A Short Survey on the User Cold Start Problem in Recommender Systems: Metadata and Meta-Learning Methods.
Big Data(2022)
摘要
In recommender systems, the cold start problem, especially the user cold start one, is quite challenging yet important as it mostly comes with the rapid growth of the product and business. A well-performed new or early user recommender system has the potential to not only offer great onboarding experience, but also largely convert these users into loyal ones with considerable long-term values, which is strategically important from a product perspective. From a machine learning perspective, it is not an easy task as modern recommender systems heavily rely on large amounts of user-item interaction data to train a high quality model, and the data sparsity problem that new and early users bring is a clear challenge in terms of model training quality. In this short survey, among many proposed ideas on this topic, we focused on two general approaches for user cold start recommender system problems: metadata and meta-learning. Metadata methods generally aim at calculating similarities and learning representations by well utilizing limited user data that is collected regardless of users’ ratings for recommended items. Meta-learning methods learn the model in a more general and efficient way that could be adapted to new tasks with decent performance given data sparsity, which aligns the user cold start problem settings well.
更多查看译文
关键词
Recommender System,Cold Start,Metadata,Meta-learning
AI 理解论文
溯源树
样例
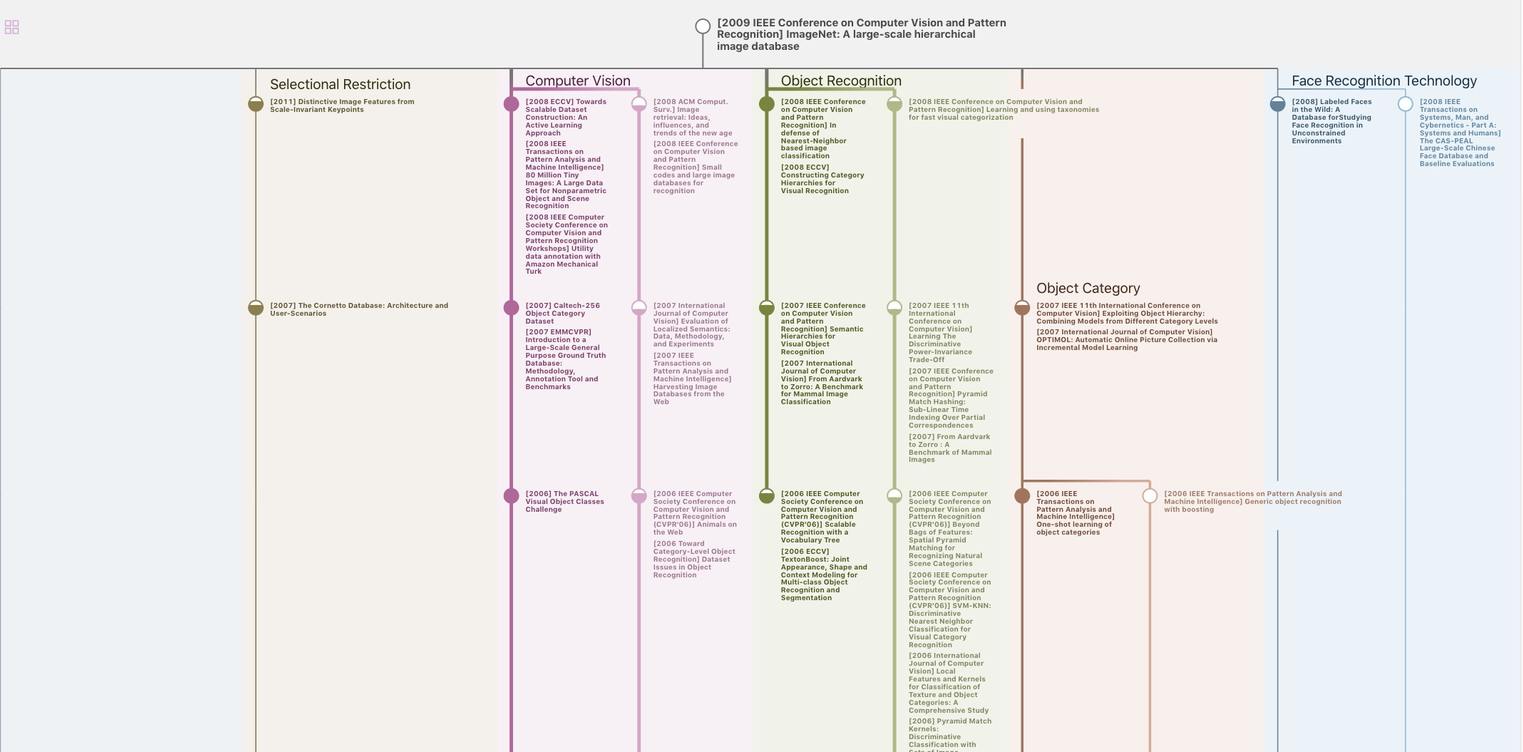
生成溯源树,研究论文发展脉络
Chat Paper
正在生成论文摘要