Joint Feature Learning for Cell Segmentation Based on Multi-scale Convolutional U-Net
BIBM(2022)
摘要
A major challenge in the analysis of tissue imaging data is cell segmentation, the task of identifying precisely the boundary of each cell in a microscopic image. The cell segmentation task is still challenging due to the variable shapes, large size differences, uneven grayscale, and dense distribution of biological cells in microscopic images. In this paper, we propose a joint feature learning method that integrates the density and boundary branch into a multi-scale convolutional U-Net (MC-Unet). To enhance the supervision of cell density and boundary detection, the density and boundary loss is constructed to guide the joint learning of multiple features, where the density loss branch can address the challenges posed by high density, while the boundary loss branch can address the problems of unclear cell boundaries and partial cell occlusion. A series of experiments on different cell datasets show that two auxiliary branches improve the learning of features on cell density and cell boundaries and that the proposed method is effective on different segmentation models. The code is available at: https://github.com/HuHaigen/Joint-Feature-Learning-for-Cell-Segmentation.
更多查看译文
关键词
Cell Segmentation,Joint Feature Learning,Convolutional Neural Network (CNN),MC-Unet,Multi-task Learning
AI 理解论文
溯源树
样例
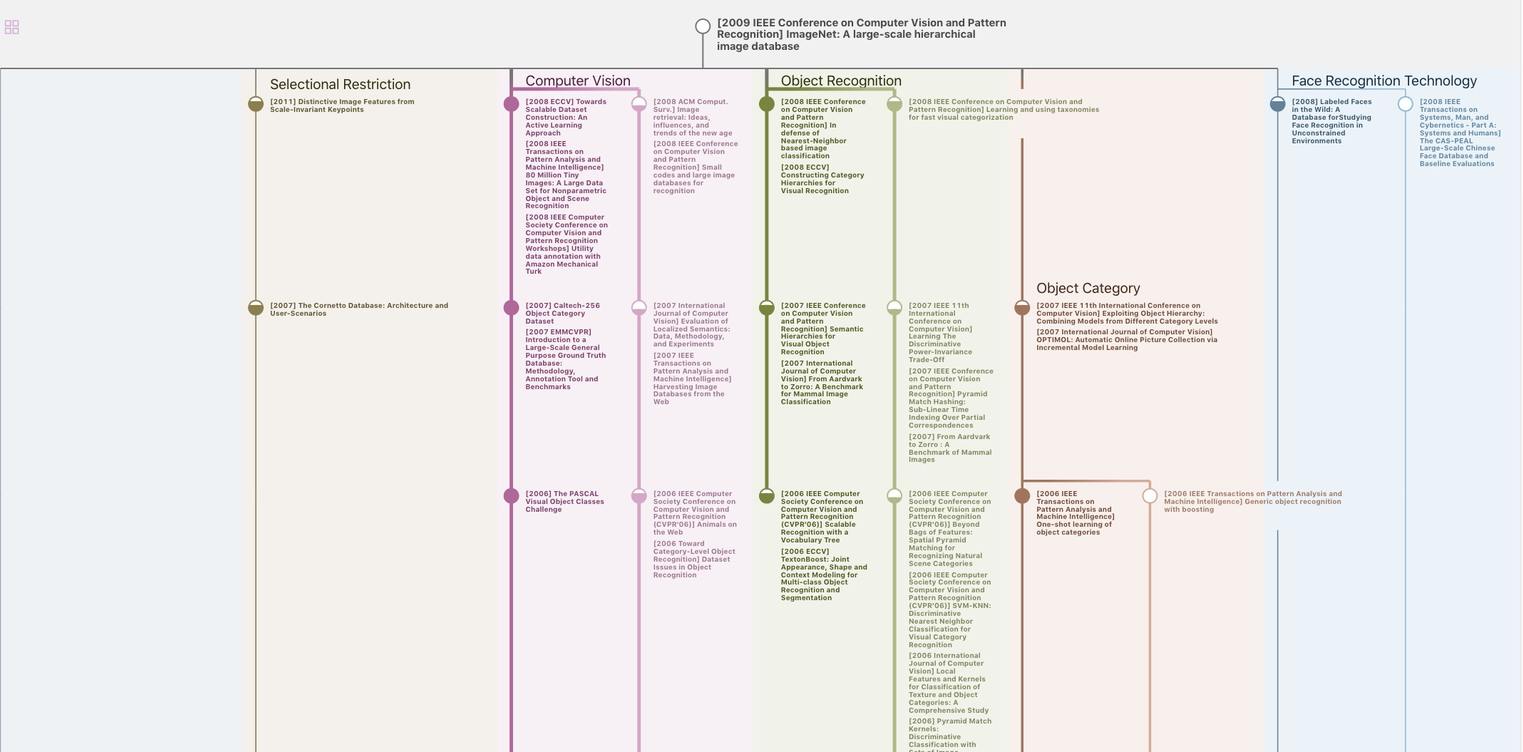
生成溯源树,研究论文发展脉络
Chat Paper
正在生成论文摘要