Feature Integration Strategies for Multilingual Fake News Classification
Big Data(2022)
摘要
The abundance of information in digital media, which in today’s world is the main source of knowledge about current events for the masses, makes it possible to spread disinformation on a larger scale than ever before. Consequently, there is a need to develop novel fake news detection approaches capable of adapting to changing factual contexts and generalizing previously or concurrently acquired knowledge. To deal with this problem, we propose a ensemble-based approach, which allows for fake news detection in multiple languages and the mutual transfer of knowledge acquired in each of them. Both classical feature extractors, such as Term frequency-inverse document frequency or Latent Dirichlet Allocation, and integrated deep NLP (Natural Language Processing) BERT (Bidirectional Encoder Representations from Transformers) models paired with MLP (Multilayer Perceptron) classifier, were employed. The results of experiments conducted on two datasets dedicated to the fake news classification t ask ( in English and Spanish, respectively), supported by statistical analysis, confirmed that utilization of additional languages could improve performance for traditional methods. Also, in some cases supplementing the deep learning method with classical ones can positively impact obtained results. The ability of models to generalize the knowledge acquired between the analyzed languages was also observed.
更多查看译文
关键词
natural language processing,classifier ensemble,transformers,bidirectional encoder,representation learning,BERT
AI 理解论文
溯源树
样例
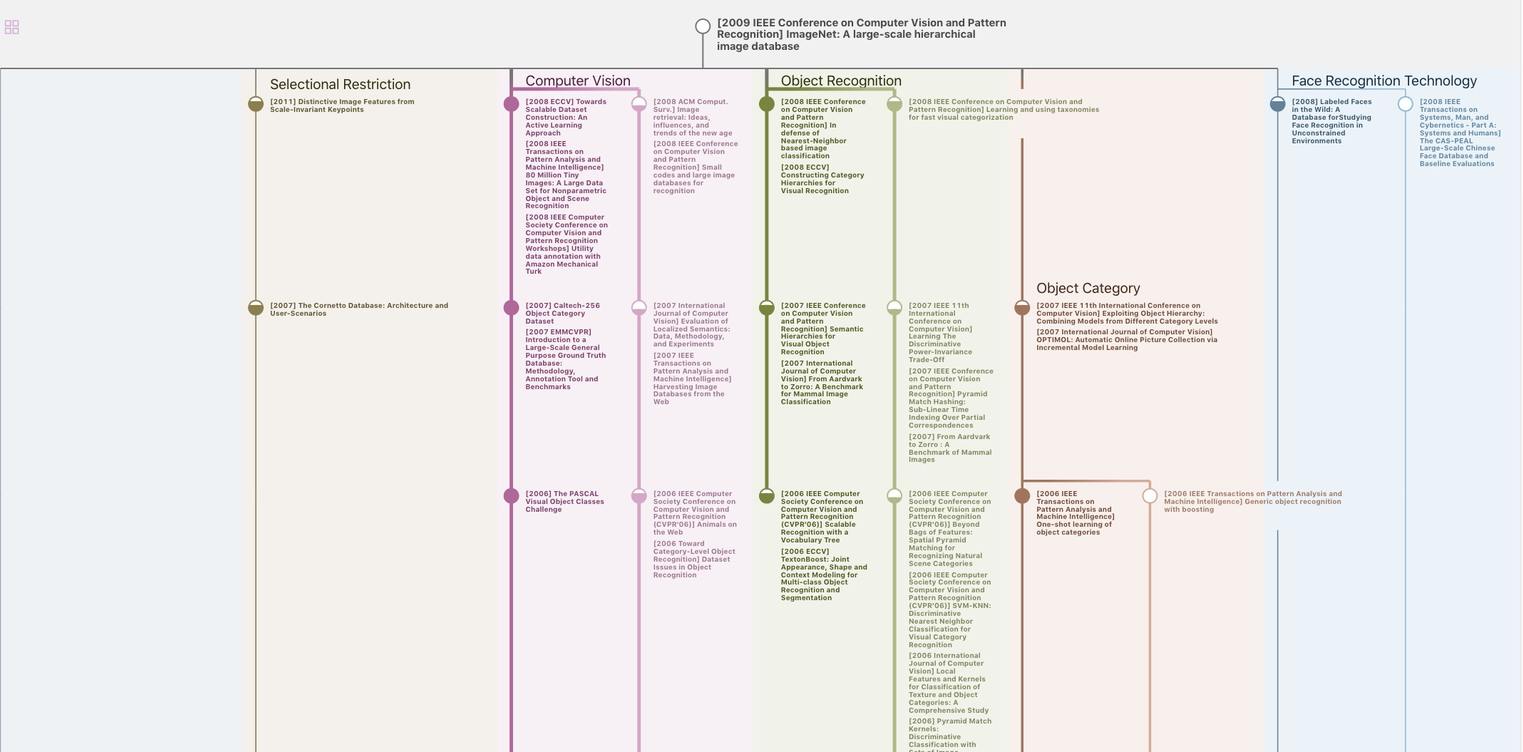
生成溯源树,研究论文发展脉络
Chat Paper
正在生成论文摘要