Modern Trends in Quantum AI: Distributed and High-Definition Computation.
ICOIN(2023)
摘要
As the technology of quantum computers experienced major advancements in recent years, Quantum computing (QC) has also naturally received an unprecedented amount of attention and the number of research on the topic has greatly increased as well. This is because compared to classical computing, QC is able to solve complex problems quickly and possess impressive computational capabilities. One of the fields which can exploit the strengths of QC is quantum machine learning (QML). QML integrates the field of QC and machine learning by applying QC to various areas of machine learning e.g., deep learning, reinforcement learning, and distributed learning. Despite the noise intermediate scale quantum (NISQ) limitation of QC, QML models still show superior performance compared to their classical counterparts by leveraging the strengths of QC. This has been thoroughly corroborated via extensive simulation results in many works which demonstrate the full potential and importance of QML. Therefore, in this paper, we aim to explore various QML methodologies e.g., quantum deep learning, quantum deep reinforcement learning, and quantum distributed learning. Furthermore, the model structures of each methodology will be discussed, highlighting the possibilities and limitations of each model. Finally, the direction of future works of QML research will be discussed as well.
更多查看译文
关键词
classical computing,high-definition computation,impressive computational capabilities,machine learning,noise intermediate scale quantum limitation,QC,QML methodologies e.g,QML models,QML research,Quantum AI,quantum computers,Quantum computing,quantum deep learning,quantum deep reinforcement learning,quantum distributed learning
AI 理解论文
溯源树
样例
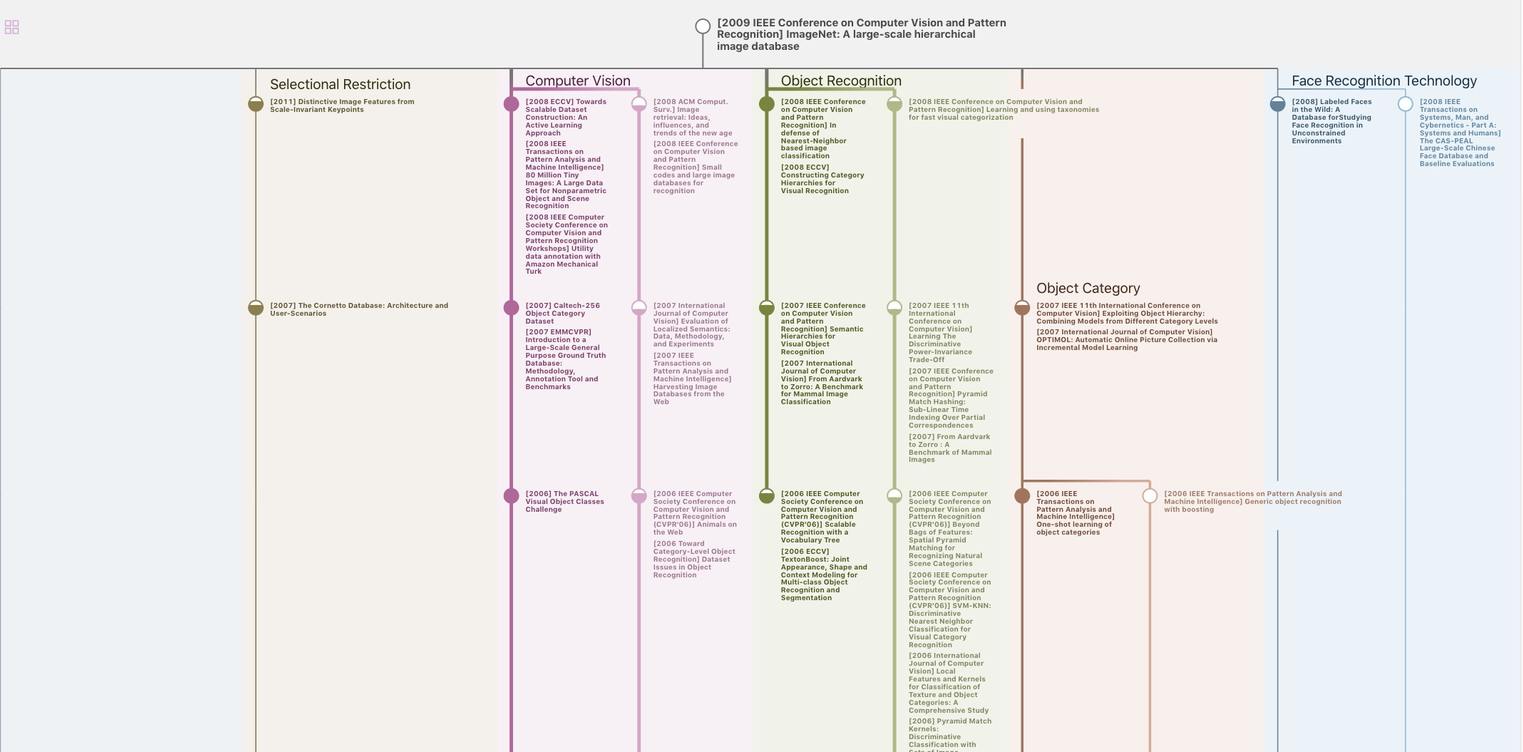
生成溯源树,研究论文发展脉络
Chat Paper
正在生成论文摘要