LLU-Swin: Low-Light Image Enhancement with U-shaped Swin Transformer.
Big Data(2022)
摘要
Low-light image enhancement is a low-level task that aims to improve the brightness and contrast of underexposed images. State-of-the-art low-light methods for image enhancement, benefiting from the development of deep learning, have made great progress. The majority of existing approaches, however, are based on convolutional neural networks, and just a few attempts have been made with Transformers which could perform admirably on high-level vision tasks by readily building long-range dependencies and global context connections. In this paper, we propose LLU-Swin, a hierarchical encoder-decoder architecture, based on Swin Transformer and U-Net for low-light image enhancement. LLU-Swin is composed of several Residual Recovery Transformer Modules (RRTM), each of which contains several improved Swin Transformer layers with a residual connection. We also show Dilated Local-enhanced Window Transformer Block (DLTB), which uses non-overlapping window-based self-attention to offer tremendous efficiency and employs Dilated Locally-enhanced Feed-Forward Network(D-LeFF) to enhance the local sensory ability. Benefits from these two designs, LLU-Swin has great power to capture both global and local contexts. Experimental results demonstrate the LLU-Swin outperforms the state-of-the-art methods in image quality and inference speed.
更多查看译文
关键词
enhancement,llu-swin,low-light,u-shaped
AI 理解论文
溯源树
样例
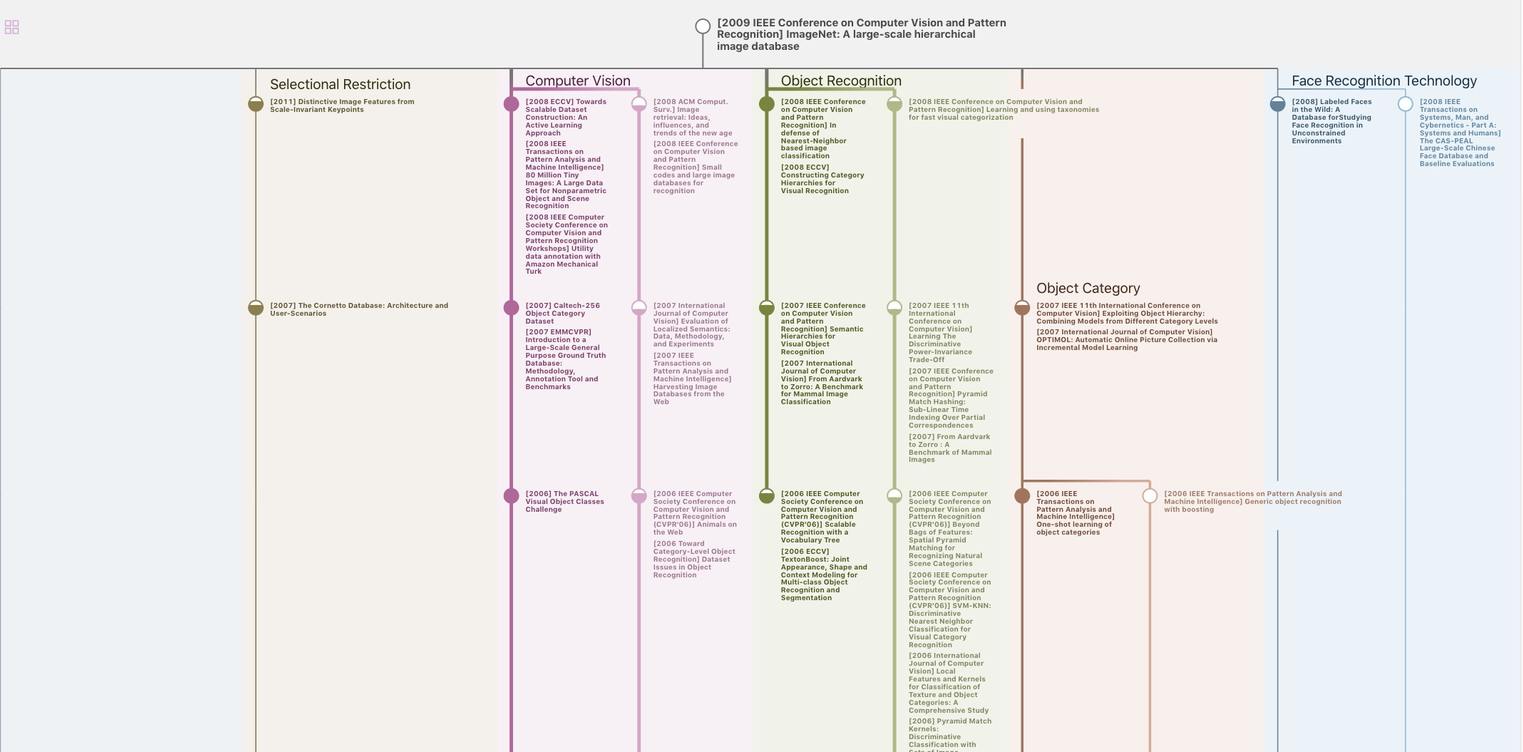
生成溯源树,研究论文发展脉络
Chat Paper
正在生成论文摘要