Mean-Field Multi-Agent Reinforcement Learning for Adaptive Anti-Jamming Channel Selection in UAV Communications
WCSP(2022)
Abstract
In the large-scale anti-jamming UAV communication network, massive number of UAV users aim to compete for limited spectrum resources while fighting against possible external interference from malicious jammers. Specifically, each UAV-to-UAV (U2U) communication link targets at finding the optimal channel selection that maximizes its long-term expected achievable rate. We formulate the distributed multi-UAV anti-jamming problem as a partially observable stochastic game (POSG), where each UAV only has partial observability of the entire network environment due to the limited sensing capabilities. To deal with the complex interactions among large-scale UAVs, we simplify the POSG problem as a mean-field game, where each U2U link only interacts with the aggregate interference from the neighboring U2U links and the malicious jammers. We propose a soft mean-field Q learning (Soft-MFQ) algorithm to obtain the Nash equilibrium of the U2Us' channel selection policies in a model-free scenario. The simulation results show that the proposed algorithm outperforms other benchmark algorithms in terms of convergence speed and the average reward, especially when the number of UAVs is large.
MoreTranslated text
Key words
UAV,anti-jamming,partially observable stochastic game,mean field,multi-agent reinforcement learning
AI Read Science
Must-Reading Tree
Example
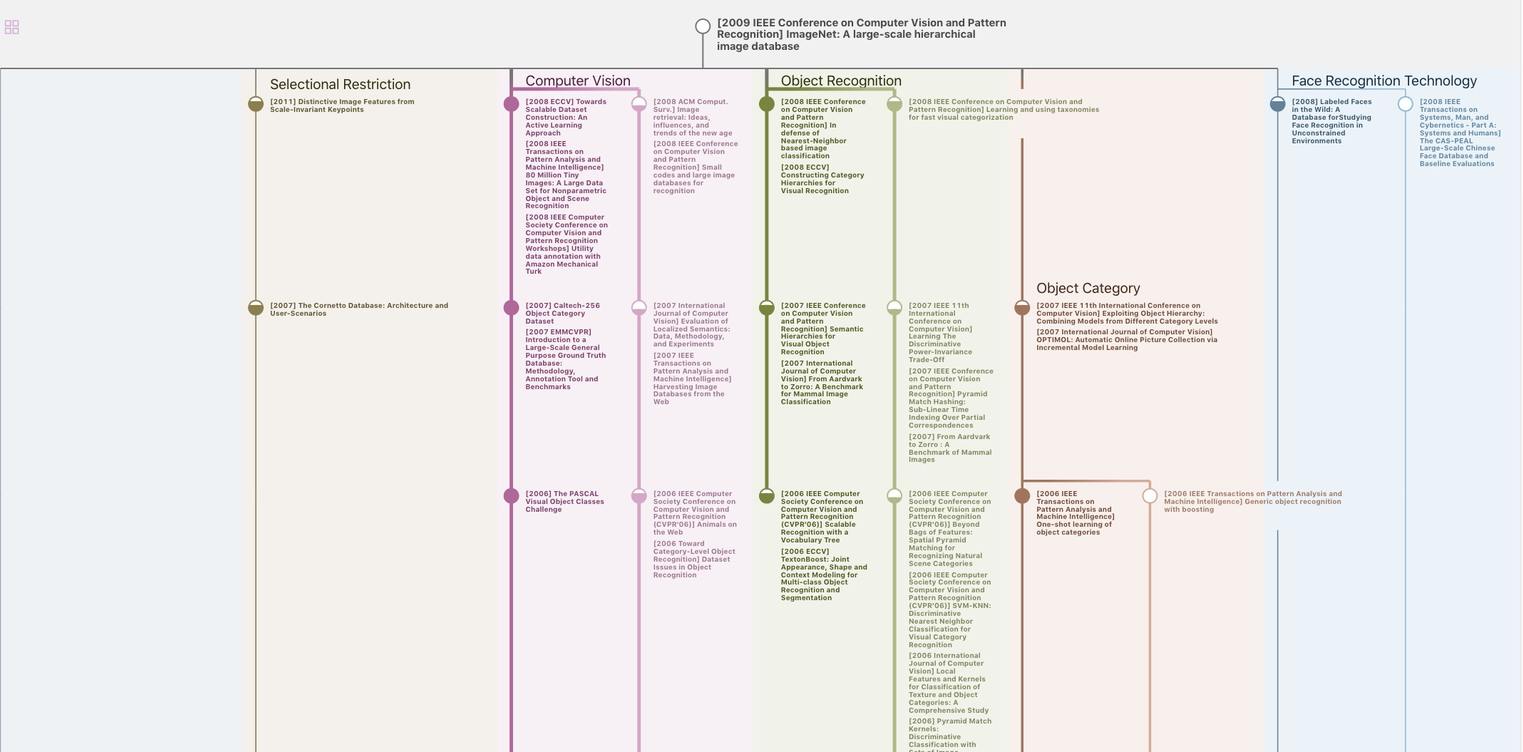
Generate MRT to find the research sequence of this paper
Chat Paper
Summary is being generated by the instructions you defined