CLD-Net: Complement Local Detail For Medical Small-Object Segmentation.
BIBM(2022)
摘要
Deep learning has achieved outstanding performance in biomedical image segmentation. However, it still lacks attention to medical small-object segmentation scenarios, which is essential in early disease diagnosis. The low-resolution feature and single receptive field for medical small-object lead to a significant gap compared with the normal-scale object segmentation. This paper proposes a novel complement local detail-based network architecture (CLD-Net) for medical small-object segmentation, which can complement local detailed information when up-sampling global features. In details, the CLD-Net architecture is established with two sub-modules, i.e., Local Edge Feature Extraction Block (LEFE) and Local-Global Feature Fusion Block (LGFF). LEFE aims to maintain a high-resolution edge-feature sequence corresponding to each layer of the encoder through a progressive scheme. LGFF further rectifies the difference of features to represent the layer-aware detailed information, while the segmentation map can be obtained by incorporating the layer-aware local detailed features into the low-resolution fine-grained global feature. The experimental results on the polyp segmentation task demonstrate the effectiveness of the proposed method. CLD-Net outperforms state-of-the-art methods for small-object segmentation.
更多查看译文
关键词
complement local detail,segmentation,cld-net,small-object
AI 理解论文
溯源树
样例
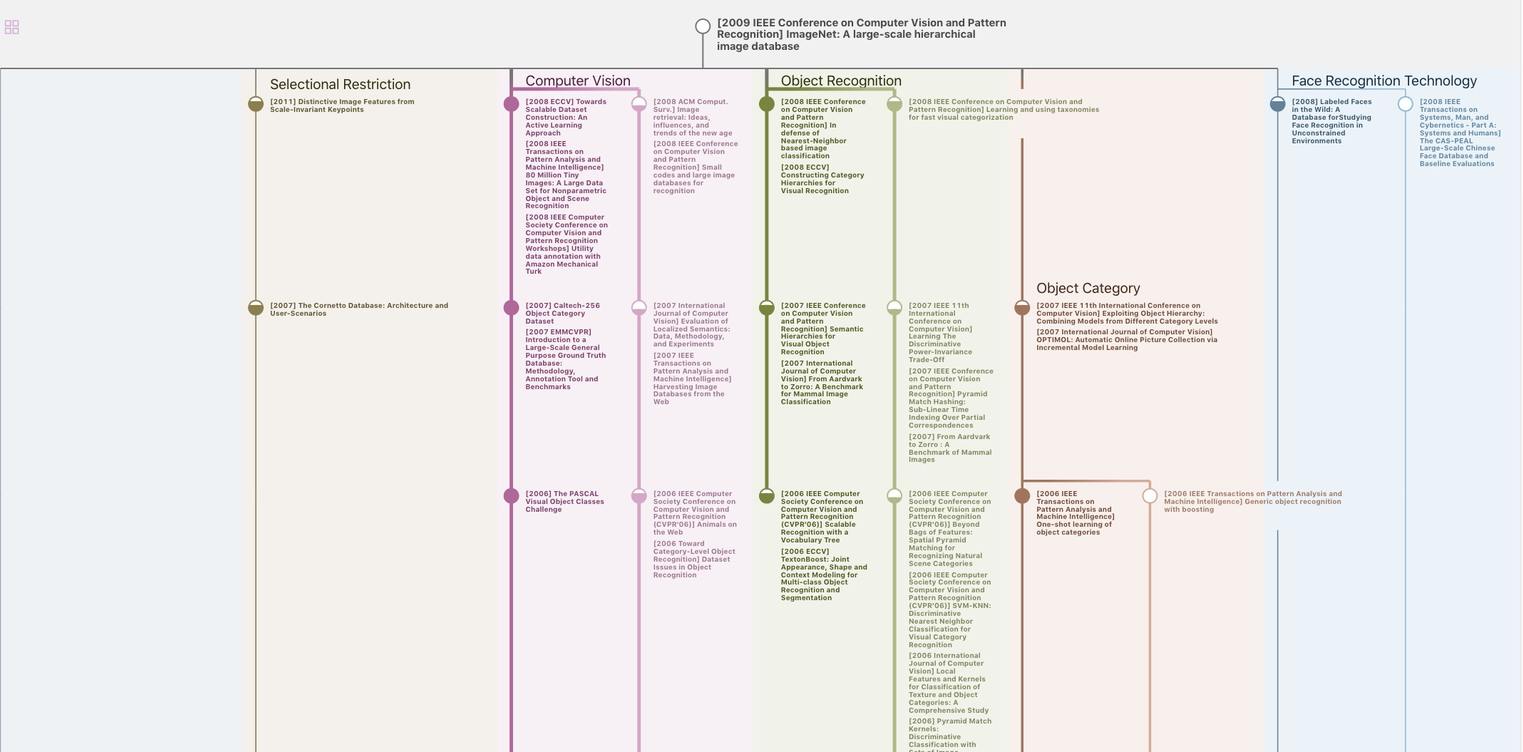
生成溯源树,研究论文发展脉络
Chat Paper
正在生成论文摘要