sleep staging. However, these existing methods face several challenges including the heterogeneity of patients' underlying health conditions and the difficulty modeling complex interactions between sleep stages. In this paper, we propose a neural network architecture named DREAM to tackle these issues for automatic sleep staging. DREAM consists of (i) a feature representation network that generates robust representations for sleep signals via the variational auto-encoder framework and contrastive learning and (ii) a sleep stage classification network that explicitly models the interactions between sleep stages in the sequential context at both feature representation and label classification levels via Transformer and conditional random field architectures. Our experimental results indicate that DREAM significantly outperforms existing methods for automatic sleep staging on three sleep signal datasets.
更多查看译文
关键词
deep learning,domain invariant,contrastive learning,sleep staging,sleep dynamics,EEG analysis
AI 理解论文
溯源树
样例
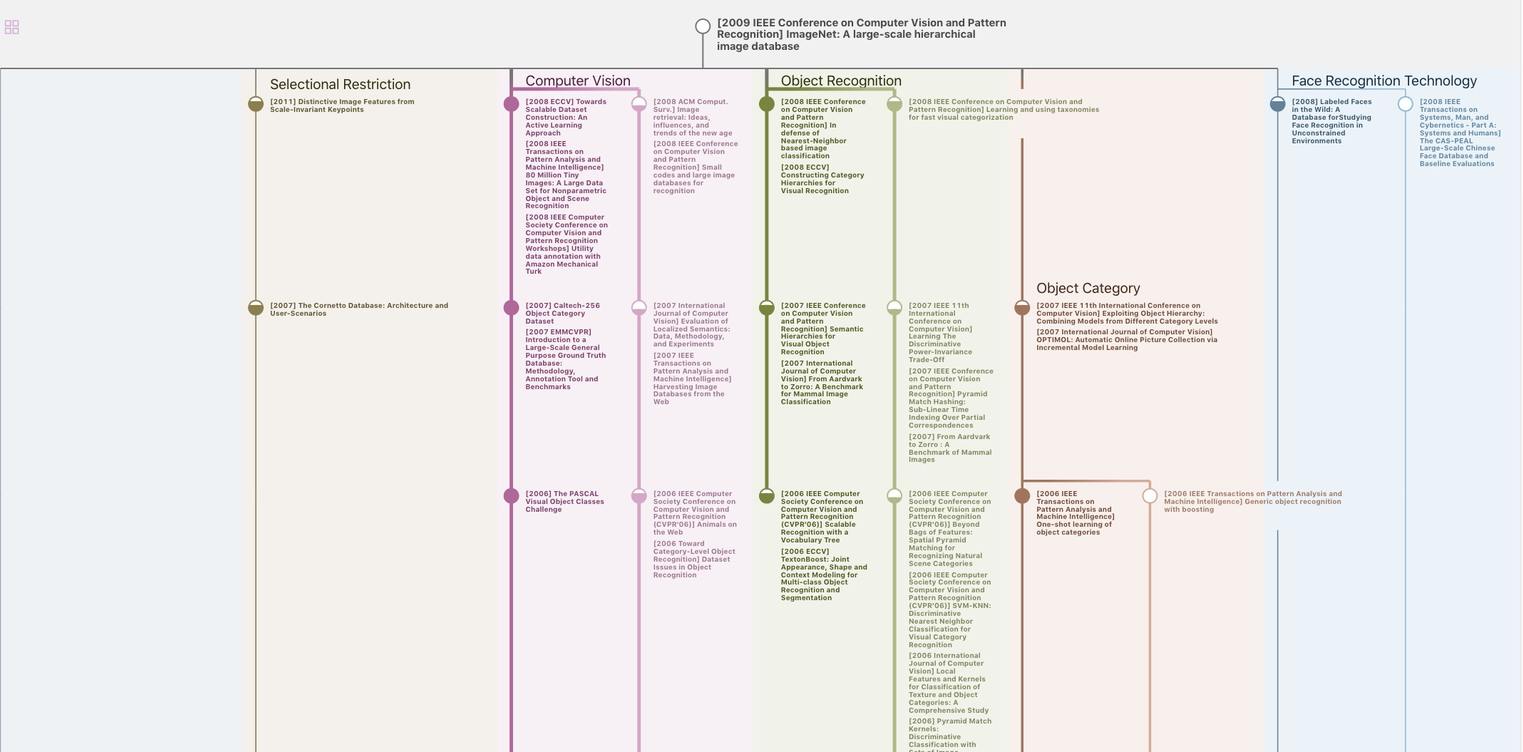
生成溯源树,研究论文发展脉络
Chat Paper
正在生成论文摘要