Performance Comparison for Deep Learning-Based Personal Identification from the database of ECG and EMG singals.
Big Data(2022)
摘要
This paper is concerned with performance comparison for personal identification from Electromyography (EMG) and Electrocardiogram (ECG) signals. For this purpose, we use Convolutional Neural Network (CNN) and Long Short Term Memory (LSTM) as the representative deep learning model for biometrics, respectively. In the case of CNN, the signals are transformed into two-dimensional time-frequency representation based on wavelet analysis. The transformed 2D images are used as input of CNN. Meanwhile, in the case of LSTM, the features are extracted such as the mean of absolute values, the amount of change in the mean of absolute values, the number of zero crossings, the number of gradient changes, and the wavelength. These five factors are used as input of LSTM.Finally, we perform the performance comparison from self-constructed ECG database and benchmark EMG database. The ECG dataset includes data from 100 people from 1 channel and the public EMG dataset includes data from 50 people from 8 channels. EMG signals are obtained while some motions are performing and ECG signals are obtained in a rest state. ECG-based biometrics is 10.95% higher accuracy than EMG-based biometrics. The experimental results revealed that the ECG signals showed more effective individual’s unique information in comparison with EMG signals through the performance comparison for personal identification.
更多查看译文
关键词
electromyogram,electrocardiogram,convolutional neural networks,long short term memory,personal identification
AI 理解论文
溯源树
样例
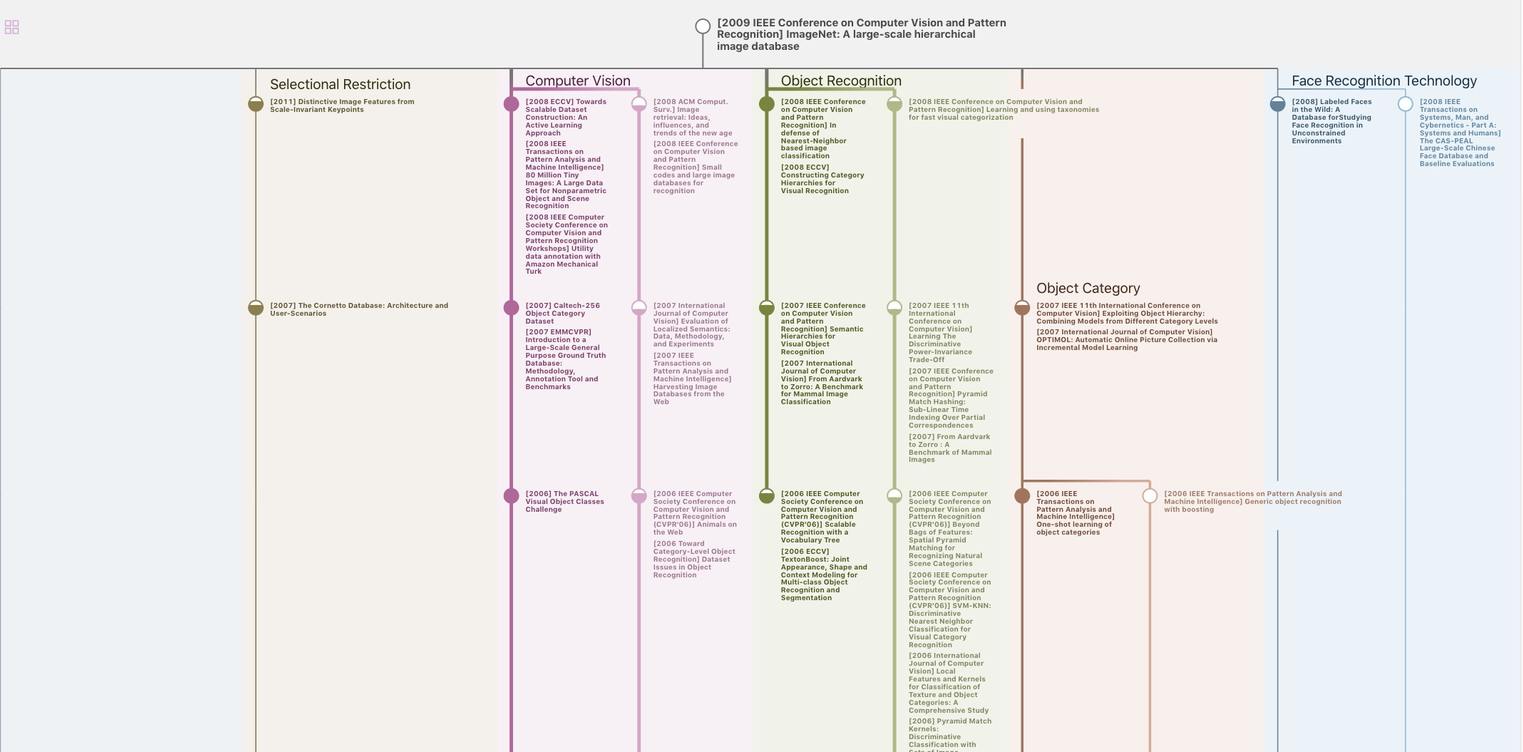
生成溯源树,研究论文发展脉络
Chat Paper
正在生成论文摘要