Poster: Empirical Evaluation of AutoML Algorithms for Motor Health Prediction.
EWSN(2022)
摘要
The past few years have witnessed a growing interest in edge analytics across different industries. In this new paradigm, data is processed at the edge close to where it is generated. Therefore, in comparison to cloud analytics, edge analytics bring benefits of reduced data transmissions, enhanced data security, and improved responsiveness of infield devices. Due to limited resources available to edge devices, one of the main challenges for edge analytics is to reduce the footprints of machine learning models in terms of timing, memory, and energy. This work evaluates and compares several common AutoML algorithms in optimizing an industrial edge analytics use case for motor health monitoring. We reveal the capabilities of existing algorithms in getting both accurate and small machine learning models. Based on our evaluations, future research directions are outlined.
更多查看译文
AI 理解论文
溯源树
样例
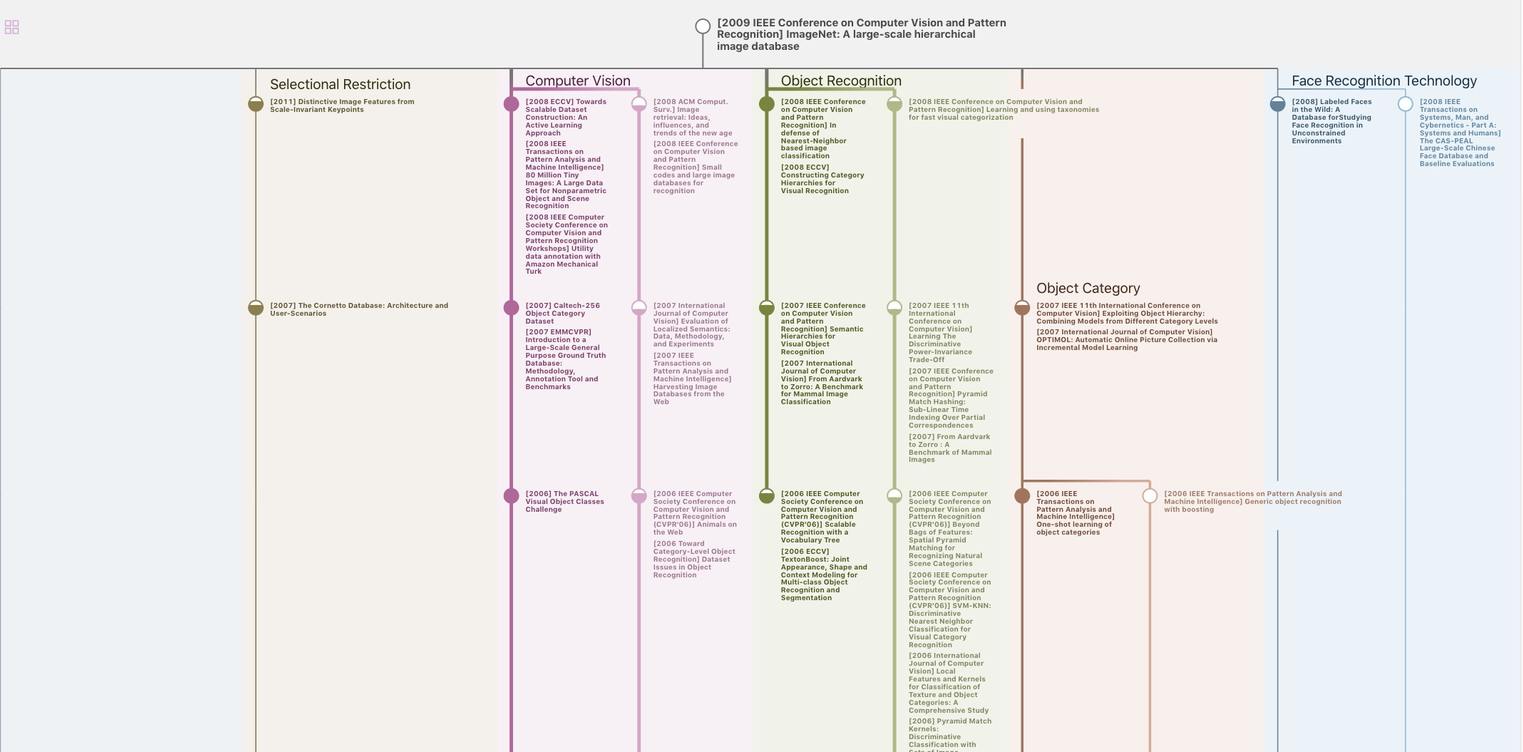
生成溯源树,研究论文发展脉络
Chat Paper
正在生成论文摘要