Uncertainty-guided Mutual Consistency Training for Semi-supervised Biomedical Relation Extraction.
BIBM(2022)
摘要
Biomedical relation extraction seeks to automatically extract biomedical relations from biomedical text, which plays an important role in biomedical studies. However, constructing high-quality biomedical annotation data is not only time-consuming but also requires a high level of knowledge in the biomedical field. To alleviate this problem, Semi-supervised Biomedical Relation Extraction aims to extract relation facts from the limited labeled data and the more readily available unlabeled samples. Existing works can be roughly categorized as self-training methods and self-ensembling methods. The former aims to generate pseudo labels, which may lead to the gradual drift problem. The latter aims to encourage the output of one model to be consistent with the other model, where the acquisition of the model is tedious. To alleviate these issues, we propose a novel Uncertainty-Guided Mutual Consistency Training framework(UG-MCT) for semi-supervised Biomedical relation extraction. Specifically, our framework consists of two models with the same structure, which differ only when updating their weights, and then an intersecting pseudo-label mechanism is designed to convert the prediction discrepancies of the two models into mutual consistency training loss, thus promoting the consistency of model predictions. In addition, we utilize uncertainty as guided information to assist the model in focusing on the confident pseudo labels and mitigate the noise of inaccurate pseudo labeling during training. Thus, our model is very simple and efficient while mitigating the noise introduced by pseudo-labels. UG-MCT is evaluated on multiple datasets in different settings and the experimental results demonstrate that our method is highly effective in semi-supervised biomedical relation extraction compared to the state-of-the-art.
更多查看译文
关键词
mutual consistency training,relation,extraction,uncertainty-guided,semi-supervised
AI 理解论文
溯源树
样例
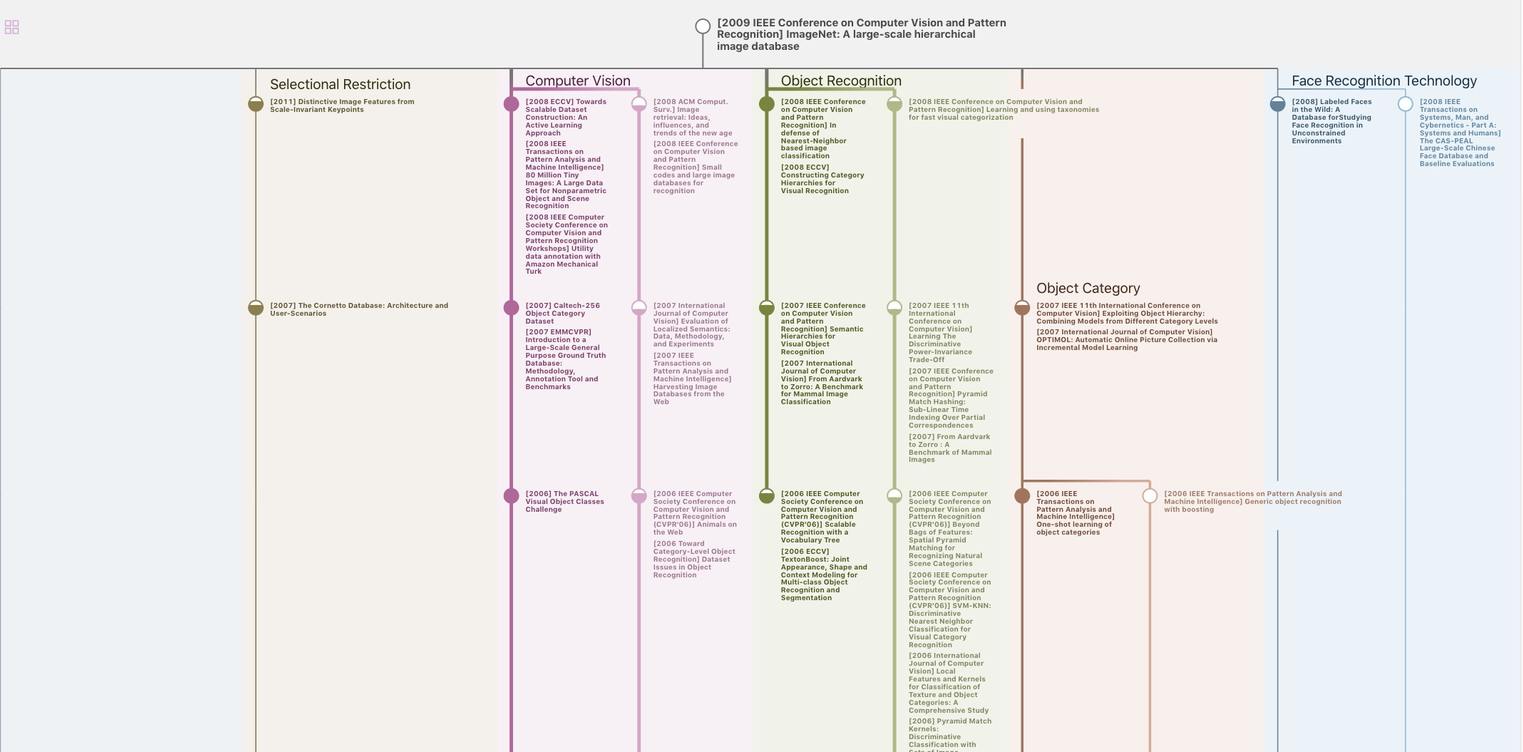
生成溯源树,研究论文发展脉络
Chat Paper
正在生成论文摘要